Cross Modal Retrieval with Querybank Normalisation
IEEE Conference on Computer Vision and Pattern Recognition(2022)
摘要
Profiting from large-scale training datasets, advances in neural architecture design and efficient inference, joint embeddings have become the dominant approach for tackling cross-modal retrieval. In this work we first show that, despite their effectiveness, state-of-the-art joint embeddings suffer significantly from the longstanding “hubness problem” in which a small number of gallery embeddings form the nearest neighbours of many queries. Drawing inspiration from the NLP literature, we formulate a simple but effective framework called Querybank Normalisation (QB-NORM) that re-normalises query similarities to account for hubs in the embedding space. QB-NORM improves retrieval performance without requiring retraining. Differently from prior work, we show that QB-NORM works effectively without concurrent access to any test set queries. Within the QB-NORM framework, we also propose a novel similarity normalisation method, the Dynamic Inverted Softmax, that is significantly more robust than existing approaches. We showcase QB-NORM across a range of cross modal retrieval models and benchmarks where it consistently enhances strong baselines beyond the state of the art. Code is available at https://vladbogo.github.io/QB-Norm/.
更多查看译文
关键词
Vision + language, Recognition: detection,categorization,retrieval
AI 理解论文
溯源树
样例
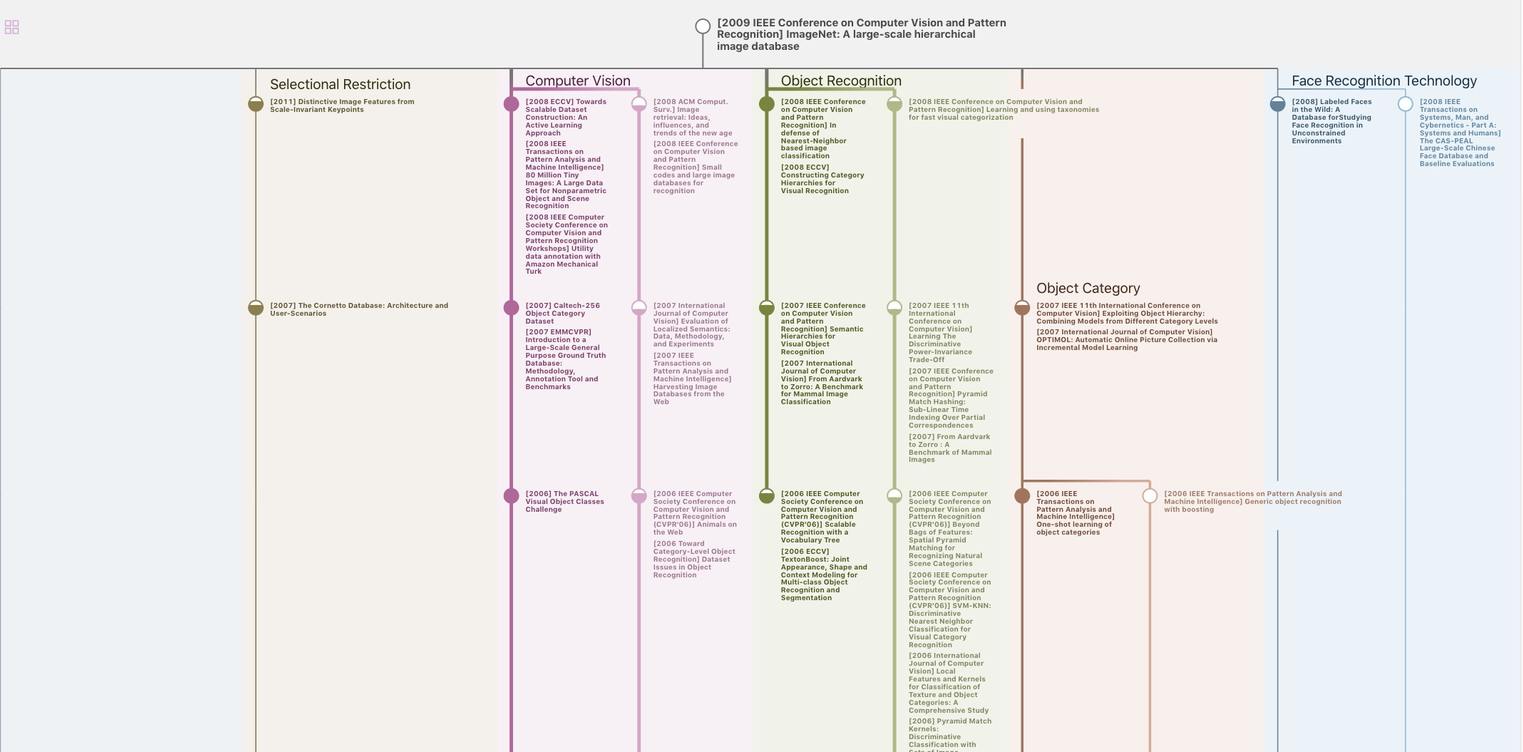
生成溯源树,研究论文发展脉络
Chat Paper
正在生成论文摘要