Deep Reinforcement Learning With Graph Representation for Vehicle Repositioning
IEEE TRANSACTIONS ON INTELLIGENT TRANSPORTATION SYSTEMS(2022)
摘要
On-demand ride services, e.g., taxi companies, ridesourcing/transportation network companies (TNCs), play a vital role in nowadays transportation modes. The relocation/repositioning of idle vehicles is an important operational problem for service providers. With the recent success of deep learning, a large and growing body of literature emerged on learning-based transportation problems, including the relocation problem. In this work, we study the idle vehicle relocation problem to achieve better drivers' profits and customers' satisfaction. In detail, we formulate the learning problem on real-world road networks while recent repositioning works under a deep reinforcement learning scheme formulate the environment as grids. Meanwhile, a graph formulation enables learning with Graph Neural Network (GNN), which shows promising results in recent transportation studies. We empirically show that preserving the road network structure and learning the data representation with GNN contribute to improving the repositioning decision policy. Our simulation experiments are conducted on two real-world datasets, in New York City and Austin, respectively. The results show our formulation's advantages in terms of reductions of order rejection rates and passenger waiting time, and increasing of vehicle occupancy rates and driver revenues.
更多查看译文
关键词
Roads,Task analysis,Vehicles,Public transportation,Reinforcement learning,Law enforcement,Companies,On-demand ride services,vehicle repositioning,graph neural network,road network,Deep Q-Network
AI 理解论文
溯源树
样例
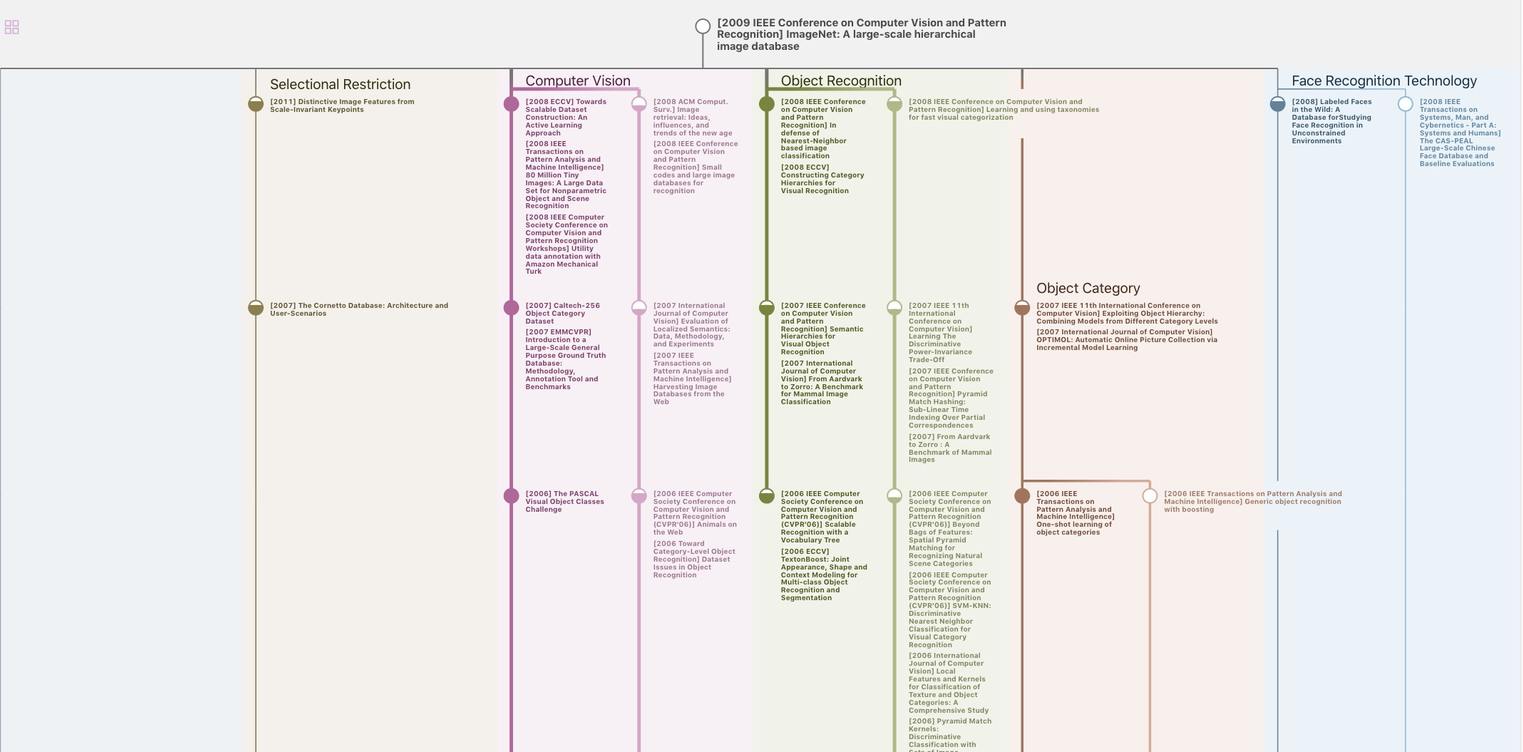
生成溯源树,研究论文发展脉络
Chat Paper
正在生成论文摘要