Lessons learnt from the use of compartmental models over the COVID-19 induced lockdown in France
medRxiv(2021)
摘要
Background: Compartmental models may help deciding on public health interventions. They were used during the first French COVID-19 lockdown to estimate the reproduction numbers and predict the number of hospital beds required. This study aimed to assess the ability of similar compartmental models to reflect equivalent epidemic dynamics. Methods: The study considered three compartmental models independently designed to describe the COVID-19 outbreak in France. These models were scrutinized and their compartments and parameters expressed in a common framework. The parameters were set alike in the three models according to values taken from the literature. The models were calibrated using a common maximum likelihood function and the same hospitalization data taken from two official public databases. The calibration procedure was repeated over three different periods to compare model abilities to (1) fit over the whole lockdown; (2) predict the course of the epidemic during the lockdown; and, (3) provide a set of profiles to forecast the hospitalization prevalence after the lockdown. The study considered national and regional coverages. Results: The three models were all flexible enough to match hospitalization data during the lockdown, but the numbers of cases in the other compartments differed. The three models failed to predict reliably the number of hospitalizations after the fitting periods at national and regional levels. At the national scale, a refined calibration led to epidemic course profiles that reflected hospitalizations dynamics and corresponded to reproduction numbers coherent with official and literature estimates. This result could not be consistently obtained at the regional scale. Conclusion: Not all predictions were consistent between models. Even over the period used for calibration by fitting to hospitalization cases, important differences remained regarding the prevalence in the other compartments. Prevalence data are needed to further constrain the calibration and perform selection between still divergent models. This underlines strongly the need for repeated prevalence studies on representative samples, stratified by age and regions, which would undergo virological or serological tests.
更多查看译文
AI 理解论文
溯源树
样例
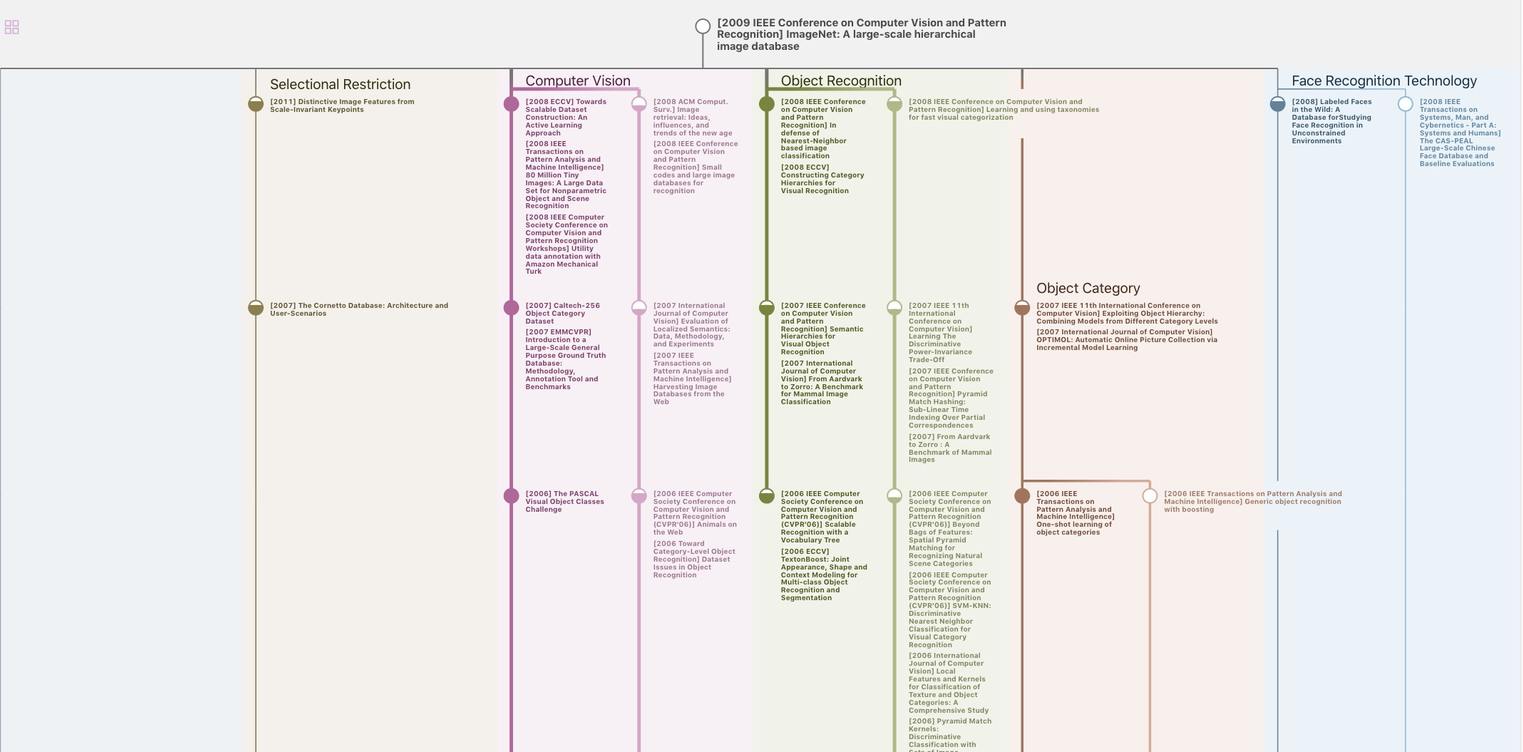
生成溯源树,研究论文发展脉络
Chat Paper
正在生成论文摘要