Session 23: Protein Informatics II
semanticscholar(2004)
摘要
Since the determination of the accessible portions of the human genome, two key points have emerged—first, it is still not certain which regions of the genome code for proteins, and second, the number of discrete protein-coding genes is far fewer than the number of different proteins. This talk will highlight the “post-genomic” issues that proteomics is now addressing and will discuss how these data can be integrated effectively with genomics data. Providing effective bioinformatics solution are key for these very complex data. High-throughput and/or high-output technologies create many challenges, including a lack of common protocols, data formats and representation, the inability to access and understand the information created by other people to avoid repetition of their work, facilitate data comparison, exchange and verification. Consequently, for proteomics to continue its current growth rate, there is a need for new approaches to ease data management and data mining. Oxford Genome Sciences has created a new platform that integrates all clinical, experimental information, experimental expression data and has advanced data pipelines for fusing mass-spectrometry data and summarizes and presents them in a biologically relevant manner. The availability of such bioinformatics solutions are crucial for Proteomics technologies to fulfil their promise of adding further definition to the functional output of the human genome. The “Oxford Genome Anatomy Project” or OGAP will provide a framework for integrating molecular, cellular, phenotypic and clinical information with experimental genetic and proteomics data. OGAP’s aim is to provide a data integration framework for all protein expression data from different platforms such as one and two dimensional gel electrophoresis, ICAT and other LC based techniques and associated biological/clinical information and reference data about genomes, biological pathways and other relevant information to act as a biological reasoning platform. OGAP’s objective is to aide the understanding of the size and diversity of the human proteome at the tissue, disease and protein isoforms levels in a context where it can be readily accessed to biological reasoning. Several models to make OGAP accessible to both academic and commercial R&D will be discussed. 23.11 Deriving Better Specificity Models for Trypsin to Improve Protein Identification by Tandem Mass Spectrometry F. Schütz, E. A. Kapp, R. J. Simpson, and T. P. Speed Division of Genetics and Bioinformatics, WEHI, Parkville, Australia; and Joint Proteomics Laboratory, Ludwig Institute for Cancer Research/WEHI, Parkville, Australia Mass spectrometry is now the method of choice for establishing the identity of a protein from unknown samples. In the bottom-up approach, proteins are digested by an enzyme to produce peptides that are then identified using CID tandem mass spectrometry and database searching. In most cases, the enzyme trypsin is used because the peptides it produces generally fragment in a more predictable manner under electrospray ionization conditions. Trypsin is generally assumed to cleave after Lysine or Arginine residues, except if followed by Proline. Slightly more complicated rules have been devised for predicting trypsin cleavage, however these rules currently only yield binary answers (cleave or no cleavage). Tandem MS database search algorithms use these rules to reduce the number of potential peptides that the algorithm has to consider, thus dramatically reducing the search time. Using a manually curated database of approximately 12,000 tandem MS ESI-IT spectra (hosted by the Joint ProteomicS Laboratory, Melbourne), we have derived new models for deducing the cleavage specificity of trypsin. Instead of a binary prediction, our models yield a score indicating the propensity for cleavage. Using this model, many cleavages that would be considered as being “missed” by trypsin using the rules described above can actually be predicted. While these results are interesting in themselves, they can also be used to improve the identification of proteins by tandem MS and database searching. Until recently, the scores calculated by tandem MS database search algorithms were mainly based on the comparison of an experimental spectrum with sequences from the database. Several groups are now incorporating additional experimental information, such as RP-HPLC retention time and/or pI into the results so as to reduce false-positives and increase the number of true-positives. We will demonstrate how our trypsin models can be used in this context. HUPO 3rd Annual World Congress, October 25–27, Beijing Molecular & Cellular Proteomics 3.10 S261
更多查看译文
AI 理解论文
溯源树
样例
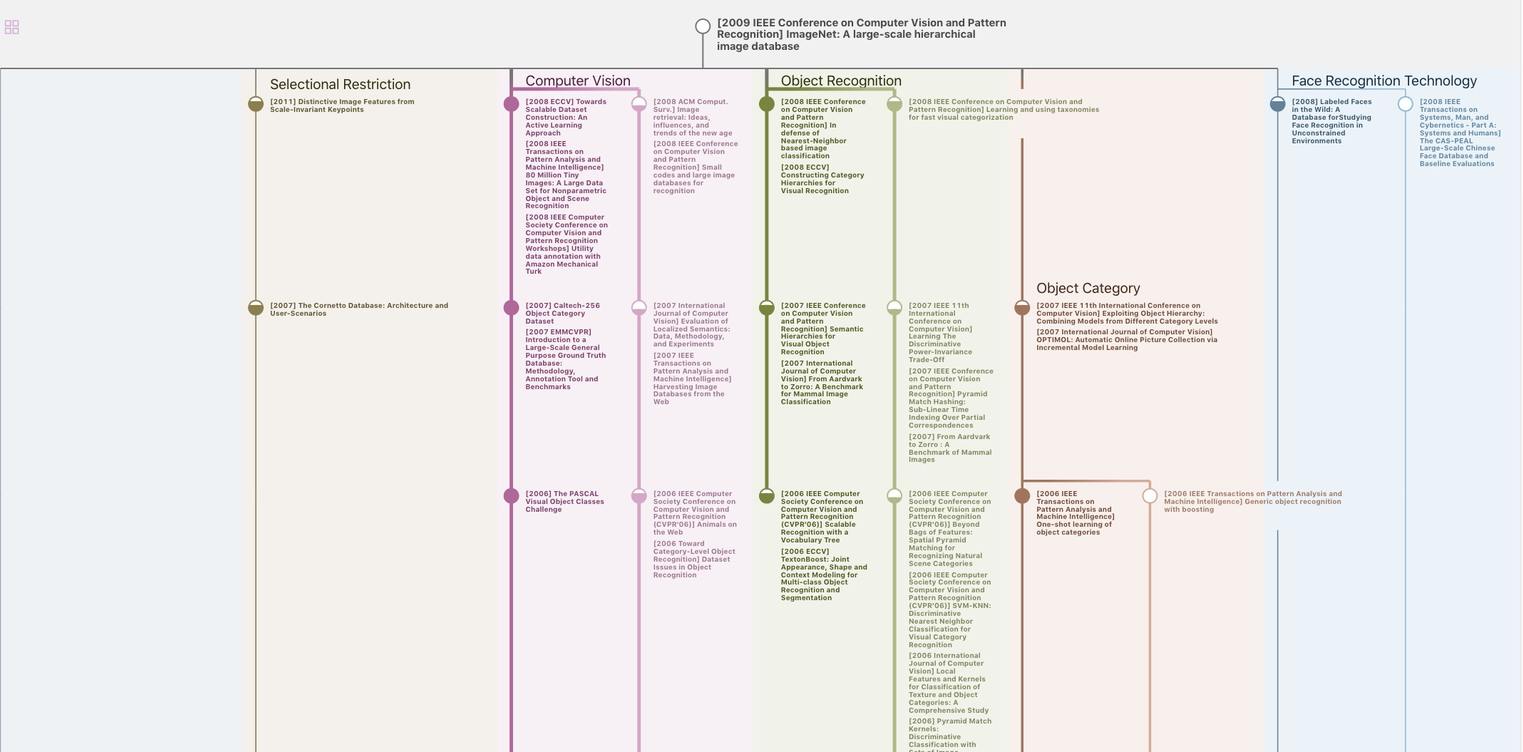
生成溯源树,研究论文发展脉络
Chat Paper
正在生成论文摘要