A novel hierarchical clustering algorithm with merging strategy based on shared subordinates
Applied Intelligence(2021)
摘要
Hierarchical clustering is a common unsupervised learning technique that is used to discover potential relationships in data sets. Despite the conciseness and interpretability, hierarchical clustering algorithms still face some challenges such as inaccuracy, time-consuming, and difficulty in choosing merging strategies. To overcome these limitations, we propose a novel Hierarchical Clustering algorithm with a Merging strategy based on Shared Subordinates (HCMSS), which defines new concepts of the local core representative and the shared subordinate belonging to multiple representatives. First, the state-of-the-art natural neighbor (NaN) is introduced to compute the local neighborhood and the local density of each data point. Next, a sharing-based local core searching algorithm (SLORE) is proposed to find local core points and divide the input data set into numerous initial small clusters. Lastly, these small clusters are merged hierarchically and form the final clustering result. We creatively split the merging process into two sub-steps: first, pre-connecting small clusters according to a shared-subordinates-based indicator that measures the stickiness between clusters; second, merging the pre-connected intermediate clusters and the remaining unconnected small clusters in a classical hierarchical way. Experiments on 8 synthetic and 8 real-world data sets demonstrate that HCMSS can effectively improve the clustering accuracy and is less time-consuming than 2 state-of-the-art benchmarks.
更多查看译文
关键词
Hierarchical clustering,Natural neighbor,Local representatives,Shared subordinates
AI 理解论文
溯源树
样例
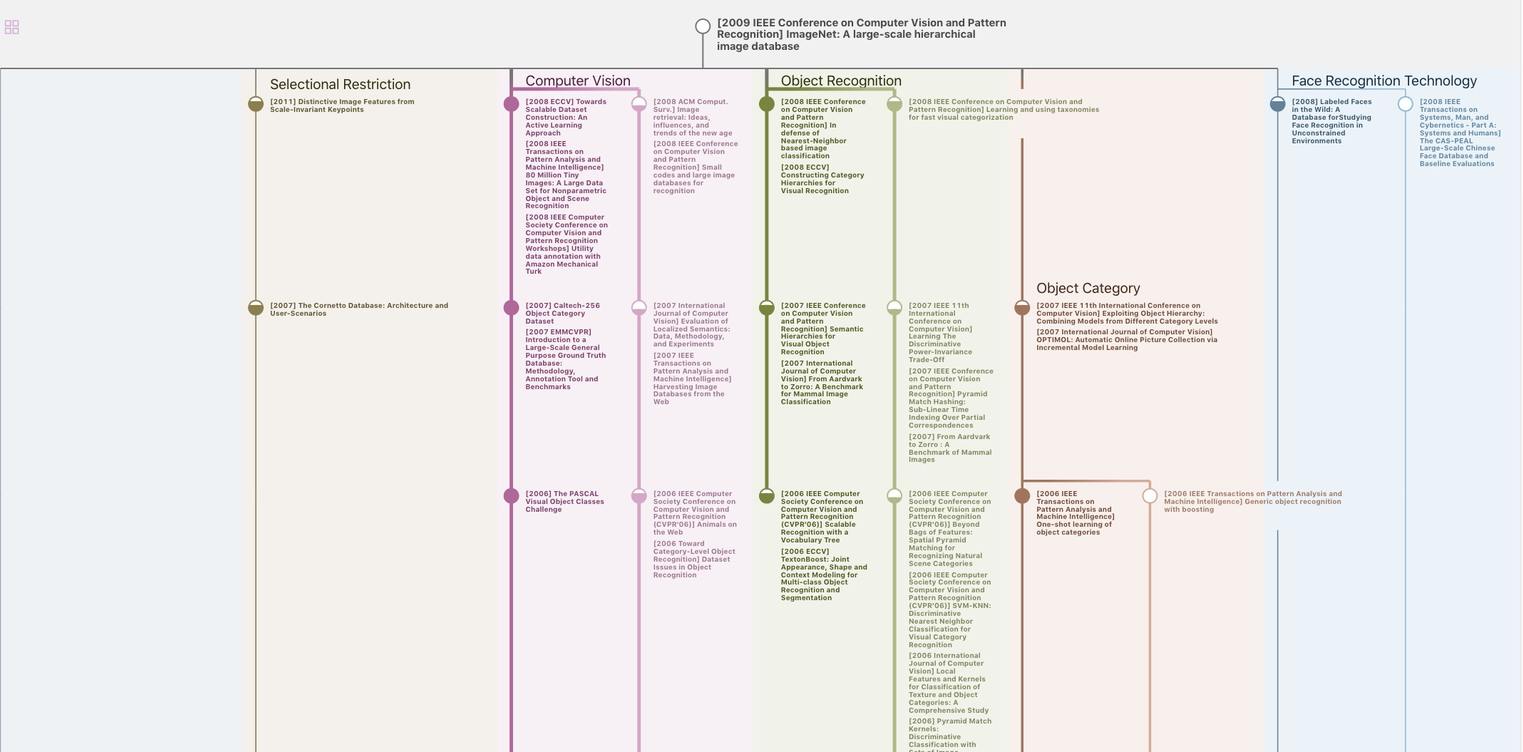
生成溯源树,研究论文发展脉络
Chat Paper
正在生成论文摘要