Satellite Aerosol Retrieval Using Scene Simulation and Deep Belief Network
IEEE TRANSACTIONS ON GEOSCIENCE AND REMOTE SENSING(2022)
摘要
Aerosol satellite remote sensing retrieval is of great importance in the study of climatic and environmental effects. However, due to varied factors affecting the signals reaching satellite sensors, accurate aerosol retrieval remains challenging. Focusing on limitations in the availability of aerosol data from real scenes, we simulated real scenes to obtain sample data to achieve aerosol retrieval using a deep belief network (DBN). The 6S atmospheric radiative transfer model was used to simulate various possible parameters of earth-atmosphere, sun, and sensor in real scenes. A large amount of simulated data was generated and used as sample datasets of DBN training to obtain the aerosol inversion model. Moderate Resolution Imaging Spectroradiometer (MODIS) data were used to perform aerosol optical thickness (AOT) retrieval experiments. Global-scale aerosol retrieval experiments were conducted based on the following three representative regions: 1) Beijing-Tianjin-Hebei region in China; 2) Midwestern and Southern United States; and 3) Central and Western Europe. The retrieval results were verified using Aerosol Robotic Network (AERONET) datasets in comparison with MCD19A2 aerosol products. Five indicators, including mean absolute error (MAE) and within expected error (f $_{=EE}$ ), were used for the evaluation. The evaluation indicators of the proposed method, in which MAEs were 0.0626, 0.0366, and 0.0487, and f $_{=EE}$ 's were 86.28%, 85.21%, and 80.71%, performed better than MCD19A2 in three typical regions. The most significant advantage of the proposed method is that high-precision retrieval of spatially continuous AOT can be achieved using single-temporal satellite imagery data, which is not possible realizing in current aerosol retrieval methods.
更多查看译文
关键词
Aerosols,Satellites,Atmospheric modeling,Remote sensing,Sensors,Vegetation mapping,MODIS,Aerosol retrieval,deep belief network (DBN),deep learning (DL) algorithm,Moderate Resolution Imaging Spectroradiometer (MODIS),scene simulation
AI 理解论文
溯源树
样例
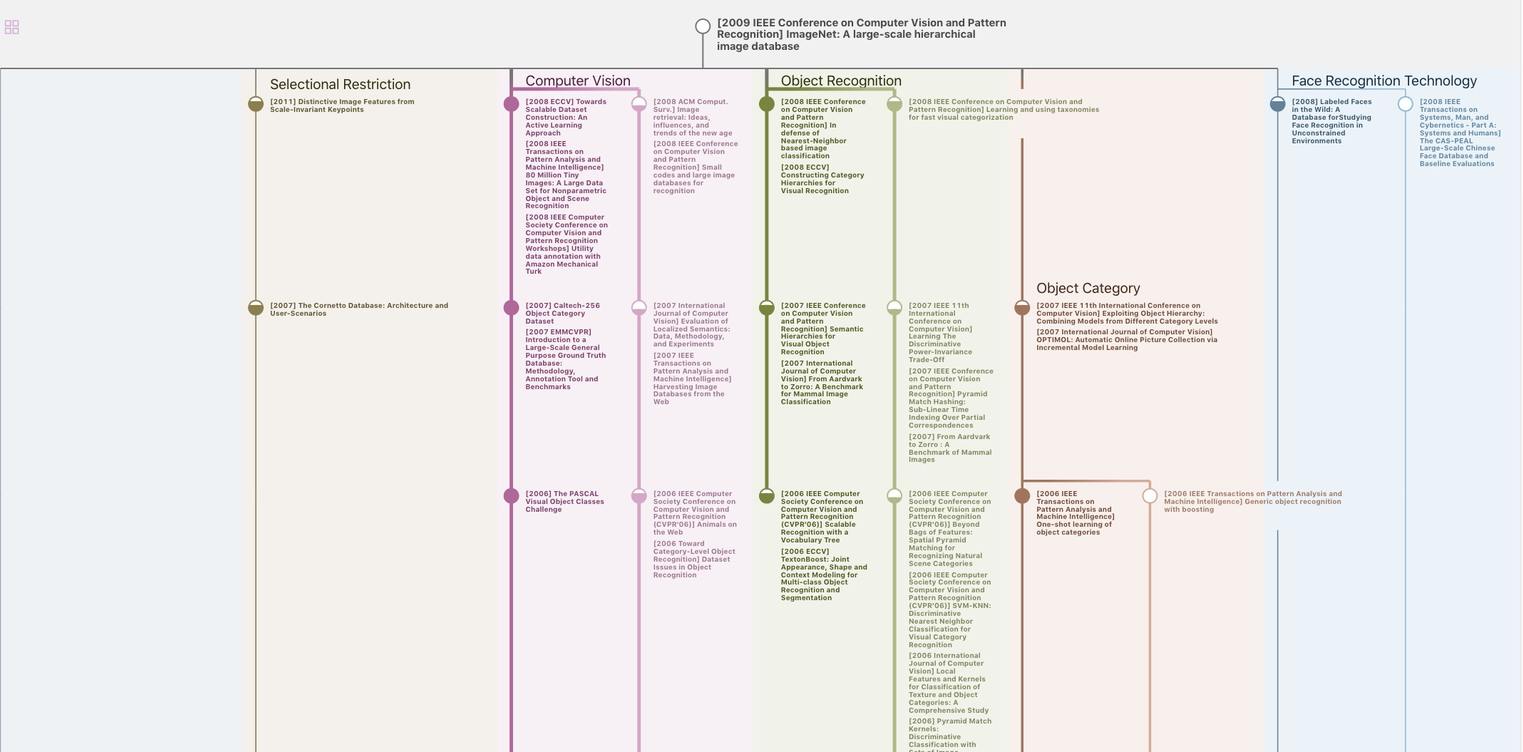
生成溯源树,研究论文发展脉络
Chat Paper
正在生成论文摘要