Machine Learning Enables Accurate and Rapid Prediction of Active Molecules Against Breast Cancer Cells
bioRxiv (Cold Spring Harbor Laboratory)(2021)
摘要
Breast cancer (BC) has surpassed lung cancer as the most frequently occurring cancer, and it is the leading cause of cancer-related death in women. Therefore, there is an urgent need to discover or design new drug candidates for BC treatment. In this study, we first collected a series of structurally diverse datasets consisting of 33,757 active and 21,152 inactive compounds for 13 breast cancer cell lines and one normal breast cell line commonly used in in vitro antiproliferative assays. Predictive models were then developed using five conventional machine learning algorithms, including naive Bayesian, support vector machine, k-Nearest Neighbors, random forest, and extreme gradient boosting, as well as five deep learning algorithms, including deep neural networks, graph convolutional networks, graph attention network, message passing neural networks, and Attentive FP. A total of 476 single models and 112 fusion models were constructed based on three types of molecular representations including molecular descriptors, fingerprints, and graphs. The evaluation results demonstrate that the best model for each BC cell subtype can achieve high predictive accuracy for the test sets with AUC values of 0.689-0.993. Moreover, important structural fragments related to BC cell inhibition were identified and interpreted. To facilitate the use of the model, an online webserver called ChemBC () and its local version software () were developed to predict whether compounds have potential inhibitory activity against BC cells.
更多查看译文
关键词
breast cancer, machine learning, graph neural networks, molecular fingerprints, structural fragments
AI 理解论文
溯源树
样例
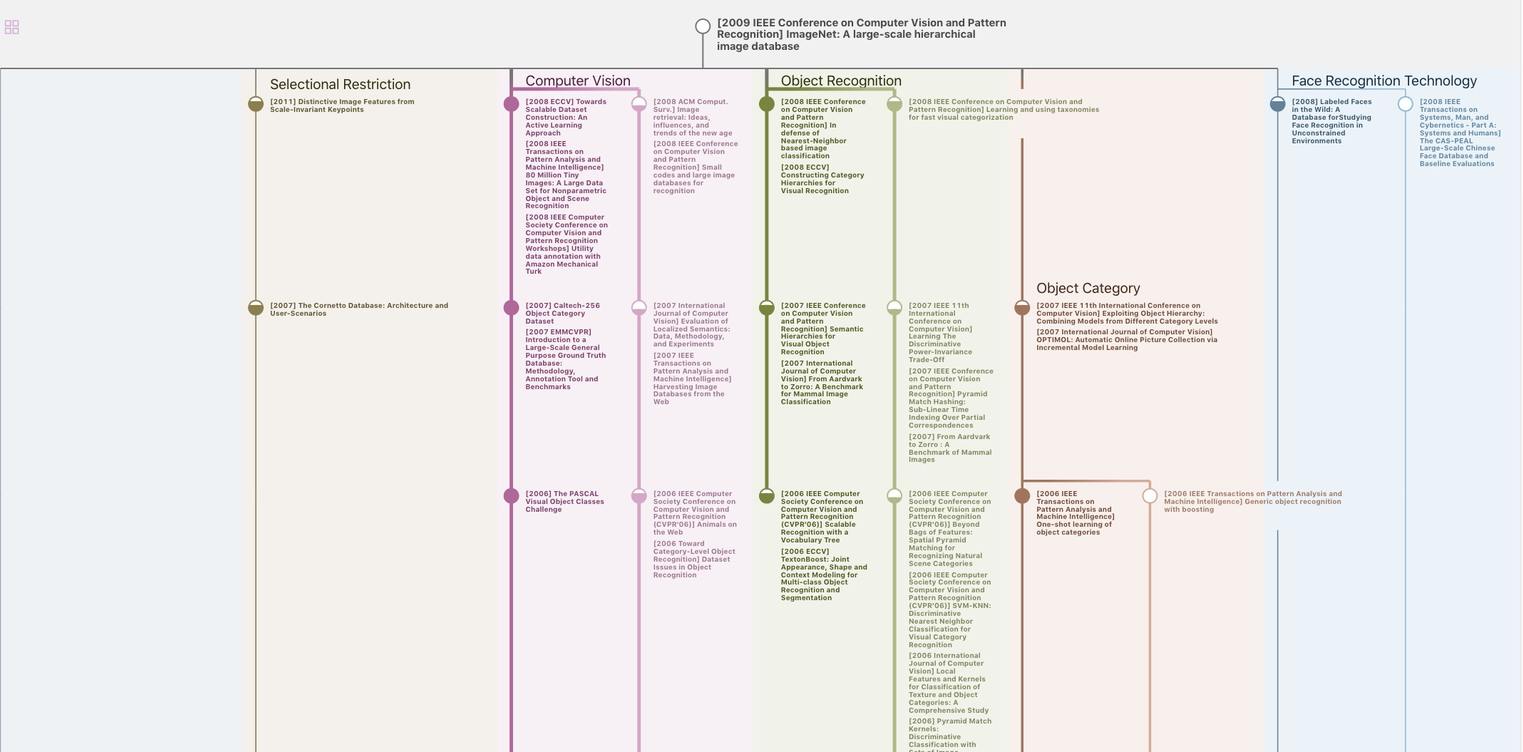
生成溯源树,研究论文发展脉络
Chat Paper
正在生成论文摘要