Mass cytometry and machine learning delineate tumor-defining cells in Sezary syndrome to allow discrimination from benign erythroderma
EUROPEAN JOURNAL OF CANCER(2021)
摘要
Background: In cancer, the lack of early diagnostic biomarkers is a challenge, results in delayed diagnosis, and can seriously affect treatment and prognosis. Therefore, defining accurate methods for early identification of rare malignant cells is of pivotal importance. We explore the potential of artificial intelligence (AI) to uncover tumor-defining cells in Sézary syndrome (SS), a rare type of skin lymphoma. Methods: We established a 37-antibody mass cytometry panel to generate single-cell data from peripheral blood and trained an AI model, called CellCNN, on the datasets. CellCNN is a supervised machine learning algorithm that trains a convolutional neural network with a single layer using labelled single-cell data as inputs. Our study included a discovery cohort of 60 individuals (20 Sézary syndrome patients and as comparators 20 healthy donors and 20 atopic dermatitis patients) and a validation cohort of 33 individuals, containing 11 individuals of each group. Algorithm performance was assessed with area under the curve (AUC), specificity, sensitivity, and F1 score (weighted average of sensitivity and specificity). Findings: We discovered a combination of cell surface markers by mass cytometry to sensitively and specifically identify tumor-defining SS cells in blood and validated our results in a blinded setting with zero false positives and only one false negative prediction (AUC of 0.98, sensitivity of 0.91, specificity of 1.0, F1 score of 0.95). To allow easy implementation into routine diagnostics, we further reduced the number of markers to 15 to be used in standard FACS assays. Diagnostic predictions with those FACS settings still achieved very high sensitivity of 1.0 and specificity of 0.91 (AUC of 0.94, F1 score of 0.95). Furthermore, we could show that our model can even classify blood samples from SS patients at an early stage of initial clinical manifestation, which is currently a grand challenge, with a high accuracy (AUC of 0.93, sensitivity of 1.0, specificity of 0.91, F1 score of 0.95). Interpretation: This study reports the successful validation and performance of an AI-based algorithm to detect rare tumor-defining cells in lymphoma blood samples. Furthermore, our model allows to accurately distinguish tumor samples at initial clinical suspicion and in advanced disease from its benign mimicker. Our findings pave the way for an easy-to-implement and sensitive diagnostic approach to facilitate early diagnosis of Sézary syndrome, and other tumors with blood involvement as well. In cancer, the lack of early diagnostic biomarkers is a challenge, results in delayed diagnosis, and can seriously affect treatment and prognosis. Therefore, defining accurate methods for early identification of rare malignant cells is of pivotal importance. We explore the potential of artificial intelligence (AI) to uncover tumor-defining cells in Sézary syndrome (SS), a rare type of skin lymphoma. We established a 37-antibody mass cytometry panel to generate single-cell data from peripheral blood and trained an AI model, called CellCNN, on the datasets. CellCNN is a supervised machine learning algorithm that trains a convolutional neural network with a single layer using labelled single-cell data as inputs. Our study included a discovery cohort of 60 individuals (20 Sézary syndrome patients and as comparators 20 healthy donors and 20 atopic dermatitis patients) and a validation cohort of 33 individuals, containing 11 individuals of each group. Algorithm performance was assessed with area under the curve (AUC), specificity, sensitivity, and F1 score (weighted average of sensitivity and specificity). We discovered a combination of cell surface markers by mass cytometry to sensitively and specifically identify tumor-defining SS cells in blood and validated our results in a blinded setting with zero false positives and only one false negative prediction (AUC of 0.98, sensitivity of 0.91, specificity of 1.0, F1 score of 0.95). To allow easy implementation into routine diagnostics, we further reduced the number of markers to 15 to be used in standard FACS assays. Diagnostic predictions with those FACS settings still achieved very high sensitivity of 1.0 and specificity of 0.91 (AUC of 0.94, F1 score of 0.95). Furthermore, we could show that our model can even classify blood samples from SS patients at an early stage of initial clinical manifestation, which is currently a grand challenge, with a high accuracy (AUC of 0.93, sensitivity of 1.0, specificity of 0.91, F1 score of 0.95). This study reports the successful validation and performance of an AI-based algorithm to detect rare tumor-defining cells in lymphoma blood samples. Furthermore, our model allows to accurately distinguish tumor samples at initial clinical suspicion and in advanced disease from its benign mimicker. Our findings pave the way for an easy-to-implement and sensitive diagnostic approach to facilitate early diagnosis of Sézary syndrome, and other tumors with blood involvement as well.
更多查看译文
关键词
Sezary syndrome,diagnostic biomarkers,mass cytometry,artificial intelligence
AI 理解论文
溯源树
样例
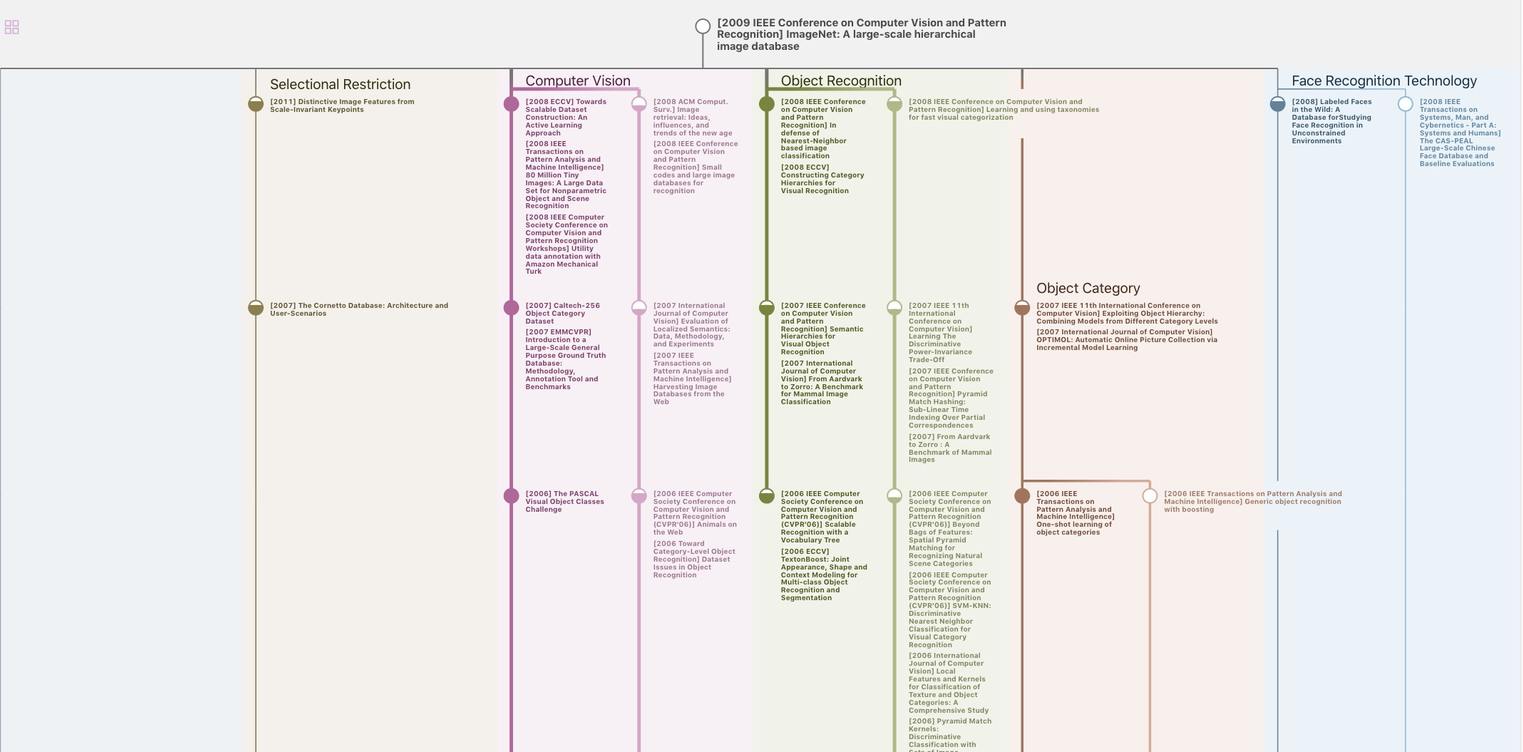
生成溯源树,研究论文发展脉络
Chat Paper
正在生成论文摘要