GATCheck: A Detailed Analysis of Graph Attention Networks
semanticscholar(2020)
摘要
Graph Attention Networks (GATs) are widely used for Representation Learning in Graphs, but there is no proper study highlighting on what tasks GATs perform better than other models and why. In this appraisal paper, we aim to improve our understanding of GATs on a variety of tasks, including link prediction, multi-class node classification, and pairwise node classification on benchmark datasets. We also perform ablation studies on the various hyperparameters of GATs and try to reason about the importance of each of these in node classification and link prediction tasks. Our study offers insights into the effectiveness of GATs as compared to other techniques, and we make our code1 public so as to facilitate future exploration. ACM Reference Format: Lovish Madaan and Siddhant Arora. 2020. GATCheck: A Detailed Analysis of Graph Attention Networks. In Proceedings of MLG’20. ACM, New York, NY, USA, 7 pages. https://doi.org/10.1145/nnnnnnn.nnnnnnn
更多查看译文
AI 理解论文
溯源树
样例
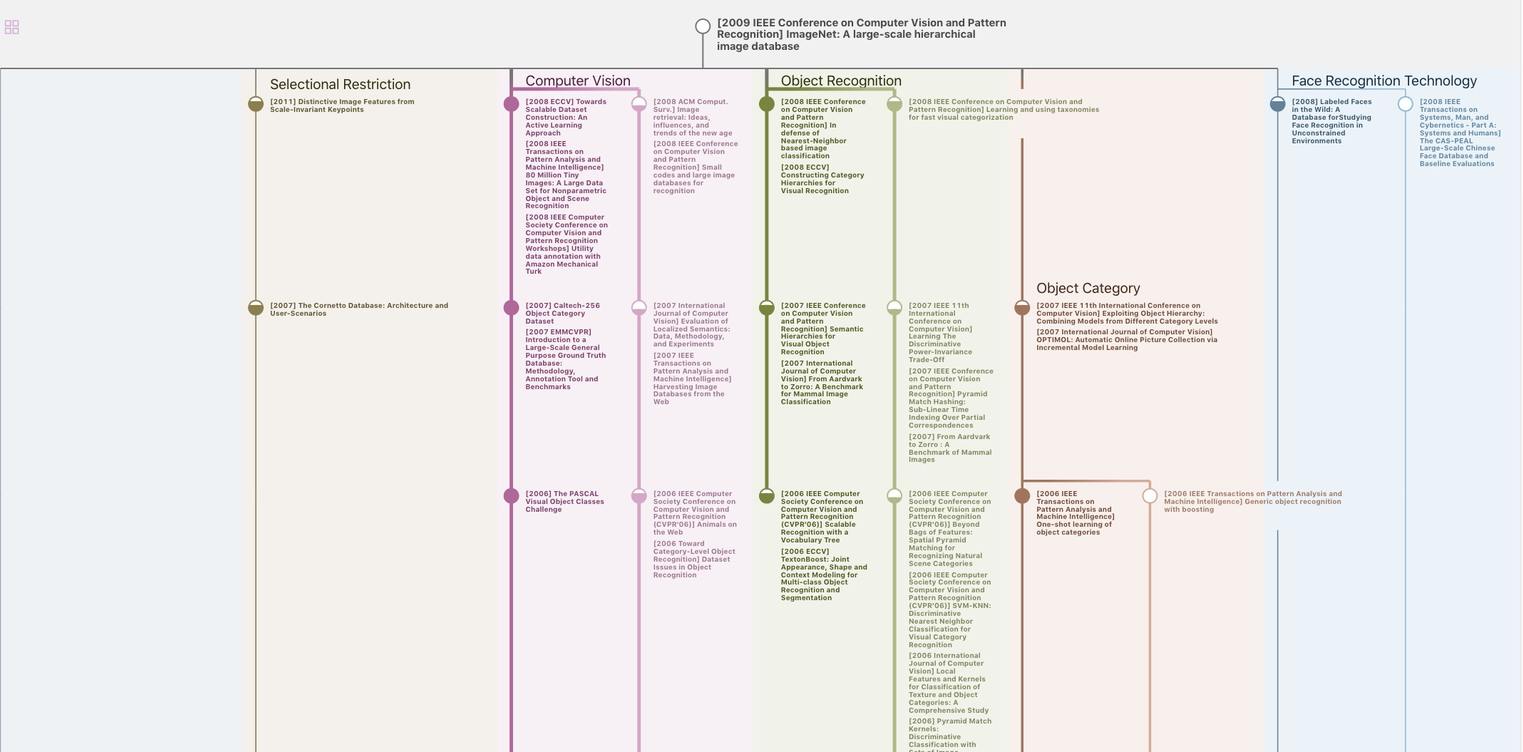
生成溯源树,研究论文发展脉络
Chat Paper
正在生成论文摘要