InSAR Phase Unwrapping by Deep Learning Based on Gradient Information Fusion
IEEE GEOSCIENCE AND REMOTE SENSING LETTERS(2022)
摘要
Phase unwrapping (PhU) is an important step in interferometric synthetic aperture radar (InSAR) technology. At present, difficulties are encountered when using deep learning to solve the PhU problem because the fringe density of the actual interferogram varies, resulting in an imbalanced class of semantic segmentation. Deep learning cannot completely use gradient information, and it is difficult to address a large number of residues. In this letter, a PhU semantic segmentation model based on gradient information fusion and improved PhaseNet network is proposed to solve the problem of imbalanced classification and error propagation. 21 613 pairs of phase samples are constructed by using simulated and real Sentinel-1 InSAR Data. The experimental results show that the average classification accuracy of the method can reach 97%, and the mean square error is only 0.97. The average processing speed of 256 x 256 slices is only 0.5 s. Compared with the traditional methods and other deep learning methods, this method solves the problem of classification imbalance, and the use of fusion gradient information improves the efficiency of the algorithm as well as reduces the burden of network classification and the error propagation, showing increased robustness in the case of many residues and high fringe density.
更多查看译文
关键词
Deep learning,interferometric synthetic aperture radar (InSAR),phase unwrapping (PhU),semantic segmentation
AI 理解论文
溯源树
样例
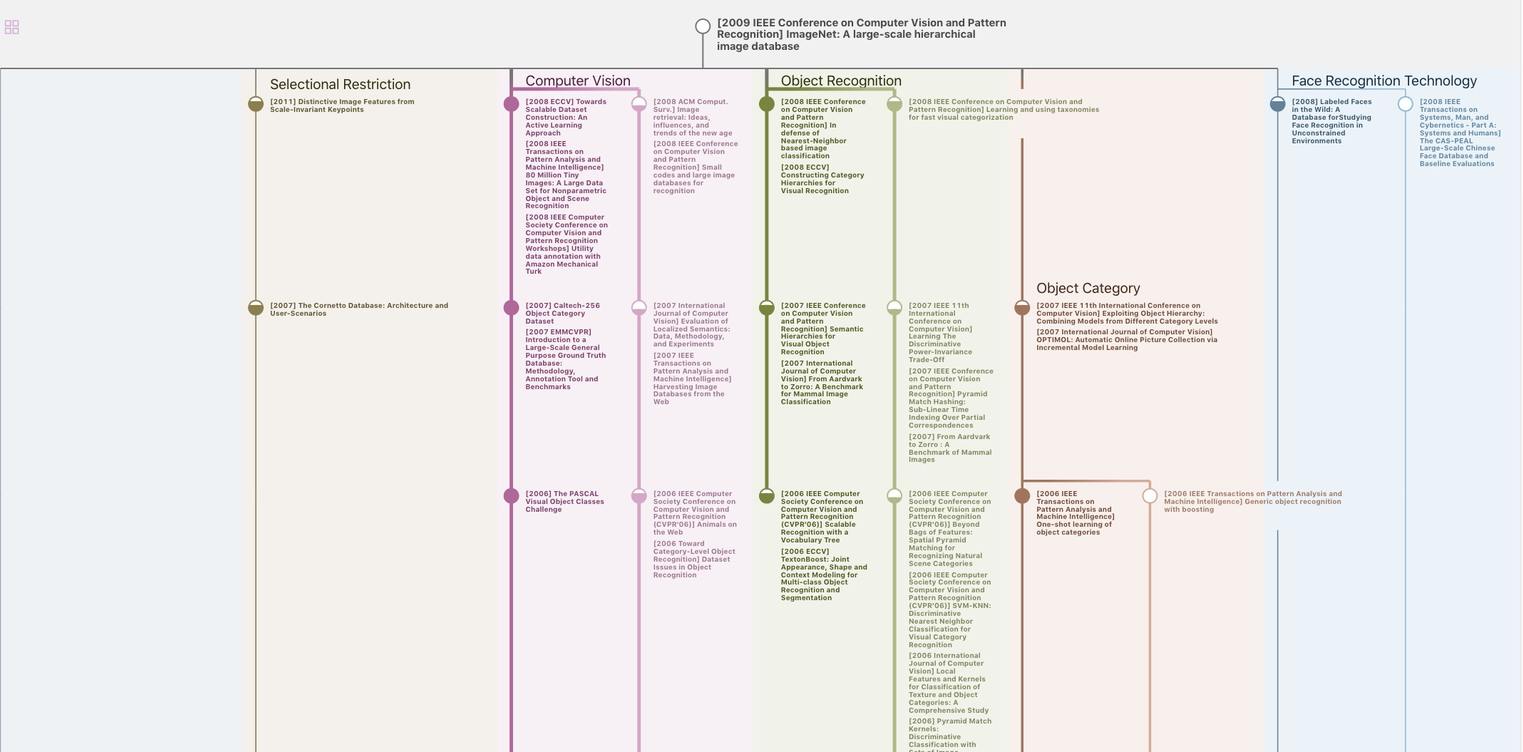
生成溯源树,研究论文发展脉络
Chat Paper
正在生成论文摘要