Dynamic Learning and Decision-Making by the Aid of Basis Weight Frontiers1
semanticscholar(2021)
摘要
A new methodology is presented to solve an important model of dynamic decision-making with a continuous unknown parameter (or state). The methodology centers on the concepts of “continuation-value function” (which gives the expected value-to-go as a function of the parameter under a feasible policy) and “efficient frontier” of such functions in each period. When the model primitives can be described through a family of basis functions, e.g. polynomials, a continuationvalue function retains that property and can be fully represented by a basis weight vector. The efficient frontiers of the weight vectors can be constructed through backward induction, which leads to an essential reduction of problem complexity and enables an exact solution for small-sized problems. A set of approximation methods based on the new methodology are developed to tackle larger problems. The methodology is also extended to the multi-dimensional (multi-parameter) setting, which features the problem of contextual multi-armed bandits with linear expected rewards. Our approximation algorithm for that problem outperforms three benchmark algorithms in challenging learning environments with many actions and short horizons.
更多查看译文
AI 理解论文
溯源树
样例
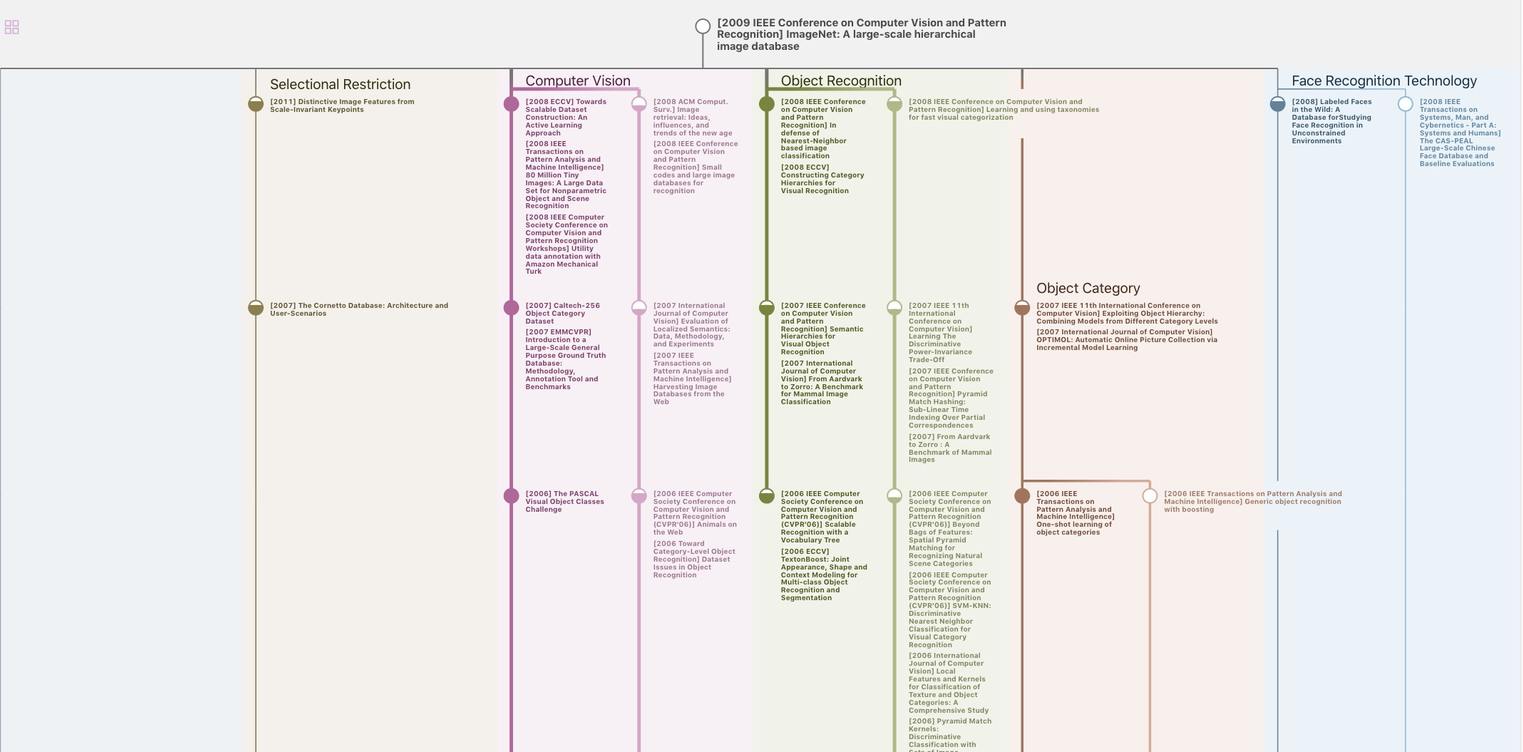
生成溯源树,研究论文发展脉络
Chat Paper
正在生成论文摘要