Hotel2vec: Learning Hotel Embeddings from User Click Sessions with Side Information.
RecTour@RecSys(2021)
摘要
We propose a new neural network architecture for learning vector representations of items with attributes, specifically hotels. Unlike previous works, which typically only rely on modeling of user-item interactions for learning item embeddings, we propose a framework that combines several sources of data, including user clicks, hotel attributes (e.g., property type, star rating, average user rating), amenity information (e.g., if the hotel has free Wi-Fi or free breakfast), and geographic information that leverages an hexagonal geospatial system as well as spatial encoders. During model training, a joint embedding is learned from all of the above information. We show that including structured attributes about hotels enables us to make better predictions in a downstream task than when we rely exclusively on click data. We train our embedding model on more than 60 million user click sessions from a leading online travel platform, and learn embeddings for more than one million hotels. Our final learned embeddings integrate distinct sub-embeddings for user clicks, hotel attributes, and geographic information, providing a representation that can be used flexibly depending on the application. An important advantage of the proposed neural model is that it addresses the cold-start problem for hotels with insufficient historical click information by incorporating additional hotel attributes, which are available for all hotels. We show through the results of an online A/B test that our model generates high-quality representations that boost the performance of a hotel recommendation system on a large online travel platform.
更多查看译文
AI 理解论文
溯源树
样例
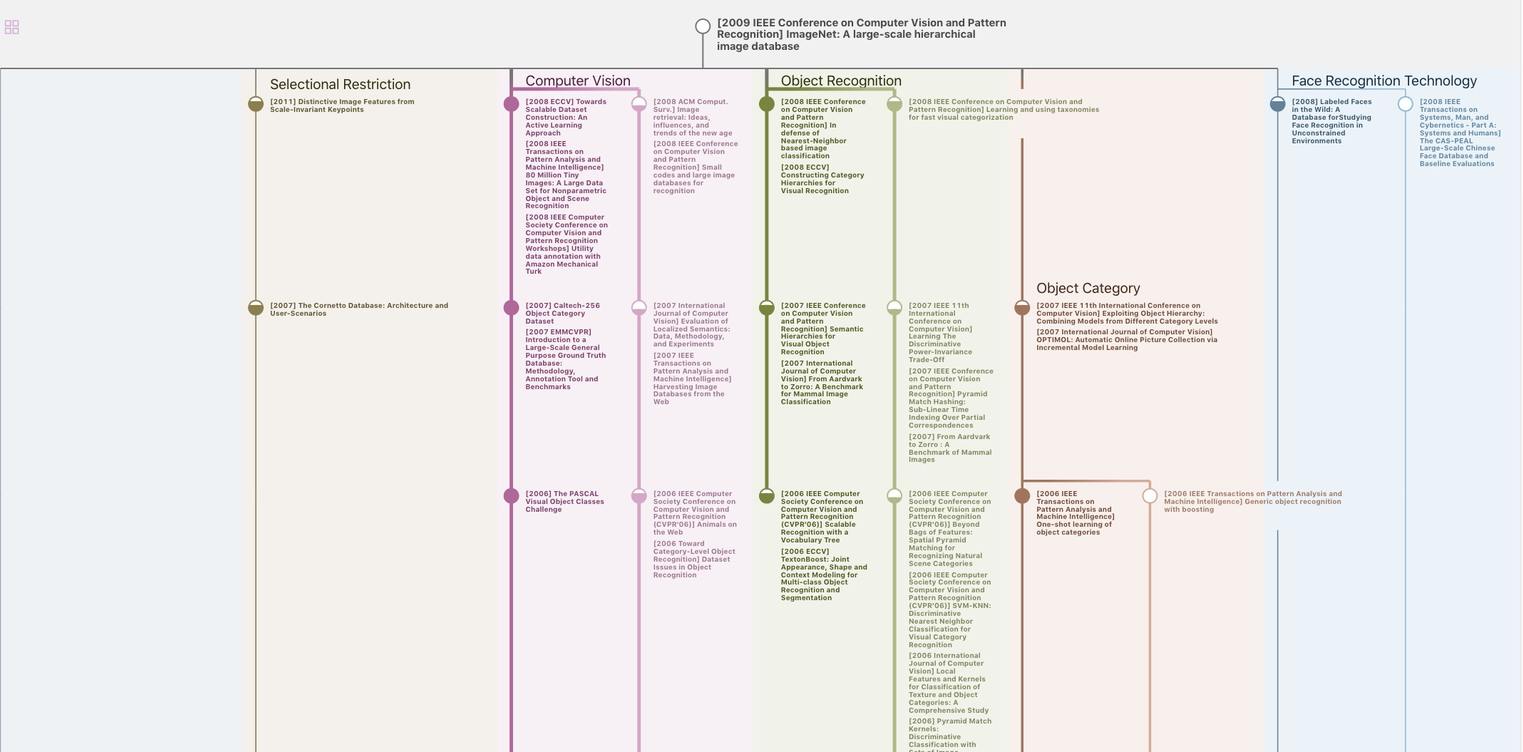
生成溯源树,研究论文发展脉络
Chat Paper
正在生成论文摘要