Automatic detection of respiratory events during sleep from Polysomnography data using Layered Hidden Markov Model
PHYSIOLOGICAL MEASUREMENT(2022)
摘要
Objective. Sleep apnea is a serious respiratory disorder, which is associated with increased risk factors for cardiovascular disease. Many studies in recent years have been focused on automatic detection of sleep apnea from polysomnography (PSG) recordings, however, detection of subtle respiratory events named Respiratory Event Related Arousals (RERAs) that do not meet the criteria for apnea or hypopnea is still challenging. The objective of this study was to develop automatic detection of sleep apnea based on Hidden Markov Models (HMMs) which are probabilistic models with the ability to learn different dynamics of the real time-series such as clinical recordings. Approach. In this study, a hierarchy of HMMs named Layered HMM was presented to detect respiratory events from PSG recordings. The recordings of 210 PSGs from Massachusetts General Hospital's database were used for this study. To develop detection algorithms, extracted feature signals from airflow, movements over the chest and abdomen, and oxygen saturation in blood (SaO(2)) were chosen as observations. The respiratory disturbance index (RDI) was estimated as the number of apneas, hypopneas, and RERAs per hour of sleep. Main results. The best F1 score of the event by event detection algorithm was between 0.22 +/- 0.16 and 0.70 +/- 0.08 for different groups of sleep apnea severity. There was a strong correlation between the estimated and the PSG-derived RDI (R (2) = 0.91, p < 0.0001). The best recall of RERA detection was achieved 0.45 +/- 0.27. Significance. The results showed that the layered structure can improve the performance of the detection of respiratory events during sleep.
更多查看译文
关键词
Sleep apnea, Respiratory Event Related Arousals, Respiratory Disturbance Index, Hidden Markov Model, Event detection, Polysomnography
AI 理解论文
溯源树
样例
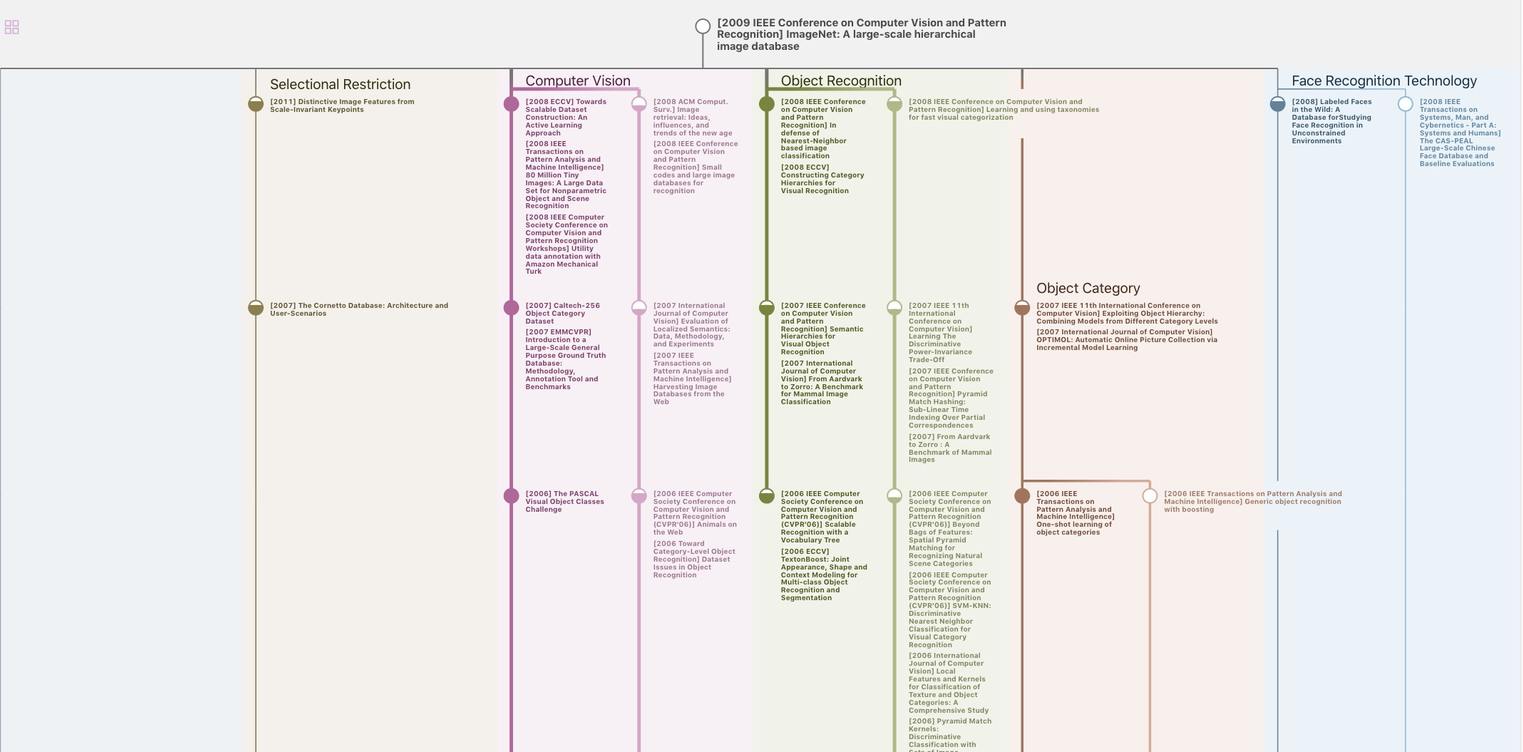
生成溯源树,研究论文发展脉络
Chat Paper
正在生成论文摘要