Dataset Security for Machine Learning: Data Poisoning, Backdoor Attacks, and Defenses
IEEE Transactions on Pattern Analysis and Machine Intelligence(2022)
摘要
As machine learning systems grow in scale, so do their training data requirements, forcing practitioners to automate and outsource the curation of training data in order to achieve stateof-the-art performance. The absence of trustworthy human supervision over the data collection process exposes organizations to security vulnerabilities; training data can be manipulated to control and degrade the downstream behaviors of learned models. The goal of this work is to systematically categorize and discuss a wide range of dataset vulnerabilities and exploits, approaches for defending against these threats, and an array of open problems in this space. In addition to describing various poisoning and backdoor threat models and the relationships among them, we develop their unified taxonomy.
更多查看译文
关键词
dataset security,backdoor attacks
AI 理解论文
溯源树
样例
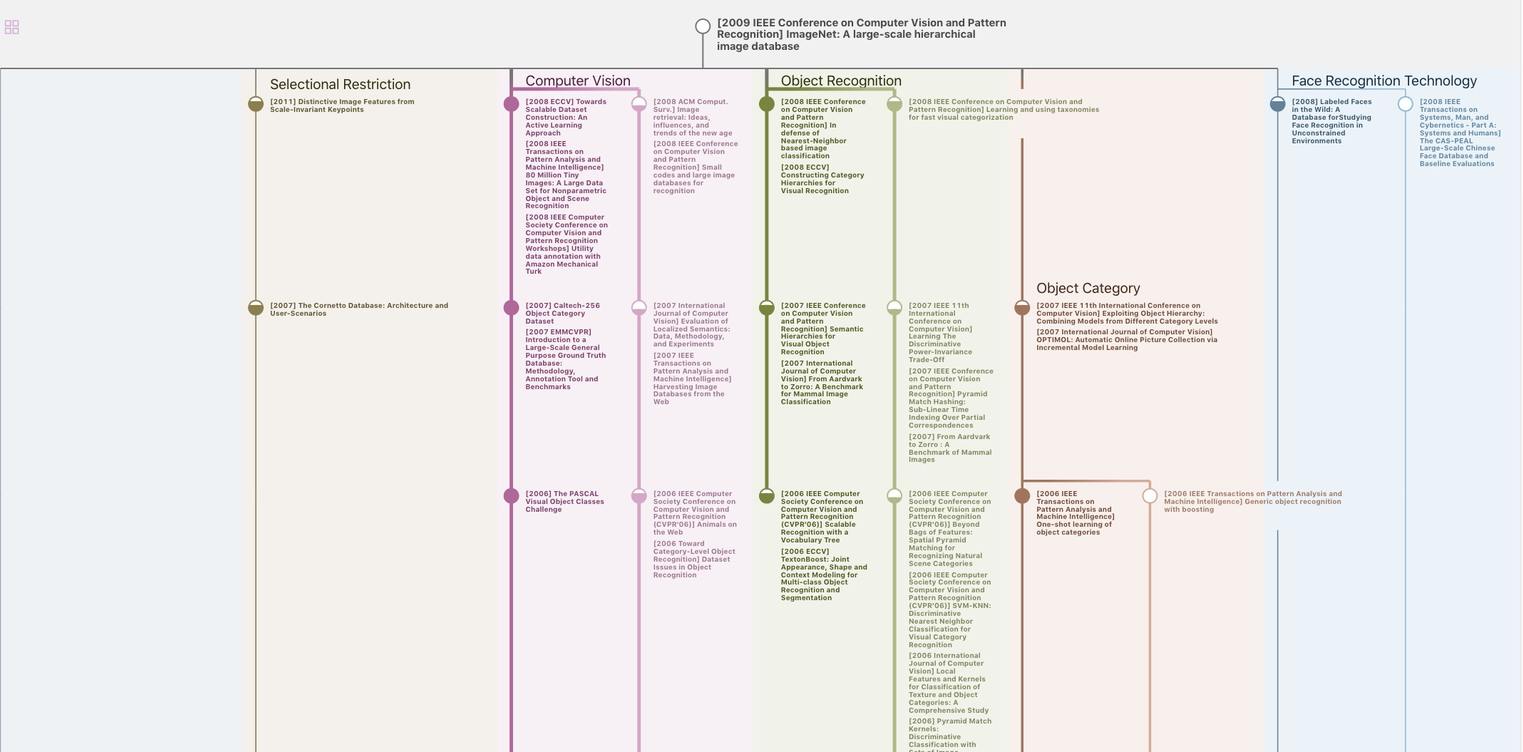
生成溯源树,研究论文发展脉络
Chat Paper
正在生成论文摘要