Knowledge Distillation with Attention for Deep Transfer Learning of Convolutional Networks
ACM TRANSACTIONS ON KNOWLEDGE DISCOVERY FROM DATA(2022)
摘要
Transfer learning through fine-tuning a pre-trained neural network with an extremely large dataset, such as ImageNet, can significantly improve and accelerate training while the accuracy is frequently bottlenecked by the limited dataset size of the new target task. To solve the problem, some regularization methods, constraining the outer layer weights of the target network using the starting point as references (SPAR), have been studied. In this article, we propose a novel regularized transfer learning framework DELTA, namely DEep Learning Transfer using Feature Map with Attention. Instead of constraining the weights of neural network, DELTA aims at preserving the outer layer outputs of the source network. Specifically, in addition to minimizing the empirical loss, DELTA aligns the outer layer outputs of two networks, through constraining a subset of feature maps that are precisely selected by attention that has been learned in a supervised learning manner. We evaluate DELTA with the state-of-the-art algorithms, including L-2 and L-2-SP. The experiment results show that our method outperforms these baselines with higher accuracy for new tasks. Code has been made publicly available.(1)
更多查看译文
关键词
Transfer learning,framework,algorithms,knowledge distillation
AI 理解论文
溯源树
样例
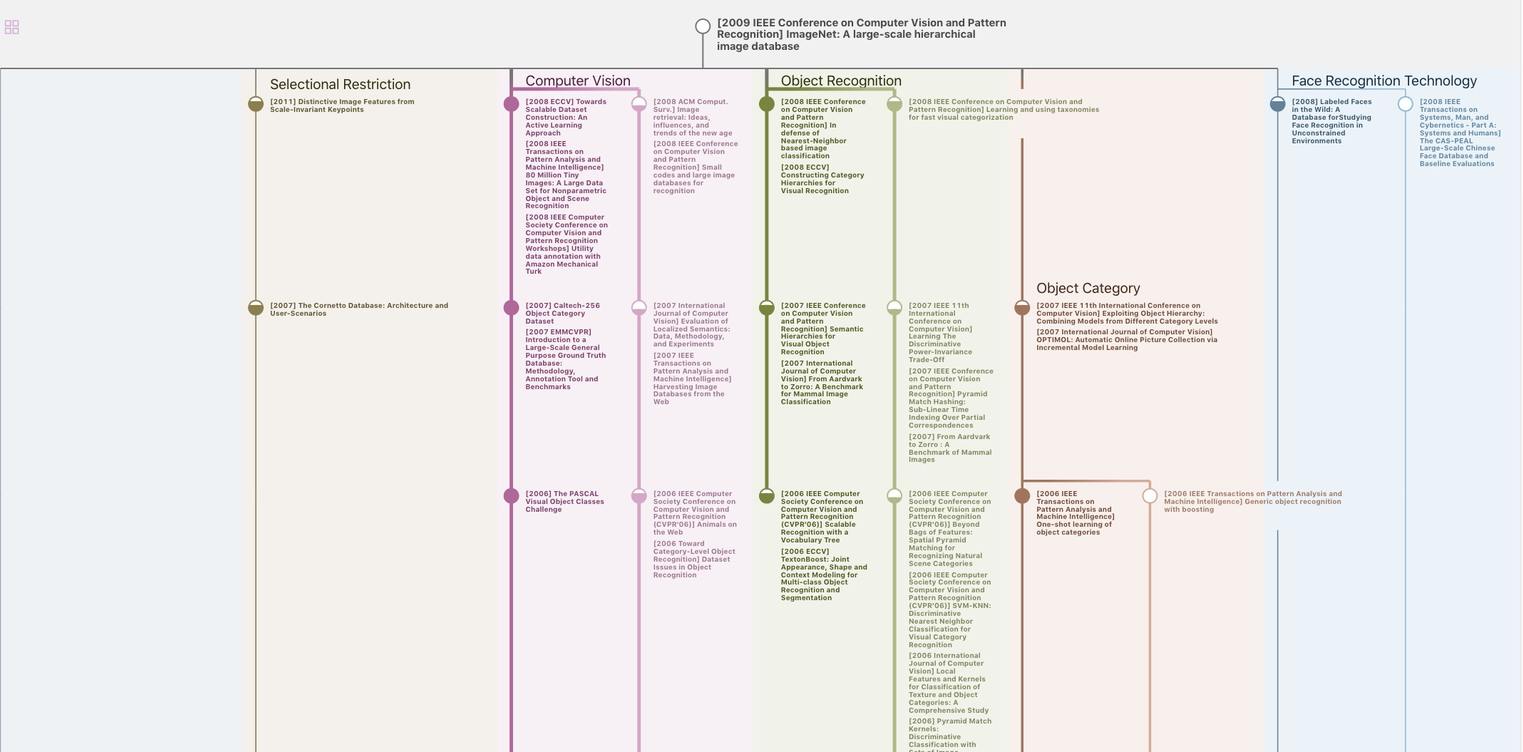
生成溯源树,研究论文发展脉络
Chat Paper
正在生成论文摘要