Can Your AI Differentiate Cats from Covid-19?Sample Efficient Uncertainty Estimation for Deep Learning Safety
semanticscholar(2020)
摘要
Deep Neural Networks (DNNs) are known to make highly overconfident predictions on Outof-Distribution data. Recent research has shown that uncertainty-aware models, such as, Bayesian Neural Network (BNNs) and Deep Ensembles, are less susceptible to this issue. However research in this area has been largely confined to the big data setting. In this work, we show that even state-of-the-art BNNs and Ensemble models tend to make overconfident predictions when the amount of training data is insufficient. This is especially concerning for emerging applications in physical sciences and healthcare where overconfident and inaccurate predictions can lead to disastrous consequences. To address the issue of accurate uncertainty (or confidence) estimation in the small-data regime, we propose a probabilistic generalization of the popular sample-efficient non-parametric kNN approach. We demonstrate the usefulness of the proposed approach on a realworld application of COVID-19 diagnosis from chest X-Rays by (a) highlighting surprising failures of existing techniques, and (b) achieving superior uncertainty quantification as compared to state-of-the-art.
更多查看译文
AI 理解论文
溯源树
样例
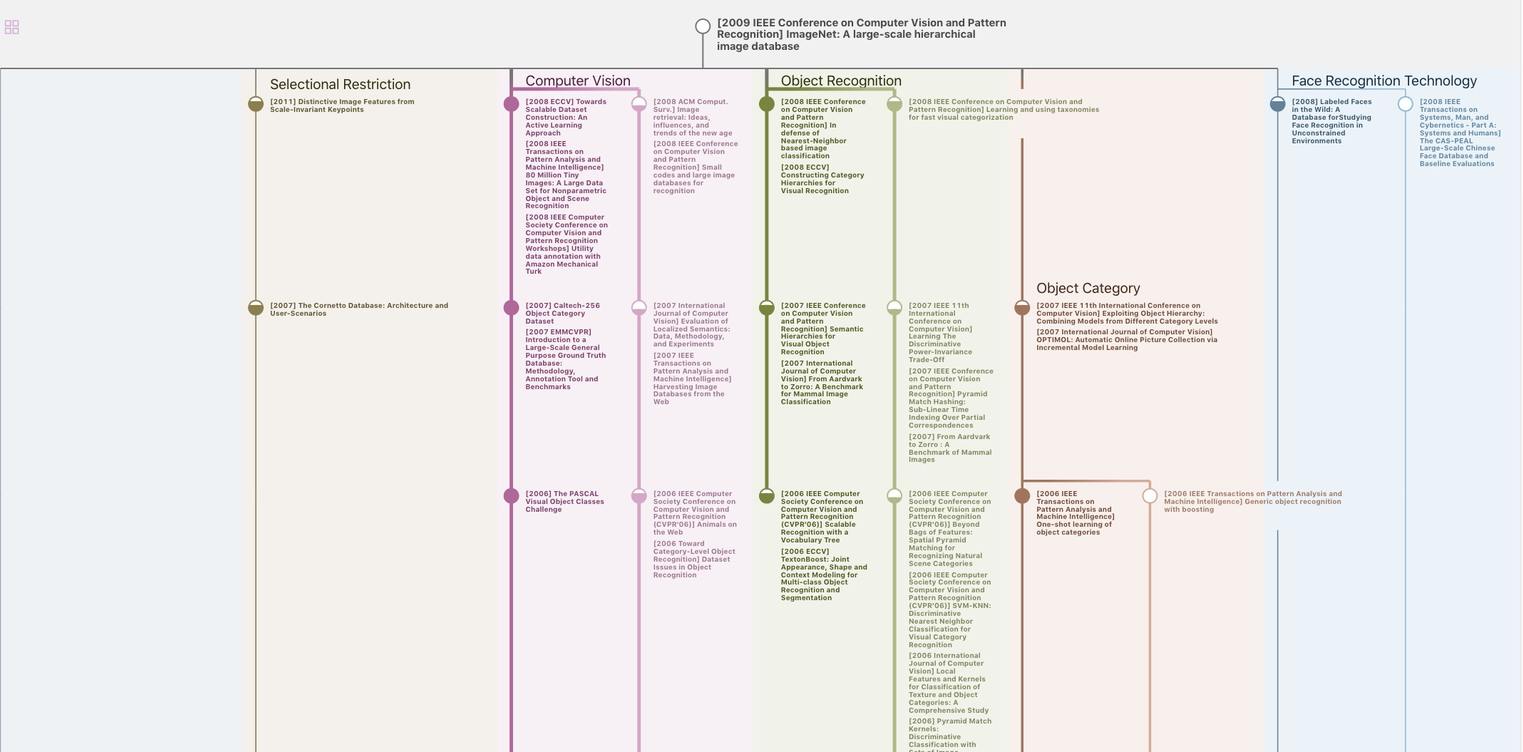
生成溯源树,研究论文发展脉络
Chat Paper
正在生成论文摘要