Orbis: Explainable Benchmarking of Information Extraction Tasks
semanticscholar(2021)
摘要
Competitive benchmarking of information extraction methods has considerably advanced the state of the art in this field. Nevertheless, methodological support for explainable benchmarking, which provides researchers with feedback on the strengths and weaknesses of their methods and guidance for their development efforts, is very limited. Although aggregated metrics such as F1 and accuracy support comparison of annotators, they do not help in explaining annotator performance. This work addresses the need for explainability by presenting Orbis, a powerful and extensible explainable evaluation framework which supports drill-down analysis, multiple annotation tasks and resource versioning. It, therefore, actively aids developers in better understanding evaluation results and identifying shortcomings in their systems. Orbis currently supports four information extraction tasks: content extraction, named entity recognition, named entity linking and slot filling. This article introduces a unified formal framework for evaluating these tasks, presents Orbis’ architecture, and illustrates how it (i) creates simple, concise visualizations that enable visual benchmarking, (ii) supports different visual classification schemas for evaluation results, (iii) aids error analysis, and (iv) enhances interpretability, reproducibility and explainability of evaluations by adhering to the FAIR principles, and using lenses which make implicit factors impacting evaluation results such as tasks, entity classes, annotation rules and the target knowledge graph more explicit.
更多查看译文
AI 理解论文
溯源树
样例
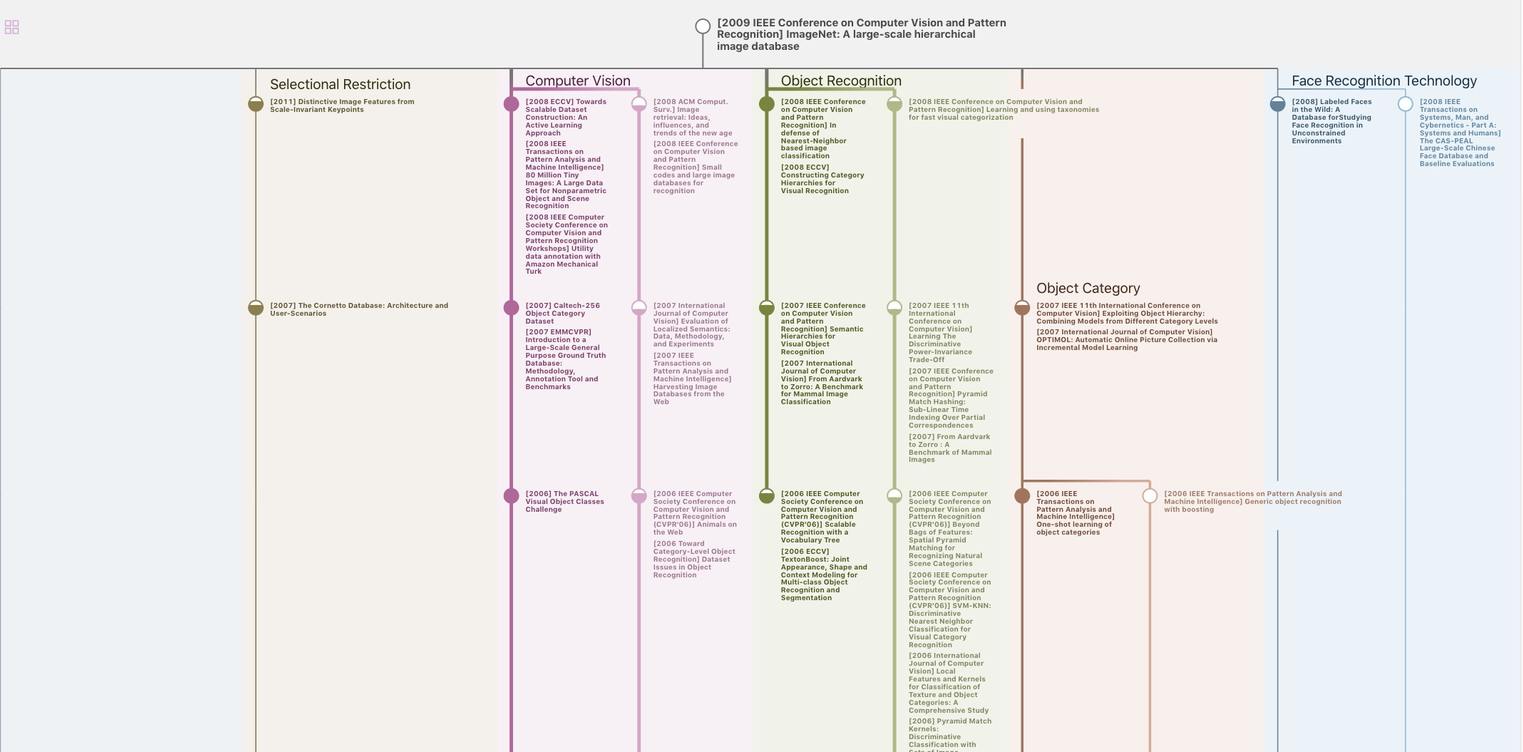
生成溯源树,研究论文发展脉络
Chat Paper
正在生成论文摘要