Supervised Contrastive Learning-Supplementary Material
semanticscholar(2020)
摘要
In Fig. 1, we compare the training setup for the cross-entropy, self-supervised contrastive and supervised contrastive (SupCon) losses. Note that the number of parameters in the inference models always stays the same. We also note that it is not necessary to train a linear classifier in the second stage, and previous works have used k-Nearest Neighbor classification [12] or prototype classification to evaluate representations on classification tasks. The linear classifier can also be trained jointly with the encoder, as long as it doesn’t propagate gradients back to the encoder.
更多查看译文
AI 理解论文
溯源树
样例
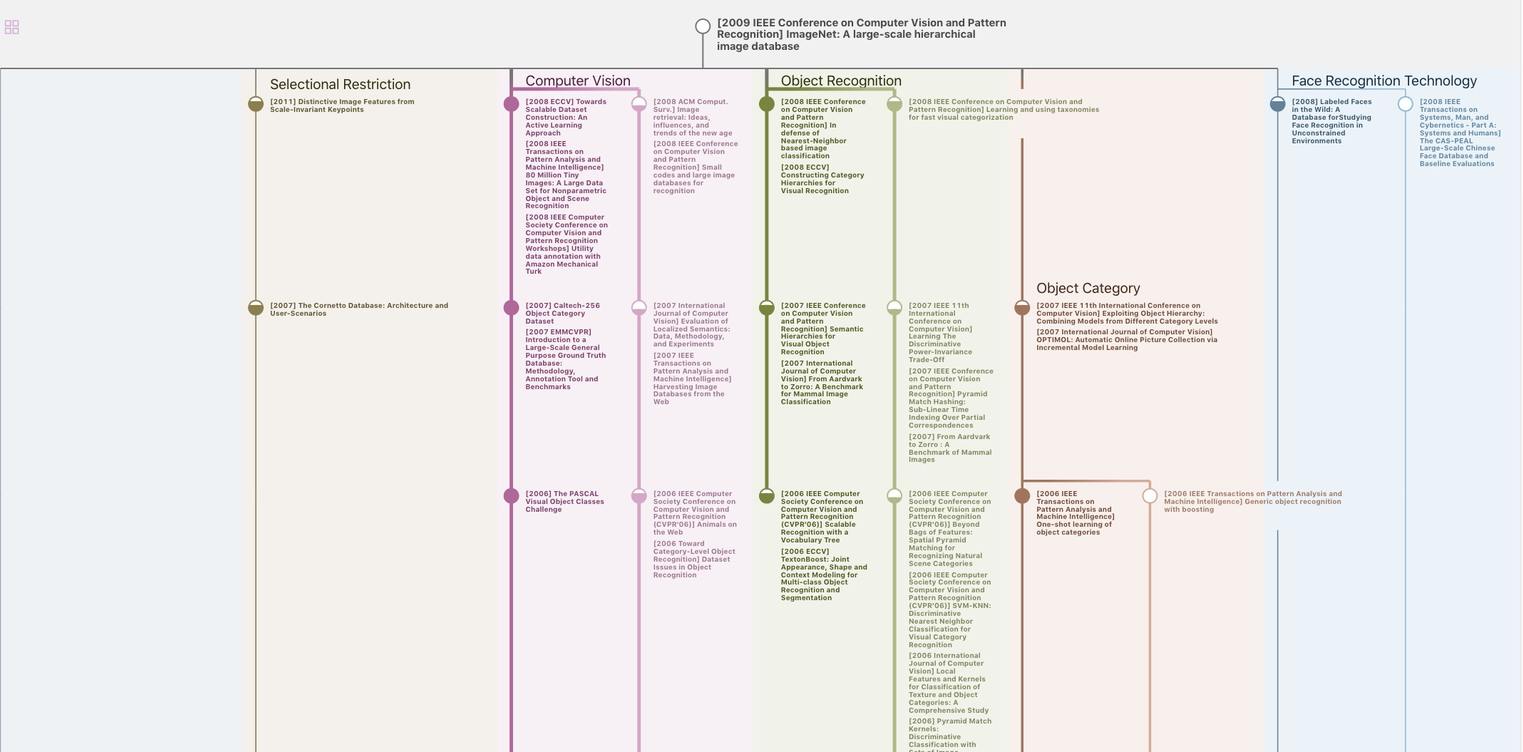
生成溯源树,研究论文发展脉络
Chat Paper
正在生成论文摘要