Machine Learning Inversion for Single-Baseline P-Band Polarimetric SAR Interferometry
IEEE GEOSCIENCE AND REMOTE SENSING LETTERS(2022)
摘要
This letter proposes a machine learning inversion scheme for P-band polarimetric interferometric synthetic aperture radar (Pol-InSAR), which can achieve the single-baseline random volume over ground (RVoG) model inversion without the assumption of the null ground-to-volume ratio. First, the potential variables—including the incidence angle and the PDHigh coherence acquired with phase diversity optimization—that are related to the forest vertical structure are analyzed for their correlations with the extinction coefficient in the RVoG model. Then, the machine learning approach is applied to forecast the extinction coefficient characterized by those potential variables. Ultimately, in the case of fixing the extinction coefficient, the forest height is estimated by a geometric process on the complex plane. The actual Pol-InSAR data verification illustrates that the inversion performance of the proposed scheme overmatches that of the traditional schemes.
更多查看译文
关键词
Forestry,Machine learning,Coherence,Solid modeling,Predictive models,Synthetic aperture radar,Backscatter,Forest vertical structure,machine learning,P-band polarimetric interferometric synthetic aperture radar (Pol-InSAR),random volume over ground (RVoG) model
AI 理解论文
溯源树
样例
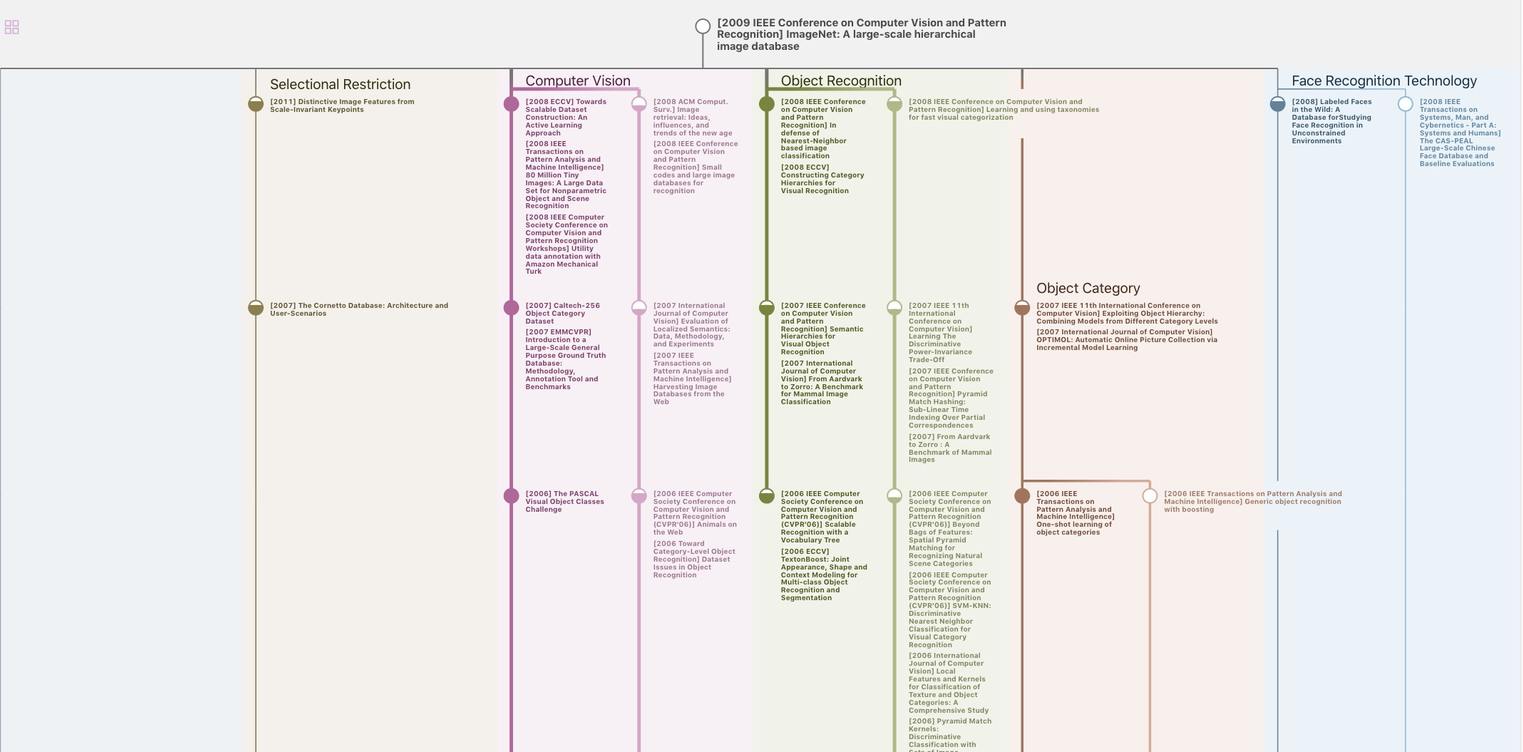
生成溯源树,研究论文发展脉络
Chat Paper
正在生成论文摘要