HiGrad: Uncertainty Quantification for Online Learning and Stochastic Approximation
JOURNAL OF MACHINE LEARNING RESEARCH(2023)
摘要
Stochastic gradient descent (SGD) is an immensely popular approach for online learn-ing in settings where data arrives in a stream or data sizes are very large. However, despite an ever-increasing volume of work on SGD, much less is known about the statistical in-ferential properties of SGD-based predictions. Taking a fully inferential viewpoint, this paper introduces a novel procedure termed HiGrad to conduct statistical inference for on-line learning, without incurring additional computational cost compared with SGD. The HiGrad procedure begins by performing SGD updates for a while and then splits the single thread into several threads, and this procedure hierarchically operates in this fashion along each thread. With predictions provided by multiple threads in place, a t-based confidence interval is constructed by decorrelating predictions using covariance structures given by a Donsker-style extension of the Ruppert-Polyak averaging scheme, which is a technical contribution of independent interest. Under certain regularity conditions, the HiGrad con-fidence interval is shown to attain asymptotically exact coverage probability. Finally, the performance of HiGrad is evaluated through extensive simulation studies and a real data example. An R package higrad has been developed to implement the method.
更多查看译文
关键词
HiGrad,stochastic gradient descent,online learning,stochastic approximation,Ruppert-Polyak averaging,uncertainty quantification,t-confidence interval
AI 理解论文
溯源树
样例
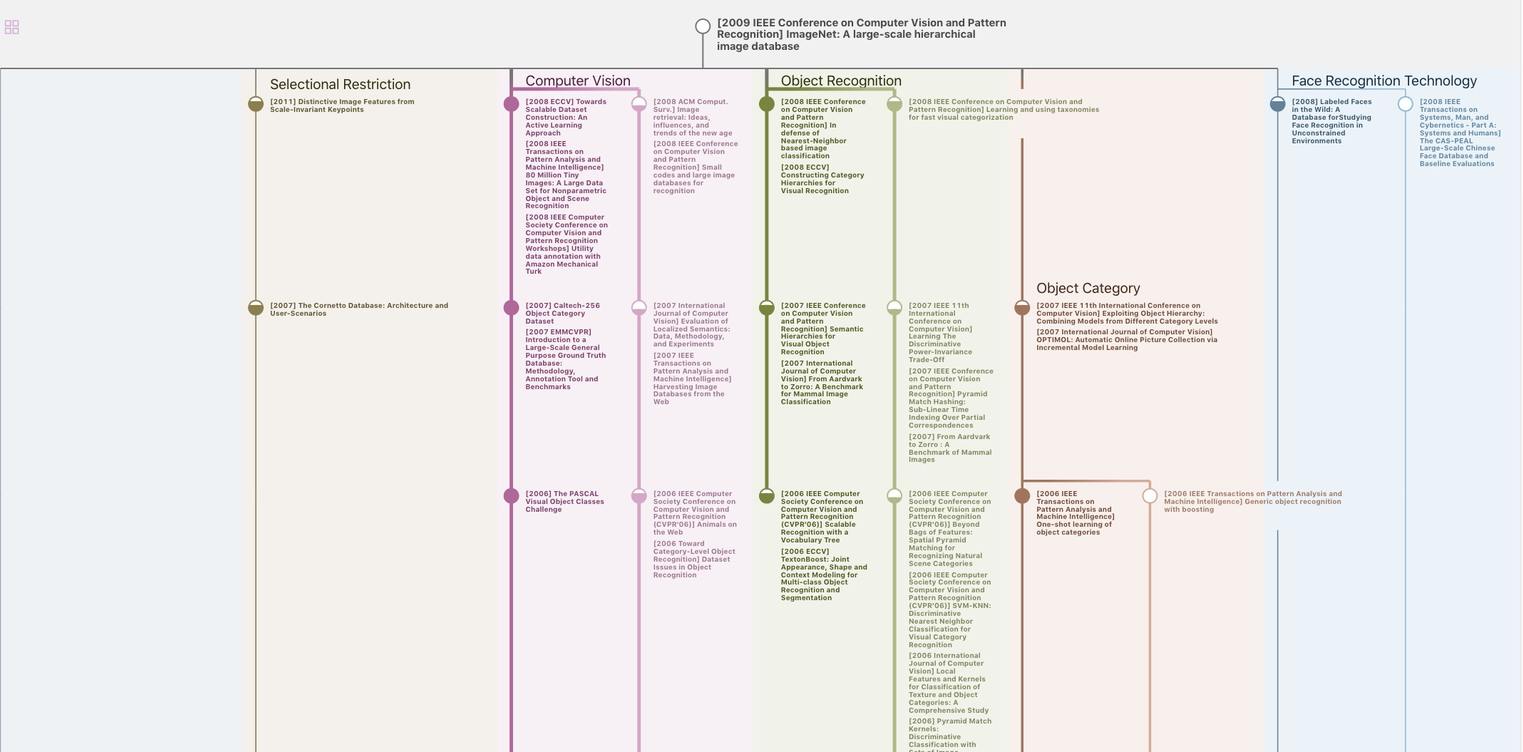
生成溯源树,研究论文发展脉络
Chat Paper
正在生成论文摘要