Mixed memory Markov models
semanticscholar(2021)
摘要
We consider how to parameterize Markov models with prohibitively large state spaces. This is done by representing the transition matrix as a convex combination-or mixtureof simpler dynamical models. The parameters in these models admit a simple probabilistic interpretation and can be fitted iteratively by an Expectation-Maximization (EM) procedure. We give examples where these models may be a faithful and/or useful representation of the underlying dynamics. We also derive a set of generalized Baum-Welch updates for hidden Markov models (HMMs) that make use of this parameterization. Because these models decompose the hidden state as the Cartesian product of two or more random variables, they are well suited to the modeling of coupled time series.
更多查看译文
AI 理解论文
溯源树
样例
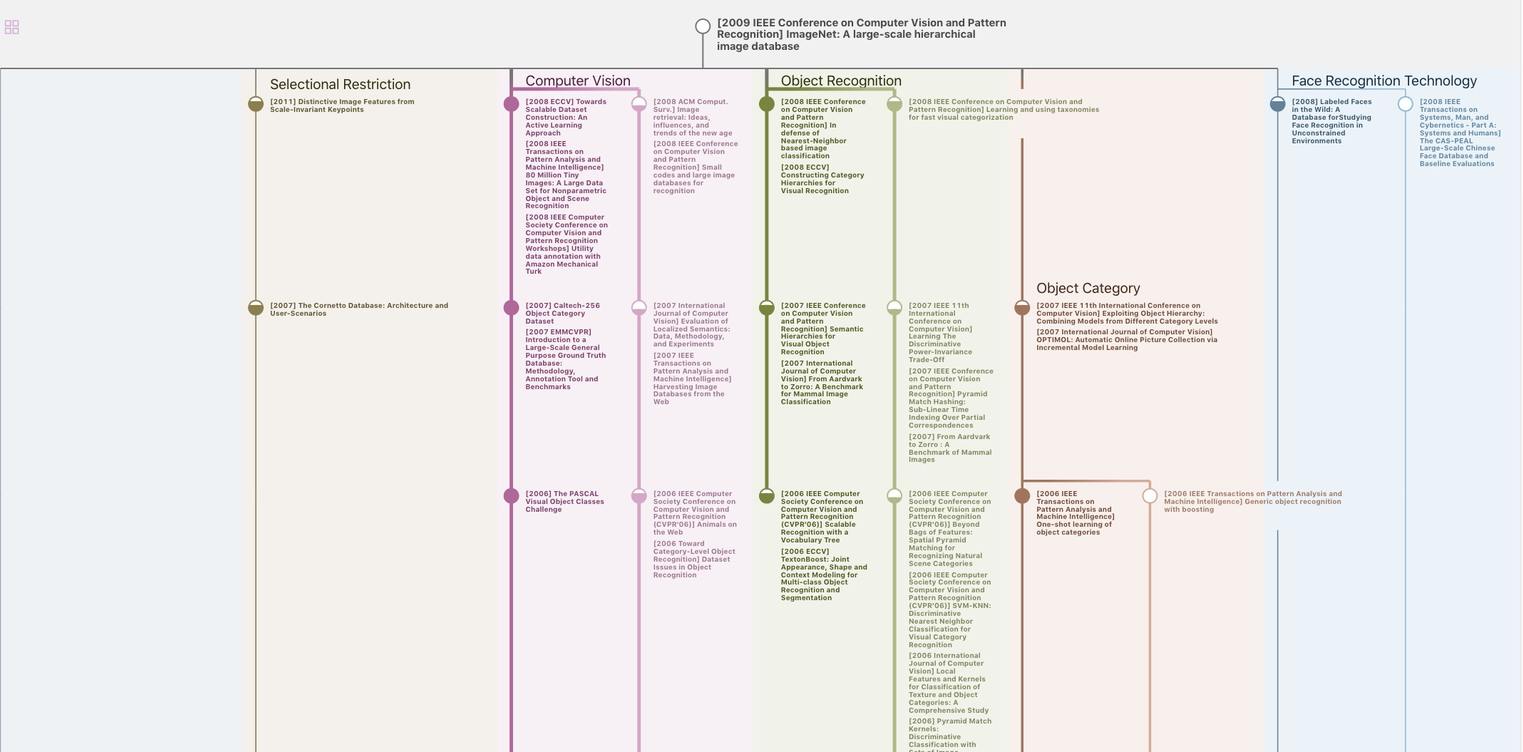
生成溯源树,研究论文发展脉络
Chat Paper
正在生成论文摘要