Mitigating Bias in Facial Recognition with FairGAN
semanticscholar(2020)
摘要
Algorithmic bias has long been a well established phenomenon in computer vision. Previous work by Buolamwini and Gebru has shown that numerous popular facial recognition algorithms that perform gender classification demonstrate performance gaps among individuals of different races [1]. Building off of this work, we seek to neutralize these biases using a data preprocessing approach rooted in Generative Adversarial Networks. We plan to reproduce the approach described in FairGAN [2] to produce images that are debiased with respect to race. In our experiments, we analyze the generated images qualitatively, and compare gender classifiers trained on the real dataset, and the synthetic dataset produced by FairGAN, respectively. We hope to show empirically that the synthetic dataset greatly reduces racial bias in a downstream gender classification task by reducing the performance gap for gender classification between light-skinned and dark skinned individuals. Although our preliminary results are largely inconclusive, we cite specific future steps to move this project closer towards its goal.
更多查看译文
AI 理解论文
溯源树
样例
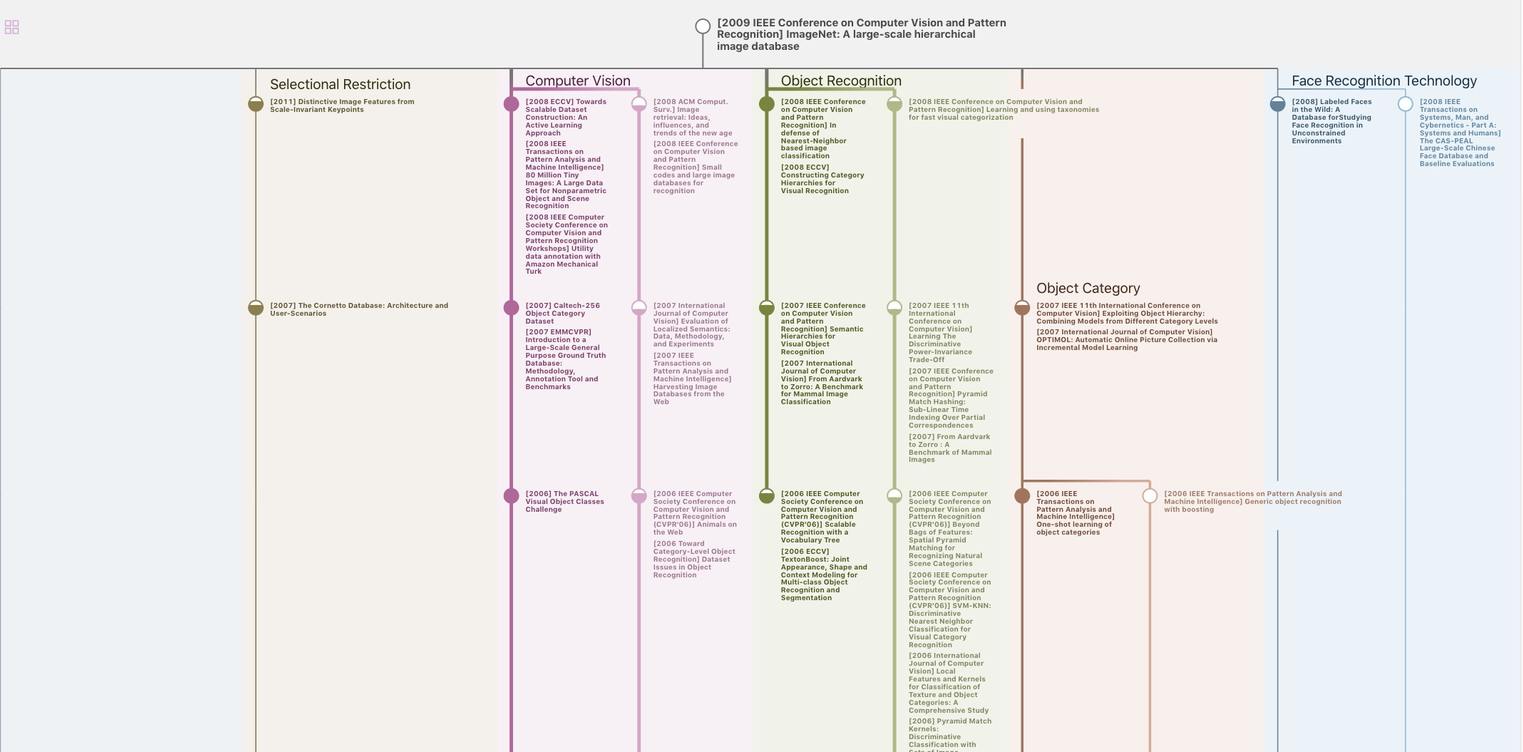
生成溯源树,研究论文发展脉络
Chat Paper
正在生成论文摘要