Cross-Modal Distillation to Improve MRI-Based Brain Tumor Segmentation With Missing MRI Sequences
IEEE Transactions on Biomedical Engineering(2022)
摘要
Convolutional neural networks (CNNs) for brain tumor segmentation are generally developed using complete sets of magnetic resonance imaging (MRI) sequences for both training and inference. As such, these algorithms are not trained for realistic, clinical scenarios where parts of the MRI sequences which were used for training, are missing during inference. To increase clinical applicability, we proposed a cross-modal distillation approach to leverage the availability of multi-sequence MRI data for training and generate an enriched CNN model which uses only single-sequence MRI data for inference but outperforms a single-sequence CNN model. We assessed the performance of the proposed method for whole tumor and tumor core segmentation with multi-sequence MRI data available for training but only
$T_{1}$
-weighted (
$T_{\text{1}w}$
) sequence data available for inference, using BraTS 2018, and in-house datasets. Results showed that cross-modal distillation significantly improved the Dice score for both whole tumor and tumor core segmentation when only
$T_{\text{1}w}$
sequence data were available for inference. For the evaluation using the in-house dataset, cross-modal distillation achieved an average Dice score of 79.04% and 69.39% for whole tumor and tumor core segmentation, respectively, while a single-sequence U-Net model using
$T_{\text{1}w}$
sequence data for both training and inference achieved an average Dice score of 73.60% and 62.62%, respectively. These findings confirmed cross-modal distillation as an effective method to increase the potential of single-sequence CNN models such that segmentation performance is less compromised by missing MRI sequences or having only one MRI sequence available for segmentation.
更多查看译文
关键词
Brain Neoplasms,Humans,Image Processing, Computer-Assisted,Magnetic Resonance Imaging,Neural Networks, Computer,Neuroimaging
AI 理解论文
溯源树
样例
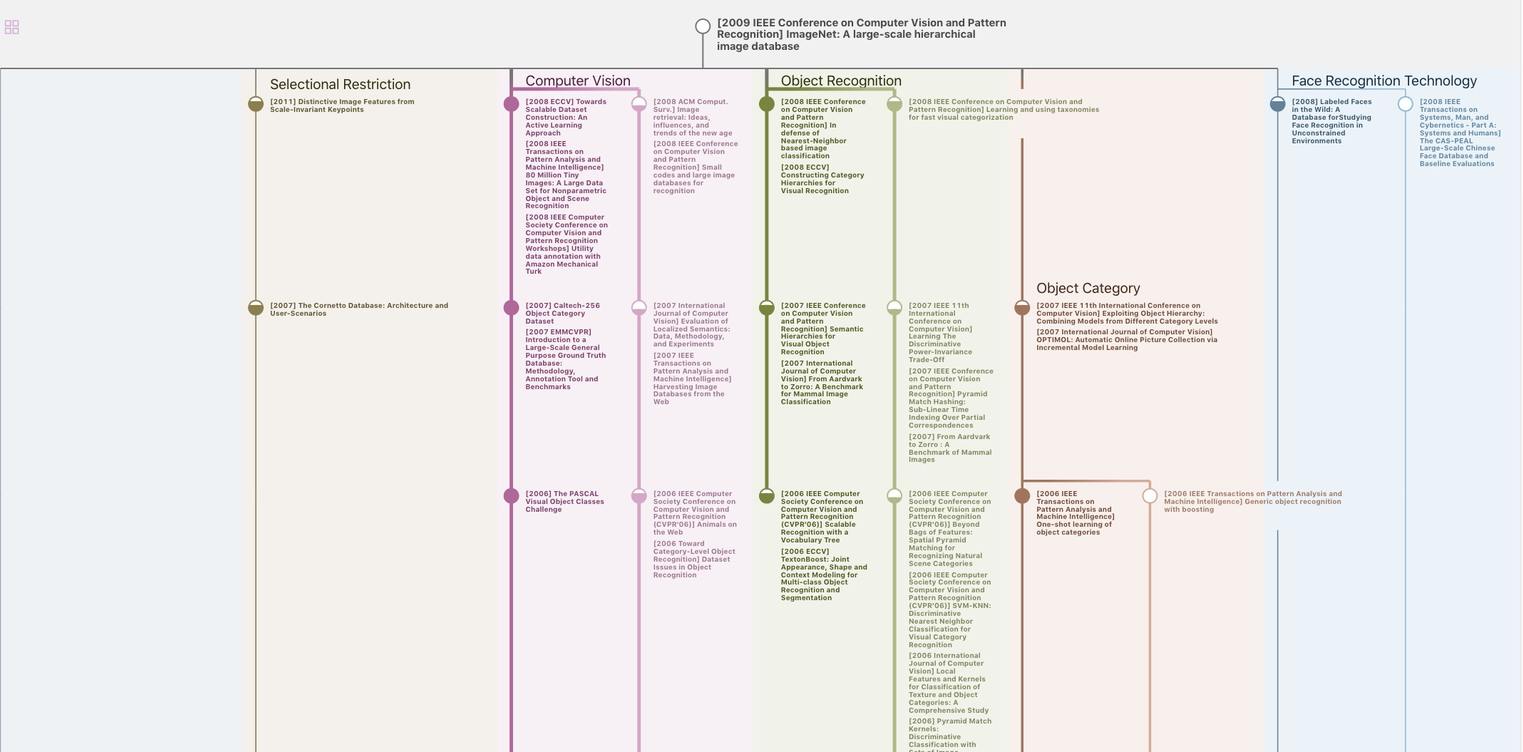
生成溯源树,研究论文发展脉络
Chat Paper
正在生成论文摘要