Separating features from functionality: A computational analysis of vaccination apps (Preprint)
semanticscholar(2021)
摘要
BACKGROUND
Vaccines and immunizations are among the greatest public health accomplishments in disease prevention. Vaccinations not only protect the vaccinated individual but also others in the community. However, inadequate access to healthcare, a fragmented vaccine delivery system, vaccine hesitancy, and lack of vaccine literacy are some of the barriers for vaccination delivery faced by medical professionals and public health agencies. With information technology (IT) at the forefront of delivering quality healthcare, emerging vaccine mHealth technology can positively impact vaccination and immunization practice and benefit individuals, families, and the community. Smartphone apps focused on vaccination and immunizations have proliferated in the digital healthcare market, though their functionality, features, user reviews and limitations have not been comprehensively studied or evaluated.
OBJECTIVE
This study aims to evaluate available vaccine apps on their functionality and features through a rigorous and systematic review with content analysis. Furthermore, we seek to identify potential limitations and drawbacks in the app's design, readability, and information exchange ability. Lastly, we propose recommendations and innovations that can be incorporated in current and future vaccine apps for improved user experience and user acceptance.
METHODS
Vaccine related apps from Android and iOS platforms were systematically searched and selected from the period between January to December 2019. A total of 119 apps were included in this review with 42 features evaluated according to the codebook guidelines. The apps selected were a mix of apps used in the United States and some used internationally, though Homebrew and Sideload apps were excluded. A comprehensive code book was developed to conduct content analysis on app functionalities and app features. We used principal component analysis (PCA) to reduce the dimensionality of the data. Further, cluster analysis was used with unsupervised machine learning to determine patterns within the data to group the apps based on pre-selected features.
RESULTS
The results indicate that readability (quality of being easy to read and understand) of the apps were highly correlated features. When examining the apps star rating score, iOS apps had an average star rating of 0.83 per Mobile App Rating Scale (MARS) as compared to 2.63 for Android. Both the iOS and Android based apps had the privacy statements available with an average of 824.91 words. Cluster one of the K-means analysis contained 27 apps; they were shown to have the most expert involvement, personalized recommendations, and readability among all five clusters.
CONCLUSIONS
The study concluded that most of the apps evaluated were well received by end users. Privacy and security concerns around collection, storage and sharing of health data were addressed. Collaboration with health providers and public health officials during design and development can improve the overall functionality of the apps.
更多查看译文
AI 理解论文
溯源树
样例
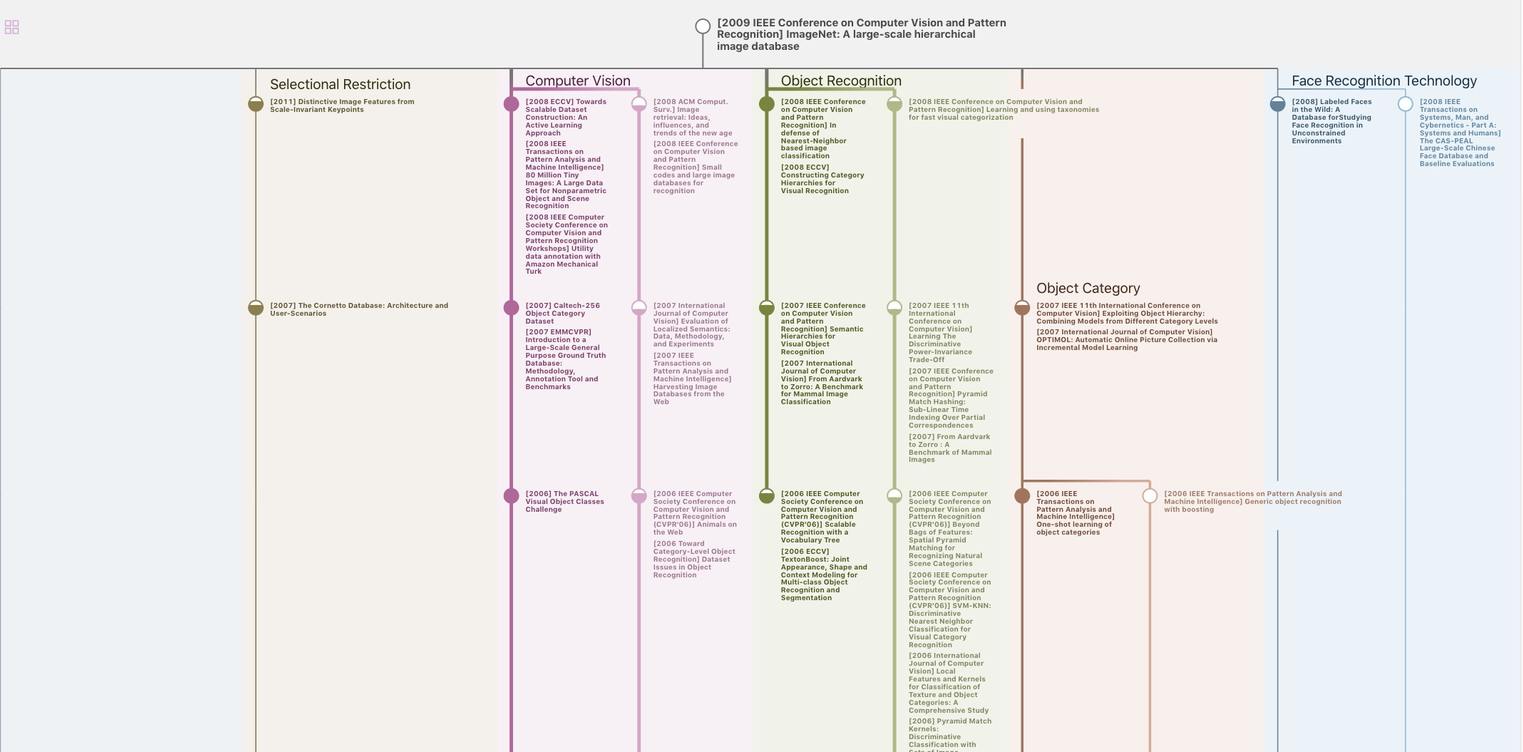
生成溯源树,研究论文发展脉络
Chat Paper
正在生成论文摘要