DATUM: Dotted Attention Temporal Upscaling Method
semanticscholar(2020)
摘要
Computational simulations frequently only save a subset of their time slices, e.g., running for one thousand cycles, but saving only fifty time slices. With this work we consider the problem of temporal upscaling, i.e. inferring visualizations at time slices that were not saved, as applied to ensemble simulations. We contribute a new algorithm, which we call DATUM, which incorporates machine learning techniques, specifically, dotted attention and convolutional networks. To evaluate our approach, we conduct 1327 experiments, on 32x32 pixel renderings of two-dimensional data sets. Our experiments infer imagery at unsaved time slices and compared to ground truth renderings both visually and with an established metric (peak signal-to-noise, or PSNR). We also compare to a linear interpolation method, and find that our technique has a significantly higher accuracy, in some cases producing renderings that are 19% more accurate. Overall, we demonstrate that our method can learn patterns from a single simulation within an ensemble and use this information to perform temporal upscaling on other simulations within the same ensemble that are sparsely saved. We show that with 1% of data from a new simulation, equivalent to a simulation saving imagery one out of every hundred cycles, is enough to improve accuracy for temporal upscaling.
更多查看译文
AI 理解论文
溯源树
样例
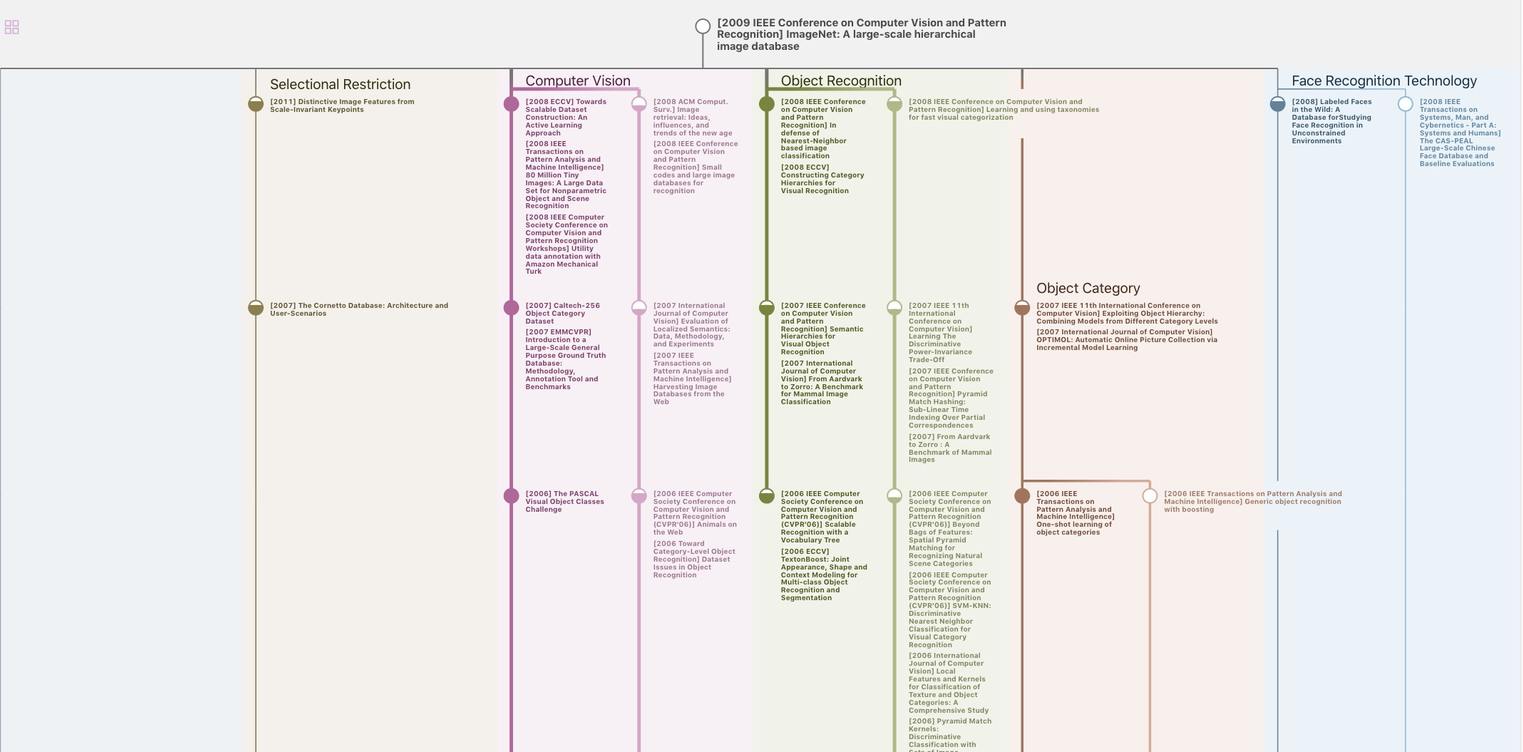
生成溯源树,研究论文发展脉络
Chat Paper
正在生成论文摘要