LE-NAS: Learning-based Ensemble with Neural Architecture Search for 3D Radiotherapy Dose Prediction
semanticscholar(2021)
摘要
Radiation therapy treatment planning is a complex process, as the target dose prescription and normal tissue sparing are conflicting objectives. Automated and accurate dose prediction for radiation therapy planning is in high demand. In this study, we propose a novel learningbased ensemble approach, named LE-NAS, which integrates neural architecture search (NAS) with knowledge distillation for 3D radiotherapy dose prediction. Specifically, the prediction network first exhaustively searches each block from enormous architecture space. Then, multiple architectures are selected with promising performance and diversity. To reduce the inference time, we adopt the teacher-student paradigm by treating the combination of diverse outputs from multiple searched networks as supervisions to guide the student network training. In addition, we apply adversarial learning to optimize the student network to recover the knowledge in teacher networks. To the best of our knowledge, we are the first to investigate the combination of NAS and knowledge distillation. The proposed method has been evaluated on the public OpenKBP dataset, and experimental results demonstrate the effectiveness of our method and its superior performance to the state-of-the-art method.
更多查看译文
AI 理解论文
溯源树
样例
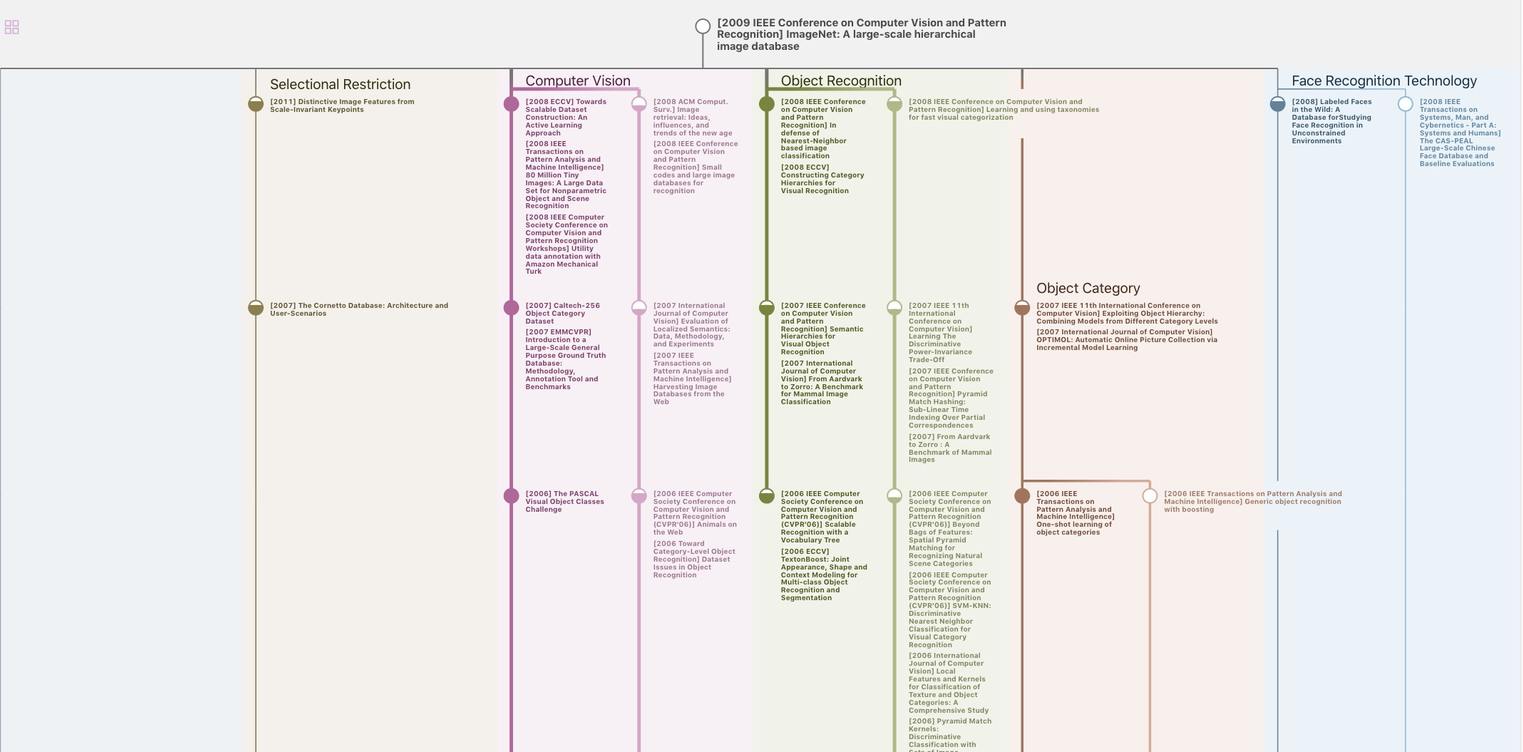
生成溯源树,研究论文发展脉络
Chat Paper
正在生成论文摘要