PEN: Process Estimator neural Network for root cause analysis using graph convolution
JOURNAL OF MANUFACTURING SYSTEMS(2022)
摘要
Root cause analysis in modern multistage assembly lines is a challenging, yet widely used technique to increase the product quality. Improvements - due to Industry 4.0 - aim for near-zero-defects manufacturing. Thus, we propose a novel root cause analysis: the Process Estimator neural Network (PEN) to solve the sparse, nonlinear problem of the state-space model empowering a graph convolution neural network. The contributions of this paper are: (1) study a novel problem of utilizing nonlinear deep neural networks to solve the state-space model; (2) elaborating the use of a graph convolution neural network to scope with the current limitations of linear approaches, which cannot process dense 3D point cloud data of the outer skin of the product; (3) how to analyze the trained network for fine tuning. We showed through a realistic experiment how PEN performs on huge 3D point clouds (188.000 points or higher) in form of meshed CAD models of first-order shell elements. These ex-periments set an example on how to overcome the fundamental performance limitations of current linear approaches.
更多查看译文
关键词
Root cause analysis,Variation source identification,Graph convolution neural network
AI 理解论文
溯源树
样例
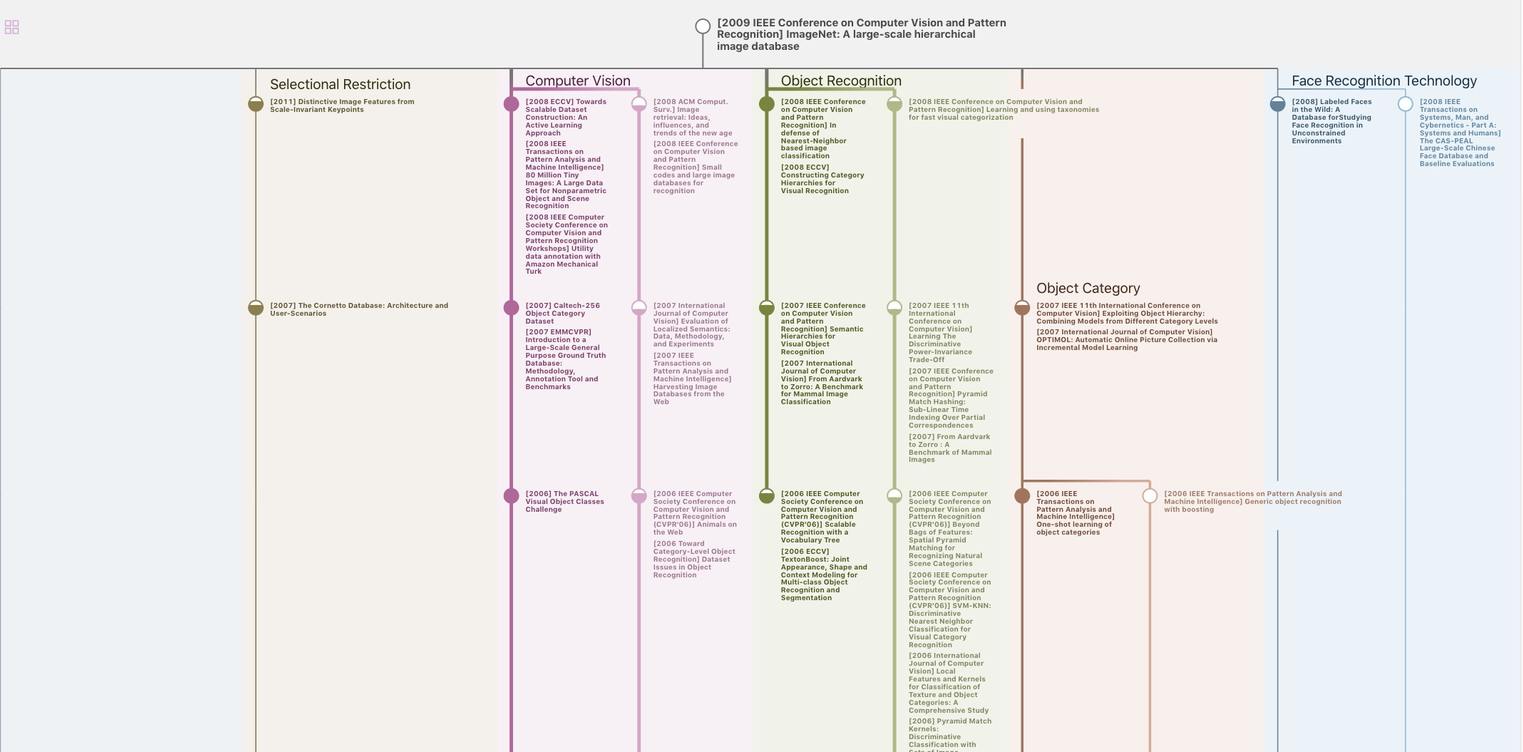
生成溯源树,研究论文发展脉络
Chat Paper
正在生成论文摘要