Group feature selection for enhancing information gain in MRI reconstruction
PHYSICS IN MEDICINE AND BIOLOGY(2022)
摘要
Magnetic resonance imaging (MRI) has revolutionized radiology. As a leading medical imaging modality, MRI not only visualizes the structures inside the body but also produces functional imaging. However, due to the slow imaging speed constrained by magnetic resonance physics, the MRI cost is expensive, and patients may feel not comfortable in a scanner for a long time. Parallel MRI (pMRI) has accelerated the imaging speed through a sub-Nyquist sampling strategy and the missing data are interpolated by the multiple coil data acquired. Kernel learning has been used in pMRI reconstruction to learn the interpolation weights and reconstruct the undersampled data. However, noise and aliasing artifacts still exist in the reconstructed image and a large number of auto-calibration signal lines are needed. To further improve kernel-learning-based MRI reconstruction and accelerate the speed, this paper proposes a group feature selection strategy to improve the learning performance and enhance the reconstruction quality. An explicit kernel mapping is used for selecting a subset of features which contribute most to estimating the missing k-space data. The experimental results show that the learning behaviors can be better predicted and therefore the reconstructed image quality can be improved.
更多查看译文
关键词
parallel MRI reconstruction, group feature selection, information gain, kernel learning
AI 理解论文
溯源树
样例
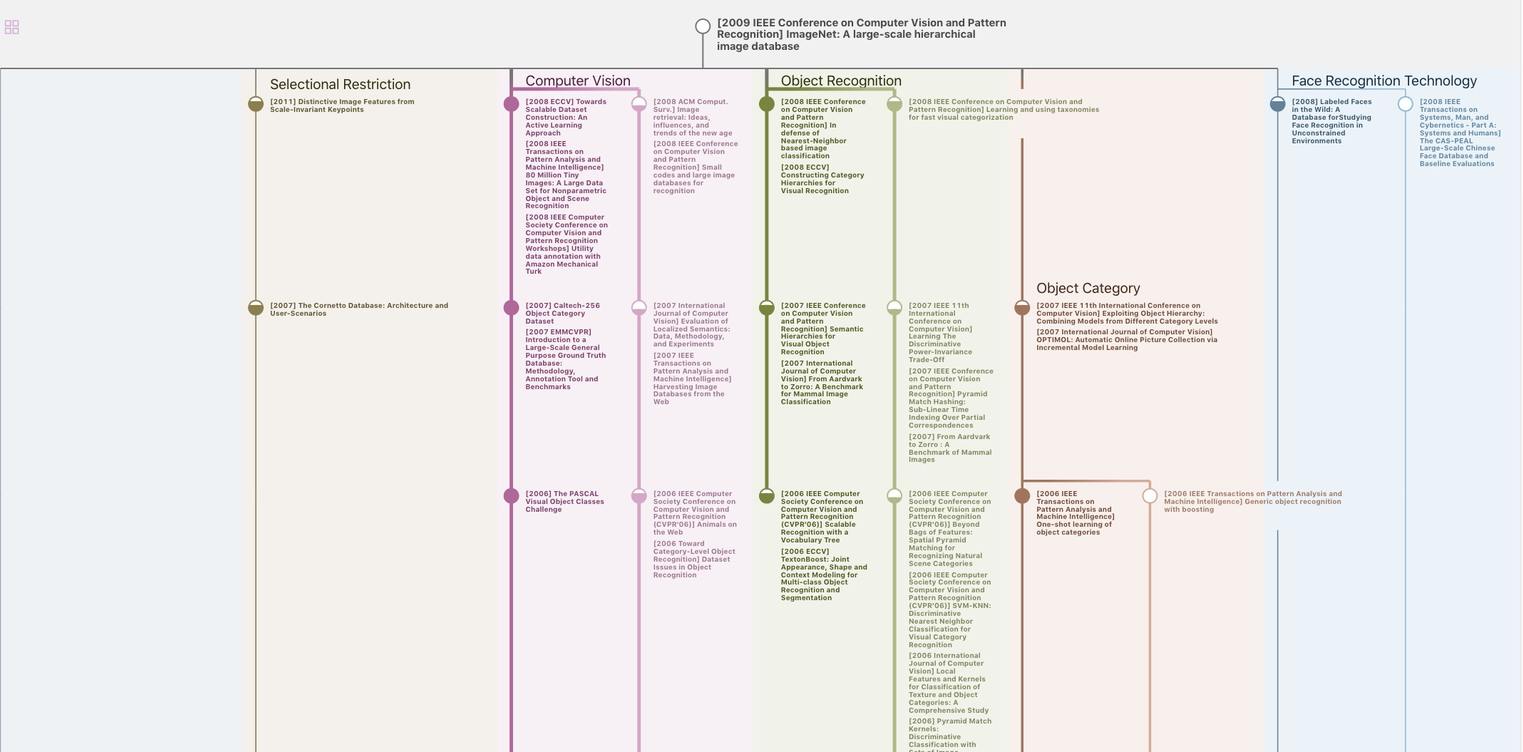
生成溯源树,研究论文发展脉络
Chat Paper
正在生成论文摘要