Deep Learning based cloud parametrization for the Community Atmosphere Model
semanticscholar(2020)
Despite significant progress in climate modeling over the last decades, the spread in simulated equilibrium climate sensitivity, i.e. the change in global mean surface temperature after a doubling of atmospheric CO2 concentrations, has not decreased since the 1970s. Estimates from the Coupled Model Intercomparison Project Phase 5 (CMIP5) range between 2.1 and 4.7°C, and this range is even larger in CMIP6. A large contribution to this uncertainty stems from differences in the representation of clouds and convection occurring at scales smaller than the resolved model grid resolution. Machine learning approaches have recently been shown to be potentially powerful alternatives to physical parametrizations of convection (Gentine et al. 2018; Rasp et al. 2018). Despite improving understanding of the minimization of generalization errors in out-of-sample climate states (Beucler et al. 2019) these approaches struggle to capture the full variability observed in the training and validation data. First experiments with autoencoder-decoder structures illustrate a compatible skill in comparison to feedforward neural networks.
In this study we modify the existing neural network parametrization using more advanced neural network structures. The new parametrization is tested with the Community Atmosphere Model Version 3 for different climate states. This work is a contribution to the development of complex subgrid-scale convective and turbulence parametrizations performed within the recently awarded European Research Council (ERC) Synergy Grant “Understanding and modelling the Earth system with machine learning (USMILE)”.
References:
Gentine, P., Pritchard, M.S., Rasp, S., Reinaudi, G., & Yacalis, G. (2018): Could Machine Learning Break the Convection Parameterization Deadlock? Geophysical Research Letters, 45, 5742-5751
Rasp, S., Pritchard, M.S., & Gentine, P. (2018): Deep learning to represent subgrid processes in climate models. Proceedings of the National Academy of Sciences
Beucler, T., Rasp, S., Pritchard, M.S., Gentine, P.,Ott, J. & Baldi, P. (2019): Enforcing Analytic Constraints in Neural-Networks Emulating Physical Systems. Physical Review Letters, https://arxiv.org/abs/1909.00912
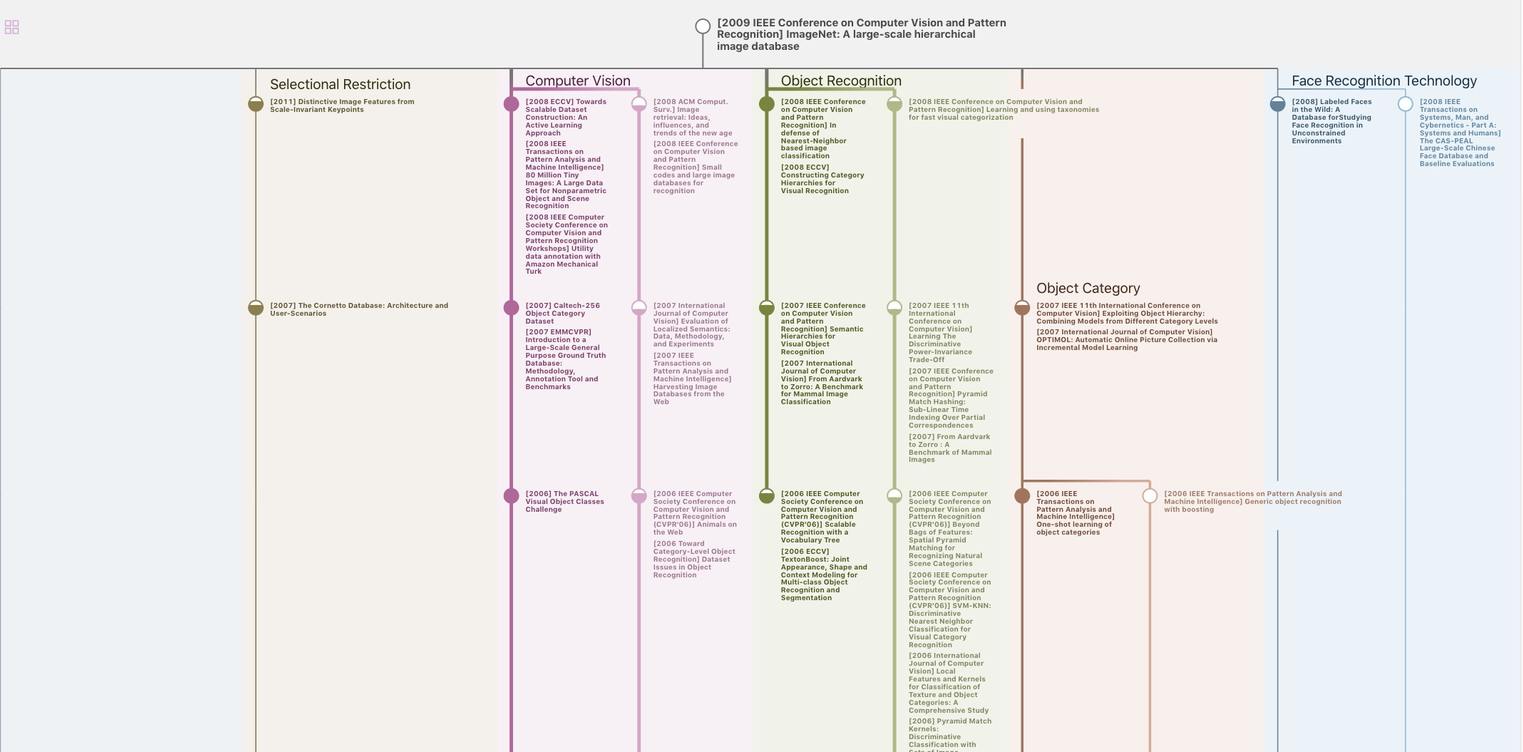