HEGANLDA: A Computational Model for Predicting Potential Lncrna-Disease Associations Based On Multiple Heterogeneous Networks
IEEE/ACM Transactions on Computational Biology and Bioinformatics(2023)
摘要
Long non-coding RNAs (lncRNAs) play vital regulatory roles in many human complex diseases, however, the number of validated lncRNA-disease associations is notable rare so far. How to predict potential lncRNA-disease associations precisely through computational methods remains challenging. In this study, we proposed a novel method, LDVCHN (LncRNA-Disease Vector Calculation Heterogeneous Networks), and also developed the corresponding model, HEGANLDA (Heterogeneous Embedding Generative Adversarial Networks LncRNA-Disease Association), for predicting potential lncRNA-disease associations. In HEGANLDA, the graph embedding algorithm (HeGAN) was introduced for mapping all nodes in the lncRNA-miRNA-disease heterogeneous network into the low-dimensional vectors which severed as the inputs of LDVCHN. HEGANLDA effectively adopted the XGBoost (eXtreme Gradient Boosting) classifier, which was trained by the low-dimensional vectors, to predict potential lncRNA-disease associations. The 10-fold cross-validation method was utilized to evaluate the performance of our model, our model finally achieved an area under the ROC curve of 0.983. According to the experiment results, HEGANLDA outperformed any one of five current state-of-the-art methods. To further evaluate the effectiveness of HEGANLDA in predicting potential lncRNA-disease associations, both case studies and robustness tests were performed and the results confirmed its effectiveness and robustness. The source code and data of HEGANLDA are available at
https://github.com/HEGANLDA/HEGANLDA
.
更多查看译文
关键词
Graph embedding algorithm,heterogeneous network,lncRNA-disease association prediction,XGBoost classifier
AI 理解论文
溯源树
样例
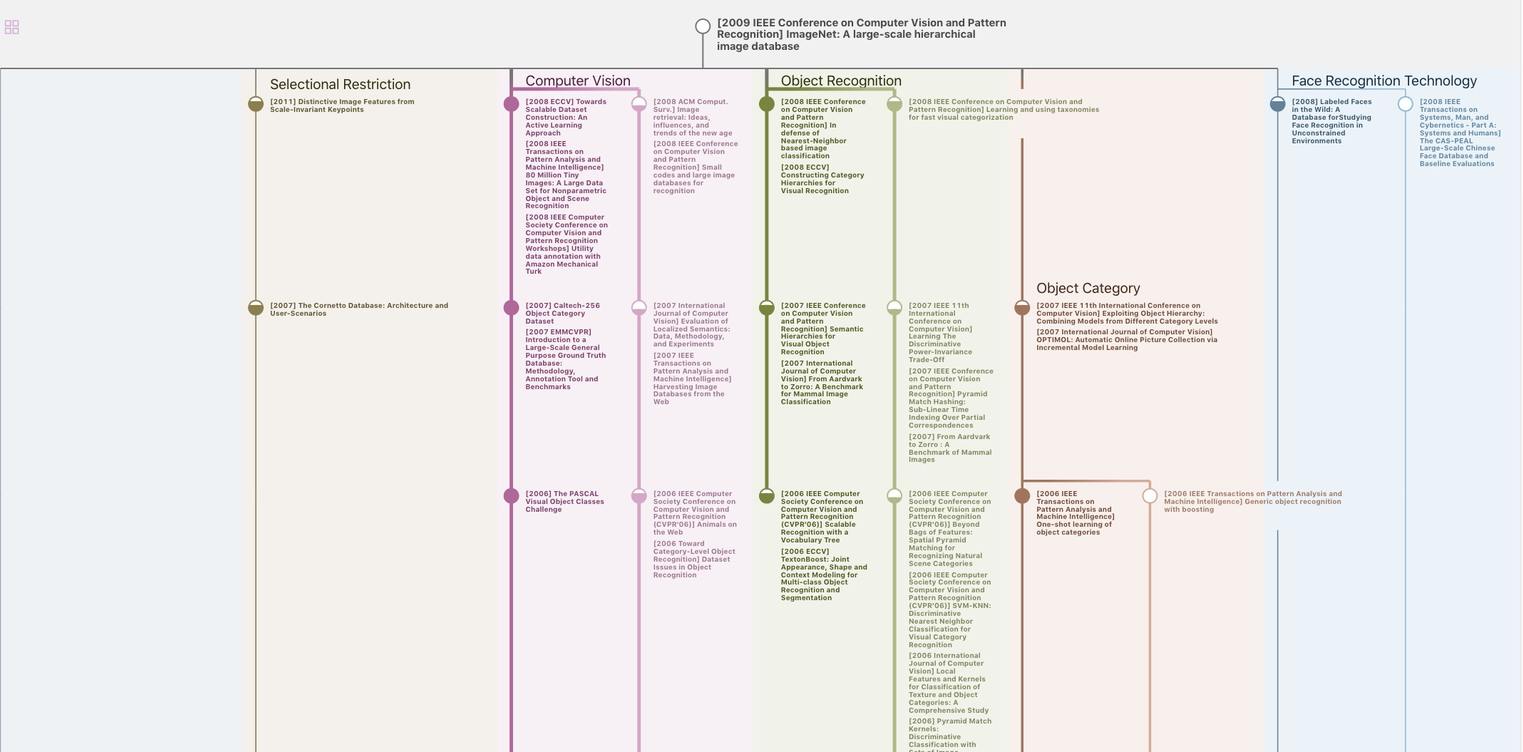
生成溯源树,研究论文发展脉络
Chat Paper
正在生成论文摘要