Large enough to matter, too small to be of consequence, or insufficient evidence? A Unified Framework Combining Minimum-Effect Significance Testing and Equivalence Testing
semanticscholar(2021)
摘要
Is an effect found in a sample large enough to warrant inferring that the true effect in the population is large enough to be meaningful? Or is it small enough to warrant inferring that the true effect in the population is too small to be meaningful? Or does the effect not offer clear enough evidence to support either inference? This article outlines a simple yet versatile approach for answering these questions by overcoming the hurdles that prevented Minimum Effect Significance Testing (MEST) from being used widely, and by integrating it with Equivalence Testing (EqT) in a unified framework.
更多查看译文
AI 理解论文
溯源树
样例
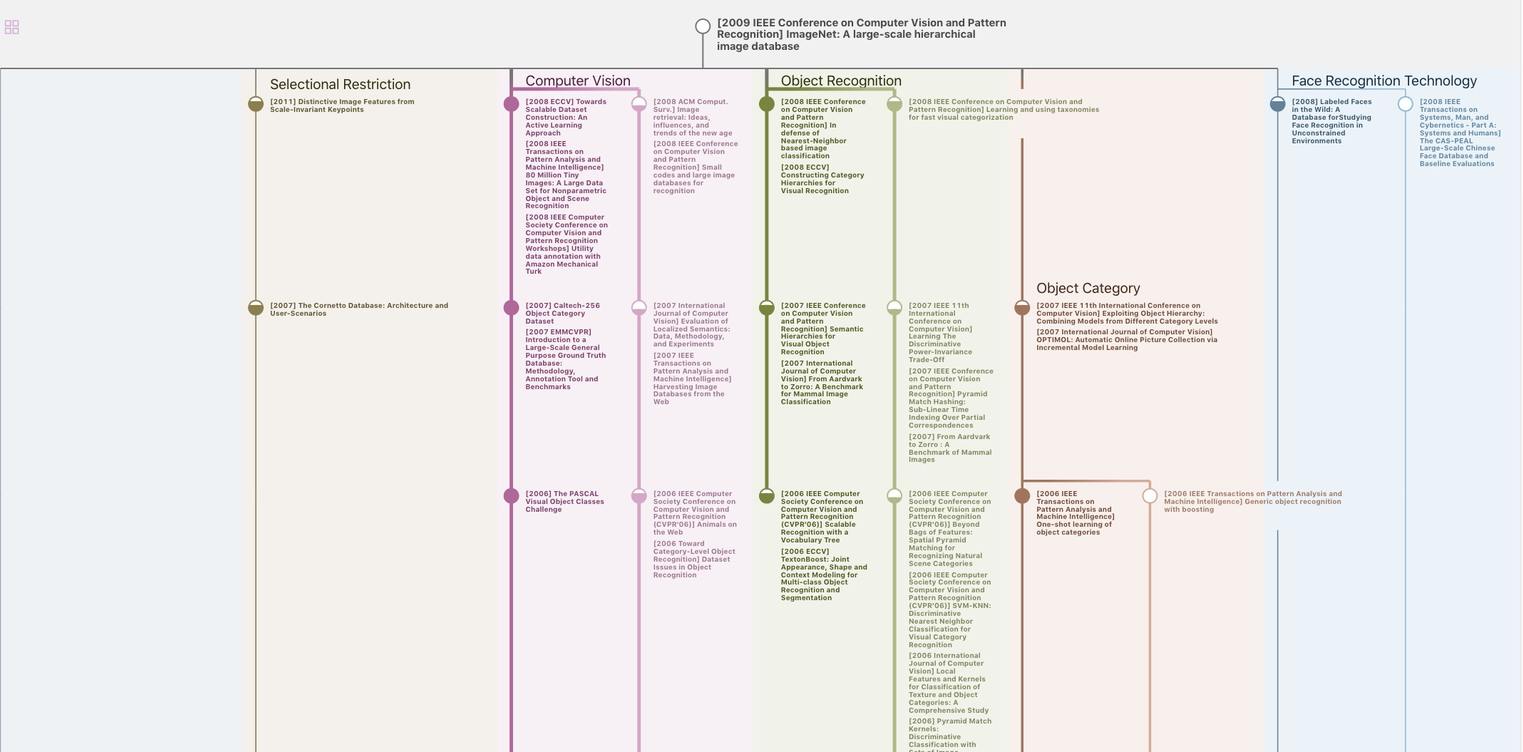
生成溯源树,研究论文发展脉络
Chat Paper
正在生成论文摘要