The Automatic Classification of Pyriproxyfen-Affected Mosquito Ovaries
INSECTS(2021)
摘要
Simple Summary With resistance to the pyrethroid family of insecticides increasing, it is more important than ever that tools are available to measure the efficacy of alternatives. Pyriproxyfen (PPF) is an alternative insecticide whose mode of action sterilises adult mosquitoes. Consequently, the efficacy of PPF-based tools can be measured through visual examination of egg development by trained experts. This, however, can be a time-consuming process for which the required expertise can be difficult to train and is not available in many contexts. As such, we propose that an objective machine learning program, which can automatically classify the fertility status of adult mosquito ovaries via a colour image, be developed to improve the speed, accuracy, and consistency of assessment. This study shows that a convolutional neural network, built in Python's TensorFlow library, can quickly classify images of dissected ovaries into either 'fertile' or 'infertile' with a high accuracy rate. Such an application would be a practical and accessible tool available to all researchers studying the efficacy of PPF or other insecticides with a similar mode of action. Pyriproxyfen (PPF) may become an alternative insecticide for areas where pyrethroid-resistant vectors are prevalent. The efficacy of PPF can be assessed through the dissection and assessment of vector ovaries. However, this reliance on expertise is subject to limitations. We show here that these limitations can be overcome using a convolutional neural network (CNN) to automate the classification of egg development and thus fertility status. Using TensorFlow, a resnet-50 CNN was pretrained with the ImageNet dataset. This CNN architecture was then retrained using a novel dataset of 524 dissected ovary images from An. gambiae s.l. An. gambiae Akron, and An. funestus s.l., whose fertility status and PPF exposure were known. Data augmentation increased the training set to 6973 images. A test set of 157 images was used to measure accuracy. This CNN model achieved an accuracy score of 94%, and application took a mean time of 38.5 s. Such a CNN can achieve an acceptable level of precision in a quick, robust format and can be distributed in a practical, accessible, and free manner. Furthermore, this approach is useful for measuring the efficacy and durability of PPF treated bednets, and it is applicable to any PPF-treated tool or similarly acting insecticide.
更多查看译文
关键词
Anopheles mosquito, fertility, ovary development, pyriproxyfen (PPF), side-effects, machine learning, image classification, automated identification, convolutional neural network
AI 理解论文
溯源树
样例
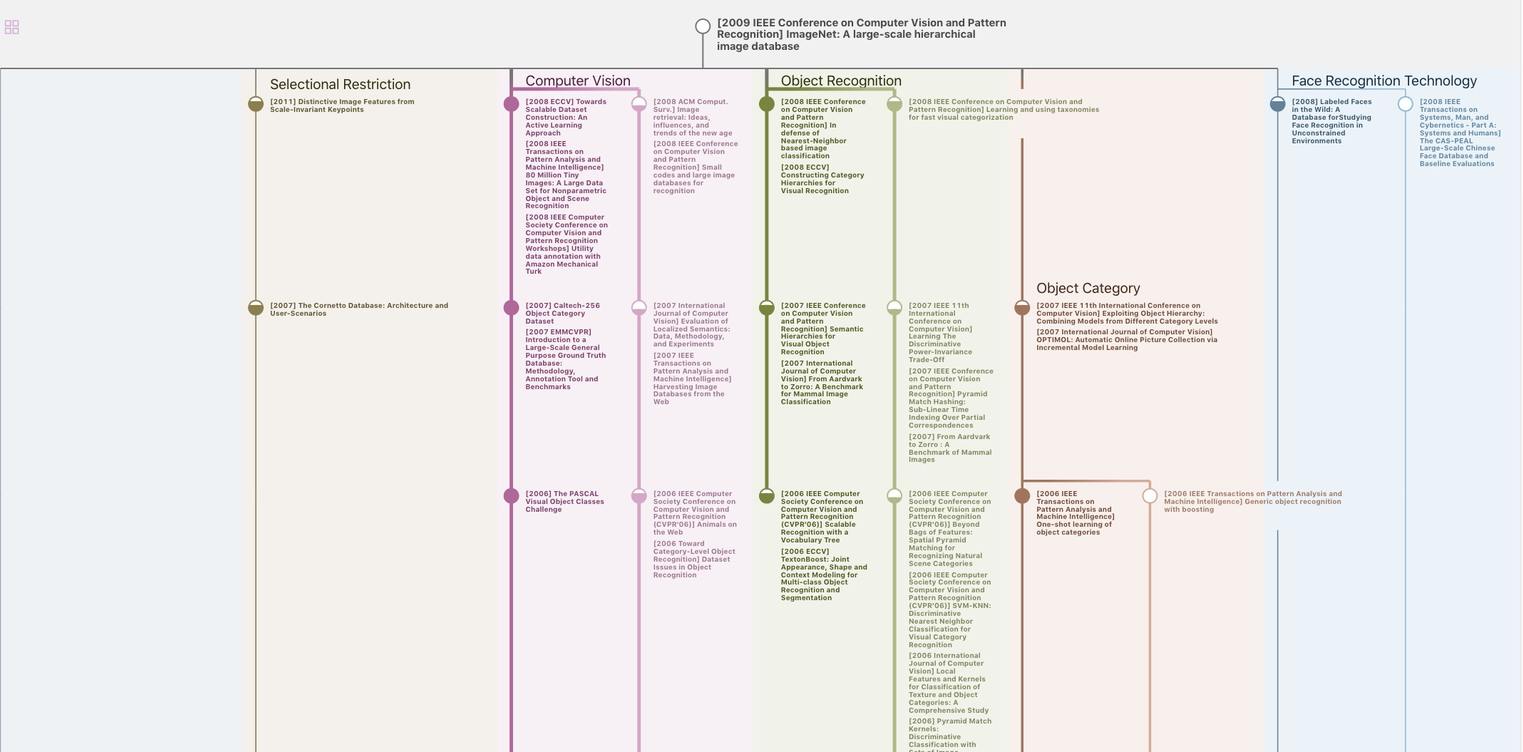
生成溯源树,研究论文发展脉络
Chat Paper
正在生成论文摘要