LiDAR-Based Remote Sensing of the Vertical Profile of Aerosol Liquid Water Content Using a Machine-Learning Model
IEEE TRANSACTIONS ON GEOSCIENCE AND REMOTE SENSING(2022)
摘要
The aerosol liquid water content (ALWC) dictates the hygroscopicity of aerosol particles. To date, measurements of ALWC have been confined primarily to ground-based observations although vertical profiles of ALWC are crucial for understanding its interactions with meteorology. This study proposes a novel method for deriving profiles of ALWC using data acquired by a Light Detection and Ranging (LiDAR), a microwave radiometer, and a suite of aerosol instruments measuring aerosol physical and chemical properties, deployed during a five-month field experiment in Guangzhou, China. The retrieval approach is based on a machine-learning model named the gradient-boosted decision tree model. The inversion accuracy and stability are assessed through comparisons with ALWC data acquired on the ground and at the top of the Guangzhou tower of 532 m above ground. The agreements are encouraging: with the coefficient of determination (R-2) = 0.870 and root-mean-square error (RMSE) = 3.28 mu g . m(-3) for all data; R-2 = 0.776 and RMSE = 2.18 mu g . m(-3) for tower data; and R-2 = 0.872 and RMSE = 4.1 mu g . m(-3) for ground data. From the vertical distribution of the retrieved ALWC in Guangzhou, ALWC is higher in the lower boundary layer, especially when air pollution is severe. The proportion of liquid water in aerosol particles is closely related to the relative humidity in the environment, which will affect the morphology of aerosol particles (with about every 10% increase in liquid water, the depolarization ratio decreases by 0.02). The model may be of general use for studying air pollution and secondary aerosol generation.
更多查看译文
关键词
Aerosol liquid water content (ALWC),Light Detection and Ranging (LiDAR),machine learning (ML),vertical profile
AI 理解论文
溯源树
样例
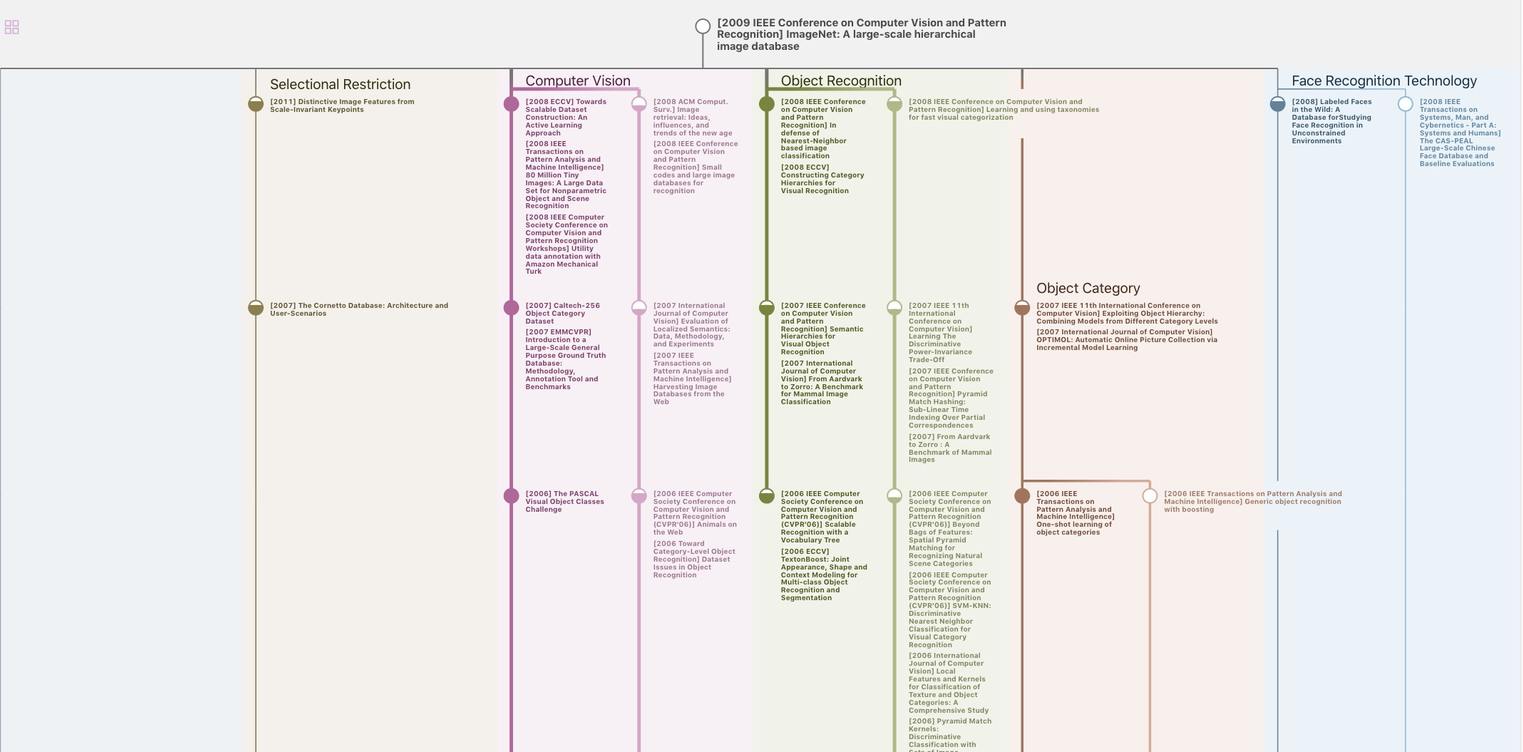
生成溯源树,研究论文发展脉络
Chat Paper
正在生成论文摘要