Defending Against Imperceptible Audio Adversarial Examples Using Proportional Additive Gaussian Noise
semanticscholar(2020)
摘要
Neural networks are susceptible to adversarial examples, which are specific inputs to a network that result in a misclassification or an incorrect output. While most past work has focused on methods to generate adversarial examples to fool image classification networks, recently, similar attacks on automatic speech recognition systems have been explored. Due to the relative novelty of these audio adversarial examples, there exist few robust defenses for these attacks. We present a robust defense for inaudible or imperceptible audio adversarial examples. This approach mimics the adversarial strategy to add targeted proportional additive Gaussian noise in order to revert an adversarial example back to its original transcription. Our defense performs similarly to other defenses yet is the first randomized or probabilistic strategy. Additionally, we demonstrate the challenges that arise when applying defenses against adversarial examples for images to audio adversarial examples.
更多查看译文
AI 理解论文
溯源树
样例
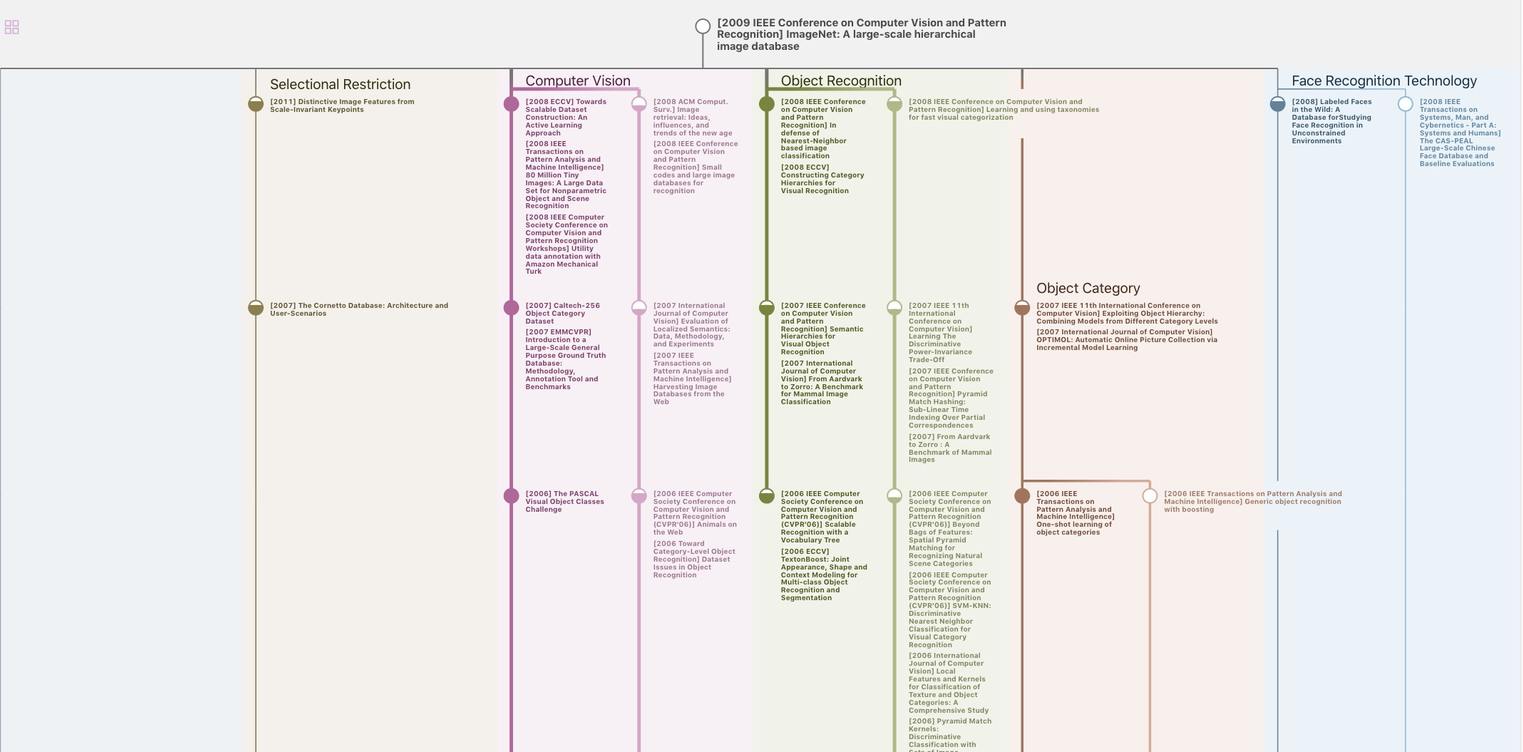
生成溯源树,研究论文发展脉络
Chat Paper
正在生成论文摘要