Stratified Sampling Based Compressed Sensing for Structured Signals
IEEE TRANSACTIONS ON SIGNAL PROCESSING(2022)
摘要
Structured compressed sensing takes signal structure into account, thereby outperforming earlier compressed sensing methods. However, results are usually based on sampling in the Fourier domain, such as in Magnetic Resonance Imaging. In the time domain, the benefits of structured compressed sensing are still unknown. This paper introduces concepts that incorporate the signal structure into both the acquisition and reconstruction of compressed sensing in time and image domain applications. First, a stratified-random sampling pattern is proposed to improve the recovery of the dominant low-frequency range of natural signals. A heuristic decay of primes criterion is developed to evaluate the properties of the sensing matrix and is used to optimize the sampling pattern. Second, the sparsity of the Fourier transform as the representation domain is improved by estimating the signal structure in a preprocessing step, and then adapting the grid of the Fourier transform. In contrast to existing methods, grid stretching is integrated into the fast Fourier transform to reduce computational complexity. Both structured acquisition and reconstruction are evaluated using simulations, as well as two real-world applications: wireless sensor networks in structural health monitoring and electron microscopy. Results show that both reconstruction errors and robustness can significantly be improved by incorporating structure into the acquisition and reconstruction.
更多查看译文
关键词
Image reconstruction,Fourier transforms,Sensors,Time measurement,Standards,Compressed sensing,Time-domain analysis,Electron microscopy,structured compressed sensing,stratified random sampling,structural health monitoring
AI 理解论文
溯源树
样例
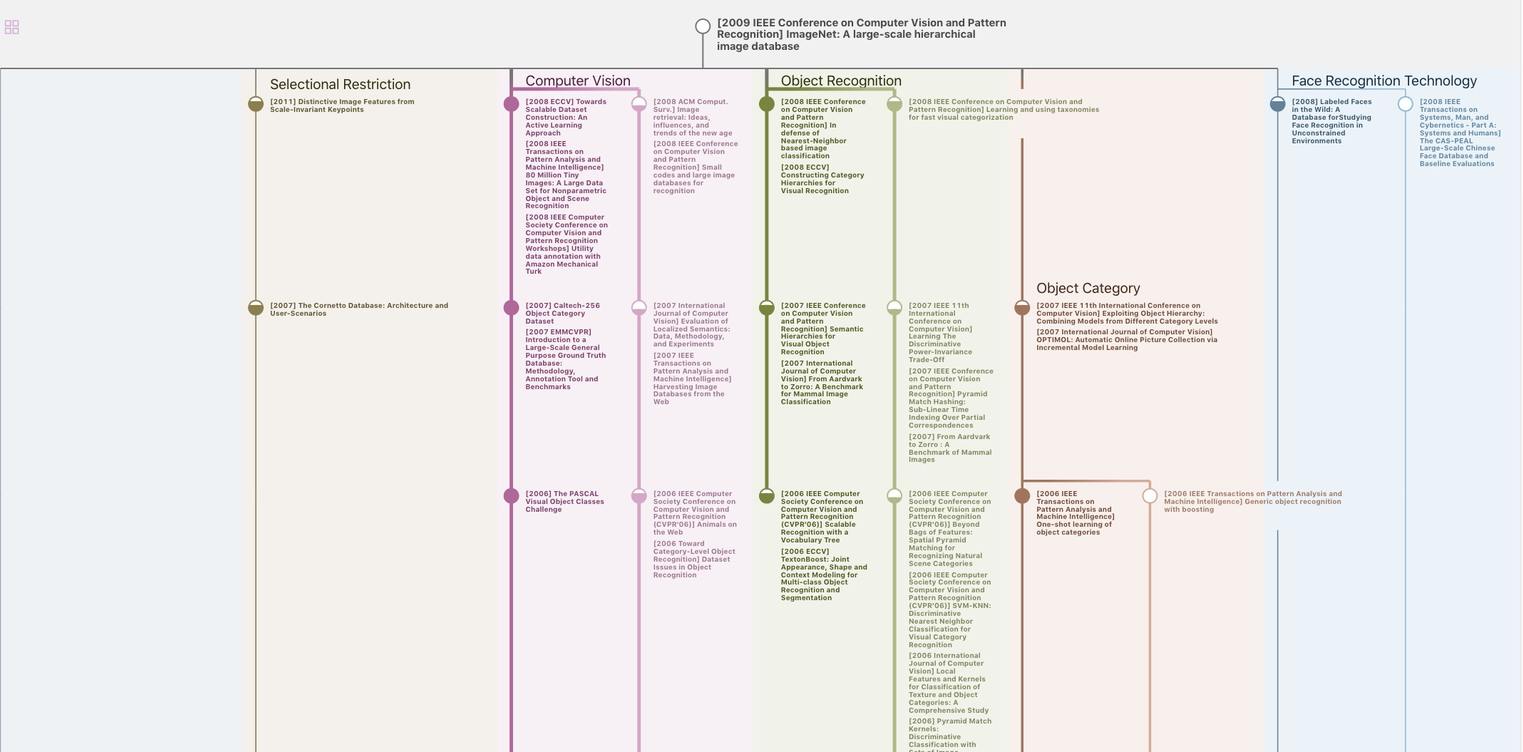
生成溯源树,研究论文发展脉络
Chat Paper
正在生成论文摘要