EFFICIENT ESTIMATION AND COMPUTATION IN GENERALIZED VARYING COEFFICIENT MODELS WITH UNKNOWN LINK AND VARIANCE FUNCTIONS FOR LARGE-SCALE DATA
STATISTICA SINICA(2022)
摘要
Generalized varying-coefficient models have emerged as a powerful tool for modeling nonlinear interactions between covariates and an index variable when the outcome follows a non-normal distribution. The model often stipulates a link function and a variance function, which may not be valid in practice. For example, a large-scale study of loan payment delinquency related to the purchase of expensive smartphones in China, found that parametric functions may not adequately characterize the data and may yield biased results. We propose a generalized varying-coefficient model with unknown link and variance functions. With a massive data set, the simultaneous estimation of these functions and the large number of varying-coefficient functions poses challenges. Thus, we further propose a global kernel estimator and a series of linear approximations that achieves computational and statistical efficiency. The estimators can be expressed explicitly as a linear function of outcomes and are proven to be semiparametrically efficient. Extensive simulations demonstrate the superiority of the method over other competing methods. Lastly, we apply the proposed method to analyze the aforementioned smartphone loan payment data.
更多查看译文
关键词
Asymptotic properties, generalized varying coefficient mod-els, local linear smoothing, quasi-likelihood, semiparametric efficiency
AI 理解论文
溯源树
样例
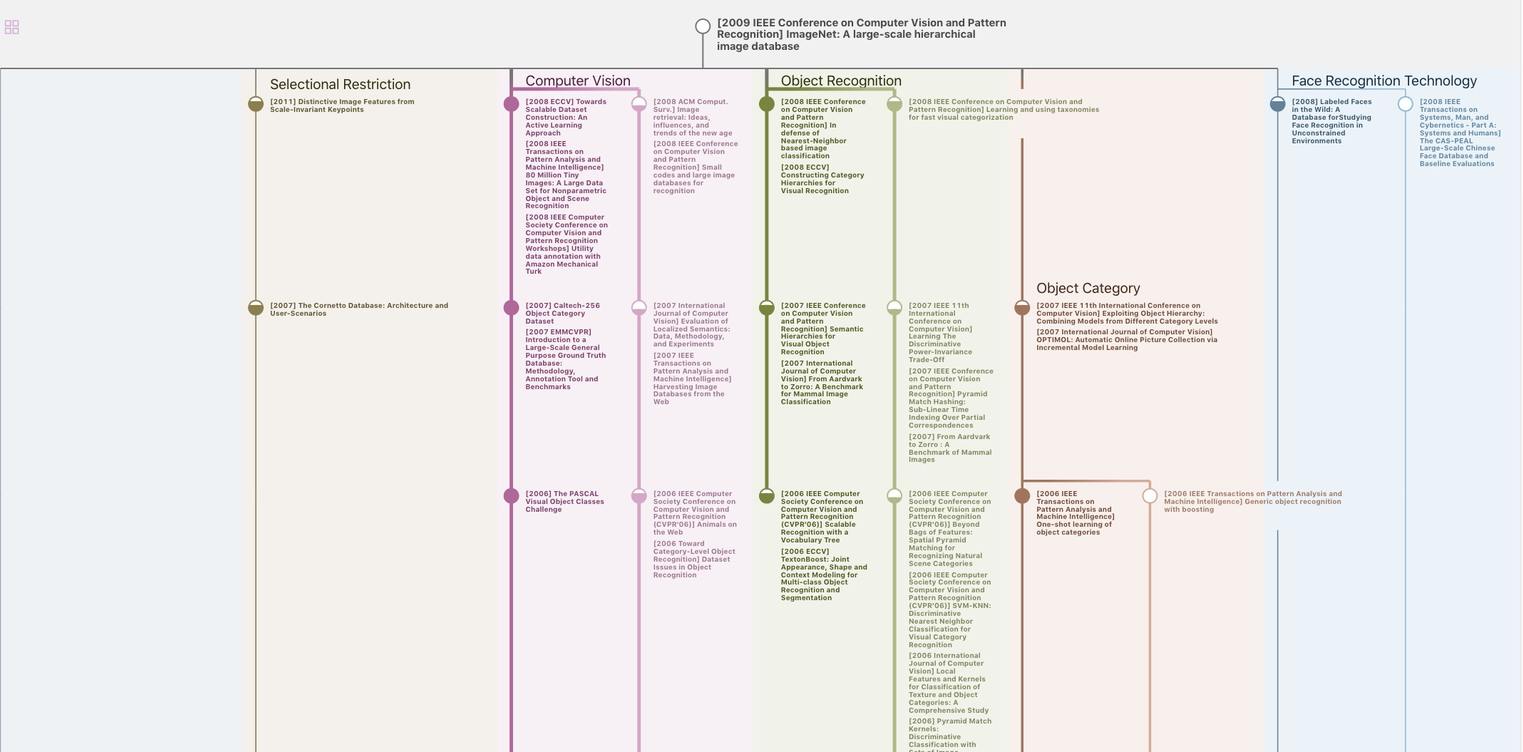
生成溯源树,研究论文发展脉络
Chat Paper
正在生成论文摘要