BotSpot++: A Hierarchical Deep Ensemble Model for Bots Install Fraud Detection in Mobile Advertising
ACM TRANSACTIONS ON INFORMATION SYSTEMS(2022)
摘要
AbstractMobile advertising has undoubtedly become one of the fastest-growing industries in the world. The influx of capital attracts increasing fraudsters to defraud money from advertisers. Fraudsters can leverage many techniques, where bots install fraud is the most difficult to detect due to its ability to emulate normal users by implementing sophisticated behavioral patterns to evade from detection rules defined by human experts. Therefore, we proposed BotSpot1 for bots install fraud detection previously. However, there are some drawbacks in BotSpot, such as the sparsity of the devices’ neighbors, weak interactive information of leaf nodes, and noisy labels. In this work, we propose BotSpot++ to improve these drawbacks: (1) for the sparsity of the devices’ neighbors, we propose to construct a super device node to enrich the graph structure and information flow utilizing domain knowledge and a clustering algorithm; (2) for the weak interactive information, we propose to incorporate a self-attention mechanism to enhance the interaction of various leaf nodes; and (3) for the noisy labels, we apply a label smoothing mechanism to alleviate it. Comprehensive experimental results show that BotSpot++ yields the best performance compared with six state-of-the-art baselines. Furthermore, we deploy our model to the advertising platform of Mobvista,2 a leading global mobile advertising company. The online experiments also demonstrate the effectiveness of our proposed method.
更多查看译文
关键词
Mobile ad fraud, app install fraud, bots fraud detection, graph neural network
AI 理解论文
溯源树
样例
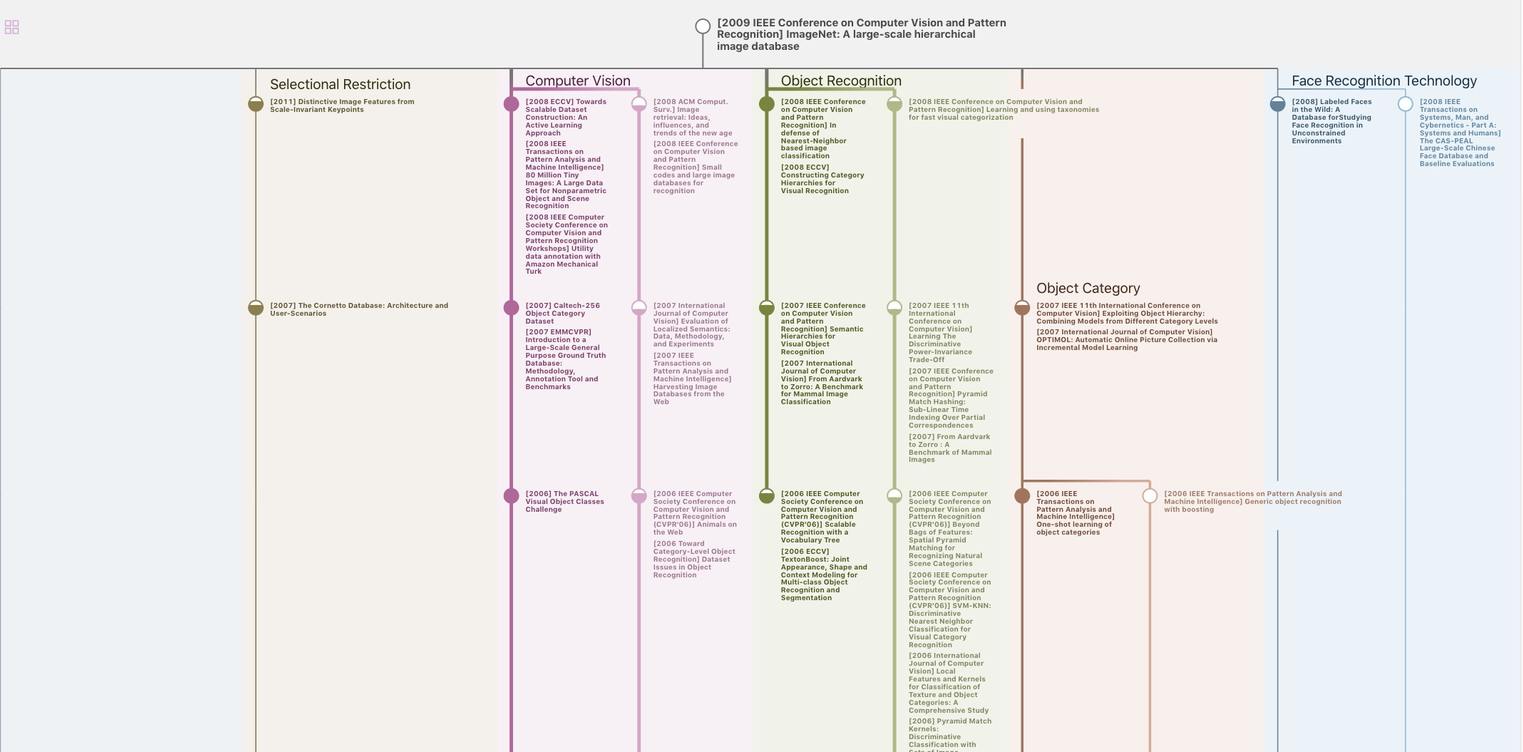
生成溯源树,研究论文发展脉络
Chat Paper
正在生成论文摘要