Gaussian Density Parametrization Flow: Particle and Stochastic Approaches
semanticscholar(2021)
摘要
Bayesian inference is intractable for most practical problems and requires approximation schemes with several trade-offs. Variational inference provides one of such approximations which, while powerful, has thus far seen limited use in high-dimensional applications due to its complexity and computational cost. This paper introduces a scalable, theoreticallygrounded, and simple-to-implement algorithm for approximate inference with a variational Gaussian distribution. Specifically, we establish a practical particle-based algorithm to perform variational Gaussian inference that scales linearly in the problem dimensionality. We show that our approach performs on par with the state of the art on a set of challenging high-dimensional problems.
更多查看译文
AI 理解论文
溯源树
样例
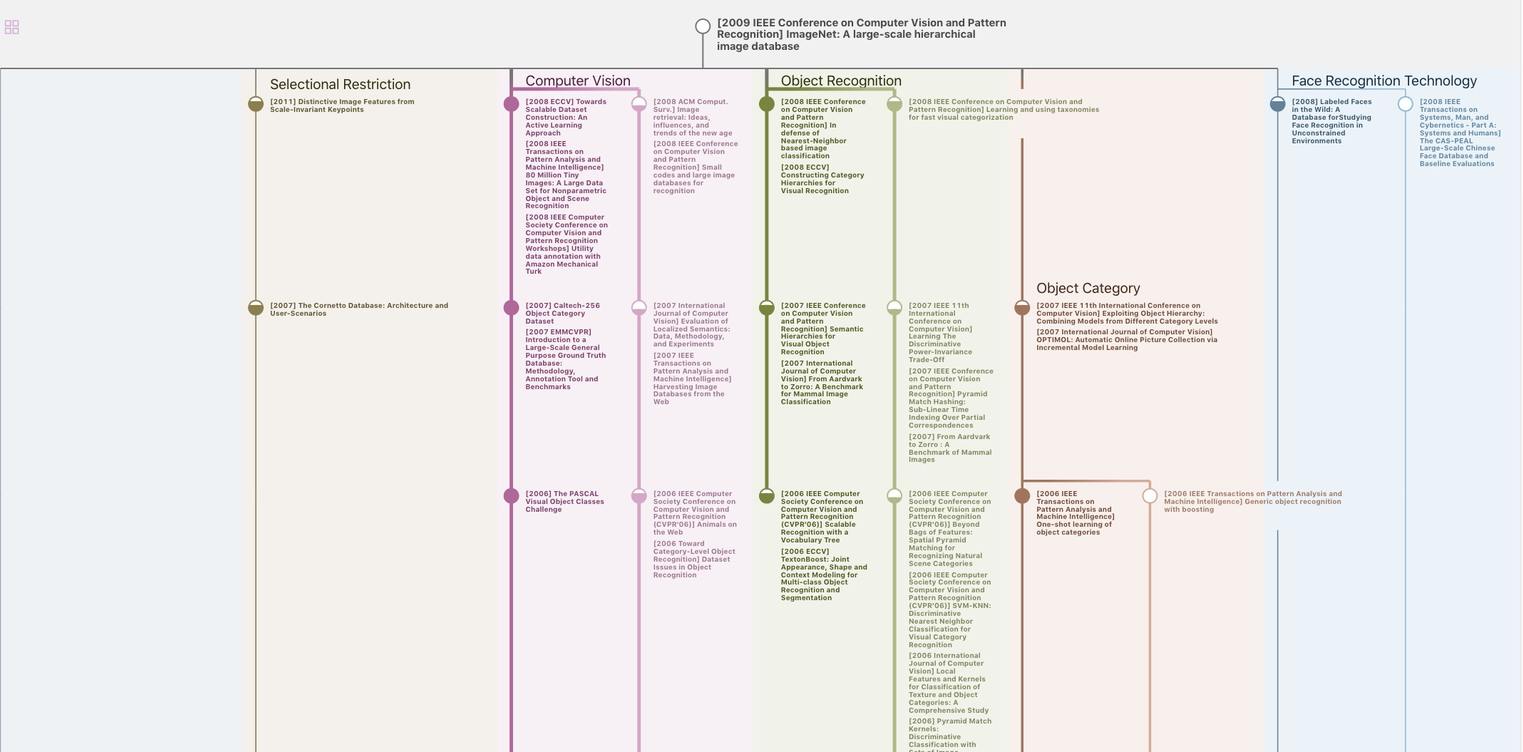
生成溯源树,研究论文发展脉络
Chat Paper
正在生成论文摘要