HAT: Hierarchical Alternative Training for Long Range Policy Transfer
semanticscholar(2020)
摘要
Humans can master a new task within a few trials by drawing upon skills acquired through prior experience. To mimic this capability, hierarchical models combining primitive policies learned from prior tasks have been proposed. However, these methods fail short comparing to the human’s range of transferability. We propose Hierarchical Alternative Training (HAT), which leverages the hierarchical structure to train the combination function and adapt the primitive polices alternatively, to efficiently produce a range of complex behaviors on challenging new tasks. We demonstrate that our method outperforms recent policy transfer methods by combining and adapting these reusable primitives in tasks with continuous action space. The experiment results further show that HAT provides a broader transferring range.
更多查看译文
AI 理解论文
溯源树
样例
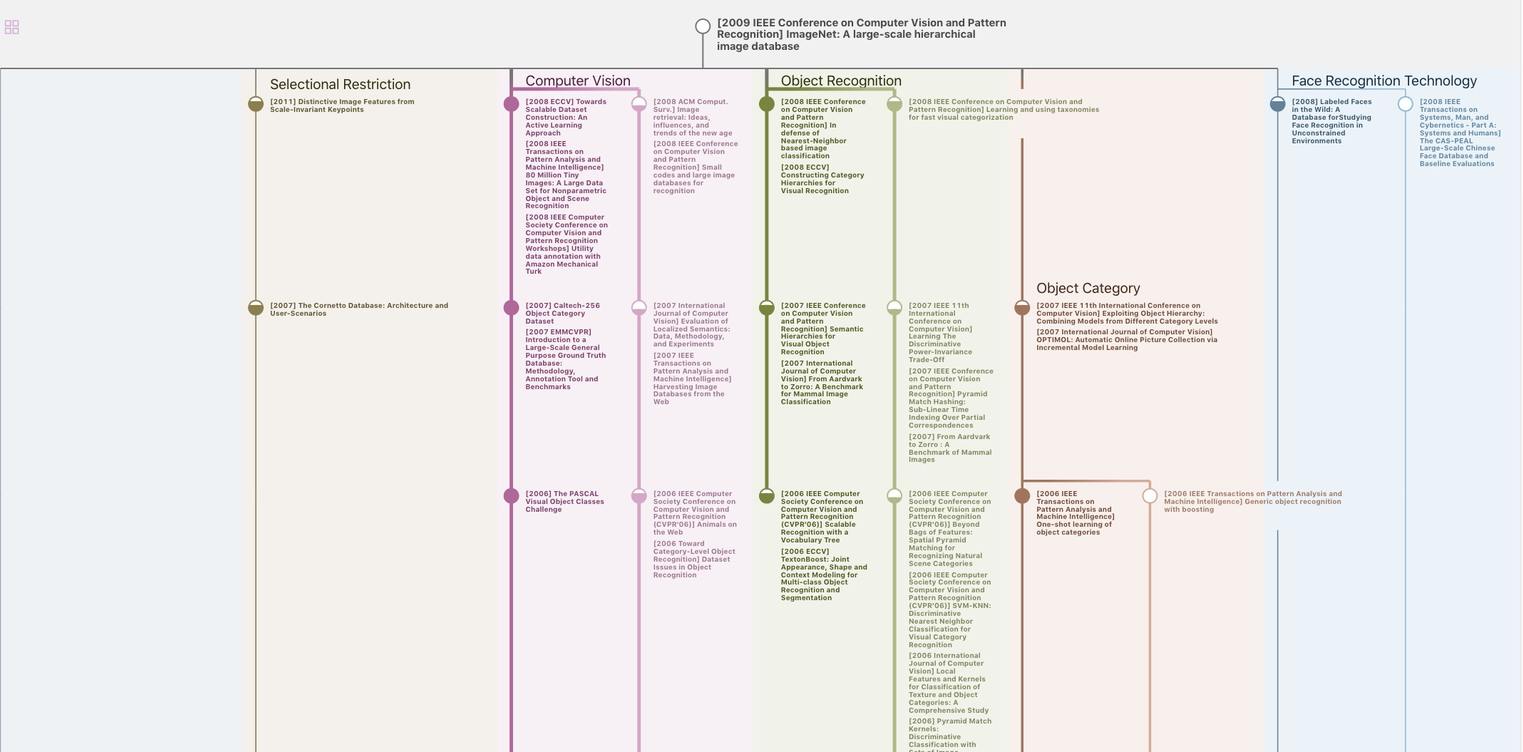
生成溯源树,研究论文发展脉络
Chat Paper
正在生成论文摘要