Generative Adversarial Network using Perturbed-Convolutions
ArXiv(2021)
摘要
Despite growing insights into the GAN training, it still suffers from instability during the training procedure. To alleviate this problem, this paper presents a novel convolutional layer, called perturbed-convolution (PConv), which focuses on achieving two goals simultaneously: penalize the discriminator for training GAN stably and prevent the overfitting problem in the discriminator. PConv generates perturbed features by randomly disturbing an input tensor before performing the convolution operation. This approach is simple but surprisingly effective. First, to reliably classify real and generated samples using the disturbed input tensor, the intermediate layers in the discriminator should learn features having a small local Lipschitz value. Second, due to the perturbed features in PConv, the discriminator is difficult to memorize the real images; this makes the discriminator avoid the overfitting problem. To show the generalization ability of the proposed method, we conducted extensive experiments with various loss functions and datasets including CIFAR-10, CelebA-HQ, LSUN, and tiny-ImageNet. Quantitative evaluations demonstrate that WCL significantly improves the performance of GAN and conditional GAN in terms of Frechet inception distance (FID). For instance, the proposed method improves FID scores on the tiny-ImageNet dataset from 58.59 to 50.42.
更多查看译文
关键词
adversarial,perturbed-convolutions
AI 理解论文
溯源树
样例
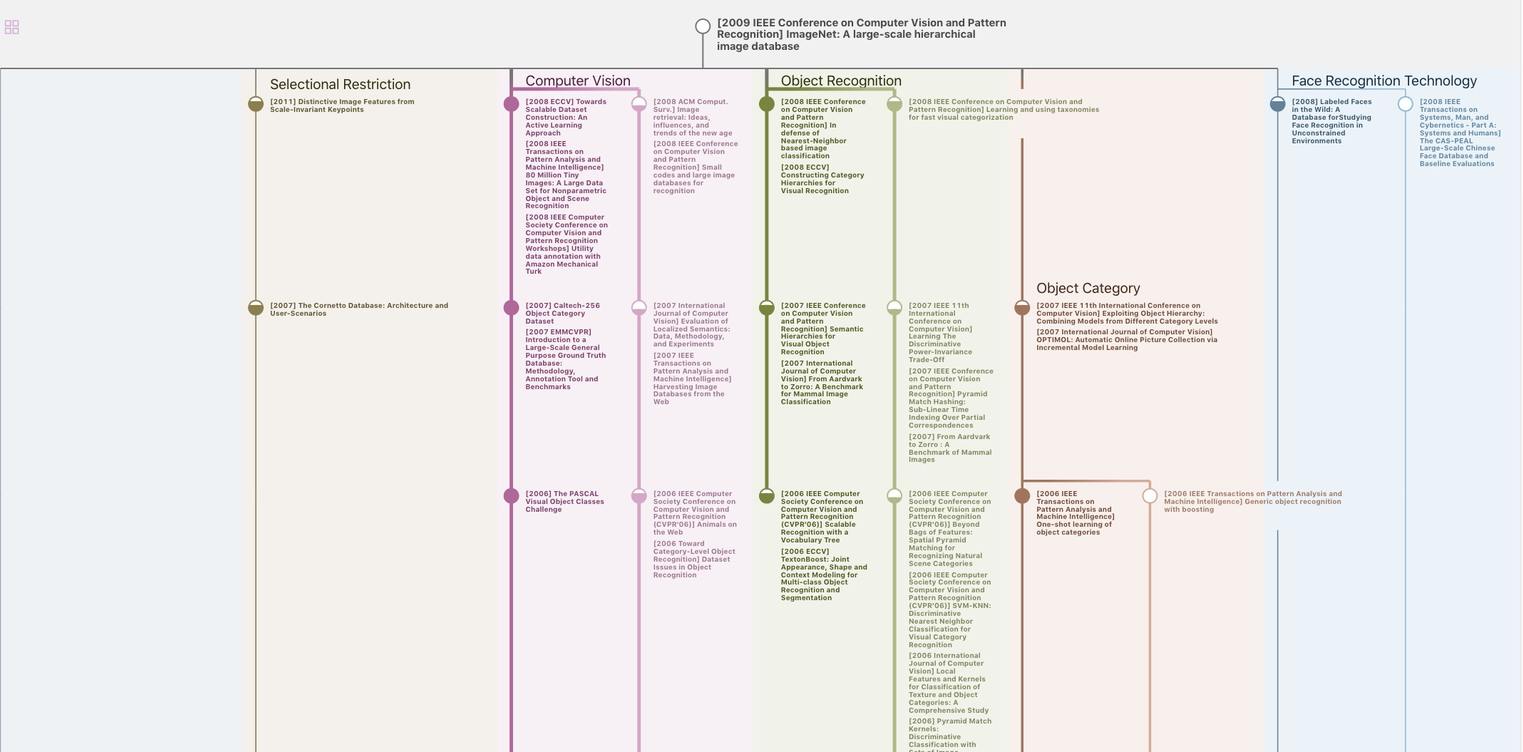
生成溯源树,研究论文发展脉络
Chat Paper
正在生成论文摘要