Dynamic supervisor for cross-dataset object detection
Neurocomputing(2022)
摘要
The application of cross-dataset training in object detection tasks is complicated because the inconsistency in the category range across datasets transforms fully supervised learning into semi-supervised learning. To address this problem, recent studies focus on the generation of high-quality missing annotations. In this study, we first specify that it is not enough to generate high-quality annotations using a single model, which looks only once for annotations. Through detailed experimental analyses, we further conclude that hard-label training is conducive for generating high-recall annotations, whereas soft-label training tends to obtain high-precision annotations. Inspired by the aspects mentioned above, we propose a dynamic supervisor framework that updates the annotations multiple times through multiple-updated submodels trained using hard and soft labels. In the final generated annotations, recall and precision improve significantly through the integration of hard-label training with soft-label training. Extensive experiments conducted on various dataset combination settings support our analyses and demonstrate the superior performance of the proposed dynamic supervisor.
更多查看译文
关键词
Cross-dataset object detection,Hard-label training,Soft-label training,Dynamic ensembling
AI 理解论文
溯源树
样例
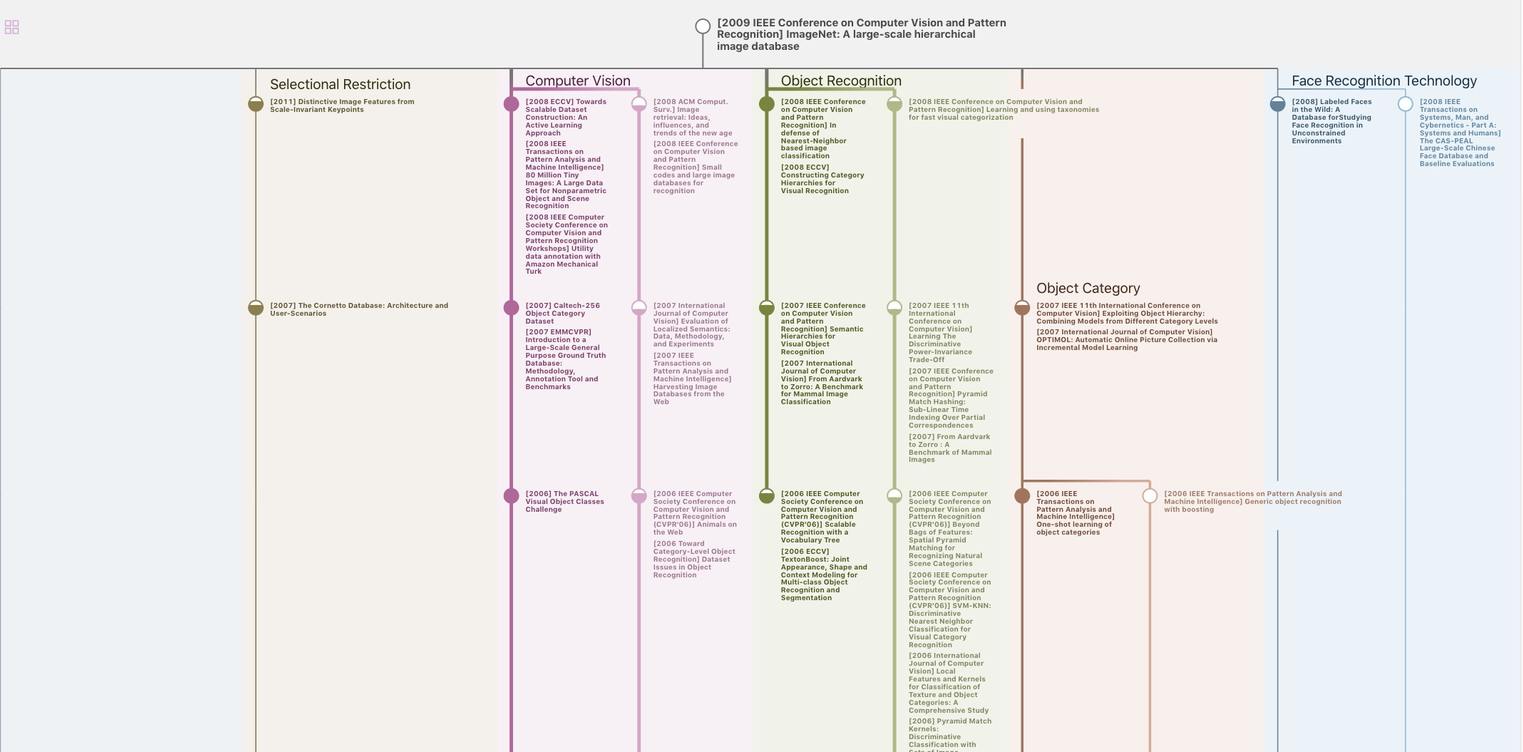
生成溯源树,研究论文发展脉络
Chat Paper
正在生成论文摘要