New Generation Iterative Solvers in LS-DYNA®
semanticscholar(2020)
摘要
Iterative solvers for sparse linear systems are used as the default option in a few places in LS-DYNA, e.g., thermal analysis and incompressible fluid flow. They are also available as a non-default option for implicit mechanics, electromagnetics, and acoustics. Until now, our suite of iterative solvers was limited to a few simple solvers (Conjugate Gradients and GMRES), and a few simple preconditioners. We recall that a preconditioner is an approximate inverse of the matrix (e.g., the stiffness matrix) that aims at improving convergence. Our preconditioners were limited to simple techniques like diagonal scaling and basic domain decomposition techniques that discard the coupling terms between processors, in MPP. Problems from customers are growing faster than memory size, making it difficult to use direct solvers. They are also often too numerically challenging to use simple iterative solvers, in particular in implicit mechanics. This has pushed us to revisit our suite of iterative solvers and preconditioners. In particular, we have been investigating the use of Block Low-Rank factorizations (BLR) and the use of Algebraic Multigrid (AMG). In the talk, we will compare these new options across all the different applications that make use of linear solvers. We will discuss convergence, memory usage, and scalability. For end users, the takeaway will be a better understanding of which solver options to use for different kinds of problems, and what to expect from them.
更多查看译文
AI 理解论文
溯源树
样例
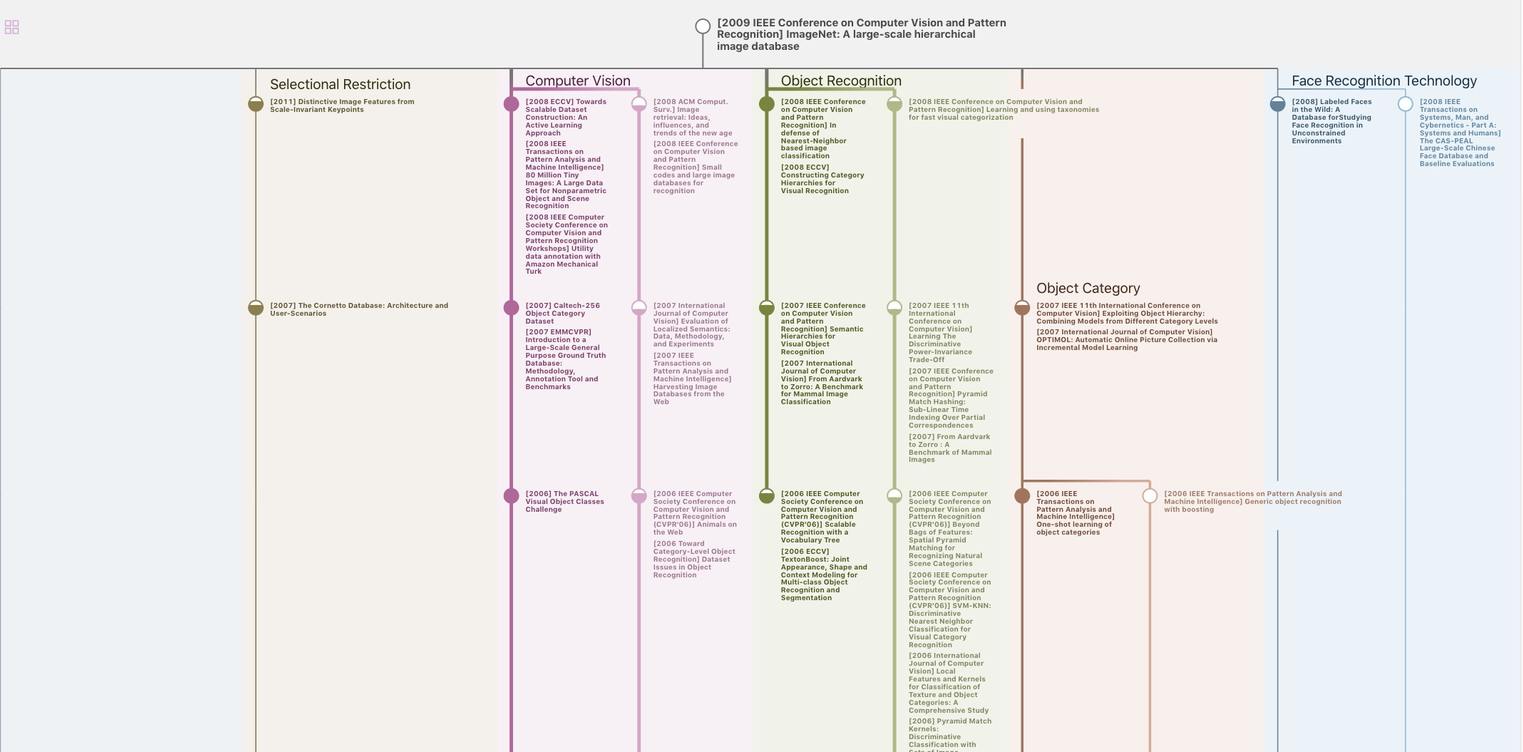
生成溯源树,研究论文发展脉络
Chat Paper
正在生成论文摘要