FedLGA: Towards System-Heterogeneity of Federated Learning via Local Gradient Approximation
arxiv(2021)
摘要
Federated Learning (FL) is a decentralized machine learning architecture,
which leverages a large number of remote devices to learn a joint model with
distributed training data. However, the system-heterogeneity is one major
challenge in a FL network to achieve robust distributed learning performance,
which comes from two aspects: i) device-heterogeneity due to the diverse
computational capacity among devices; ii) data-heterogeneity due to the
non-identically distributed data across the network. Prior studies addressing
the heterogeneous FL issue, e.g., FedProx, lack formalization and it remains an
open problem. This work first formalizes the system-heterogeneous FL problem
and proposes a new algorithm, called FedLGA, to address this problem by
bridging the divergence of local model updates via gradient approximation. To
achieve this, FedLGA provides an alternated Hessian estimation method, which
only requires extra linear complexity on the aggregator. Theoretically, we show
that with a device-heterogeneous ratio ρ, FedLGA achieves convergence
rates on non-i.i.d. distributed FL training data for the non-convex
optimization problems with 𝒪( (1+ρ)/√(ENT) +
1/T) and 𝒪( (1+ρ)√(E)/√(TK)
+ 1/T) for full and partial device participation respectively,
where E is the number of local learning epoch, T is the number of total
communication round, N is the total device number and K is the number of
selected device in one communication round under partially participation
scheme. The results of comprehensive experiments on multiple datasets show that
FedLGA outperforms current FL methods against the system-heterogeneity.
更多查看译文
AI 理解论文
溯源树
样例
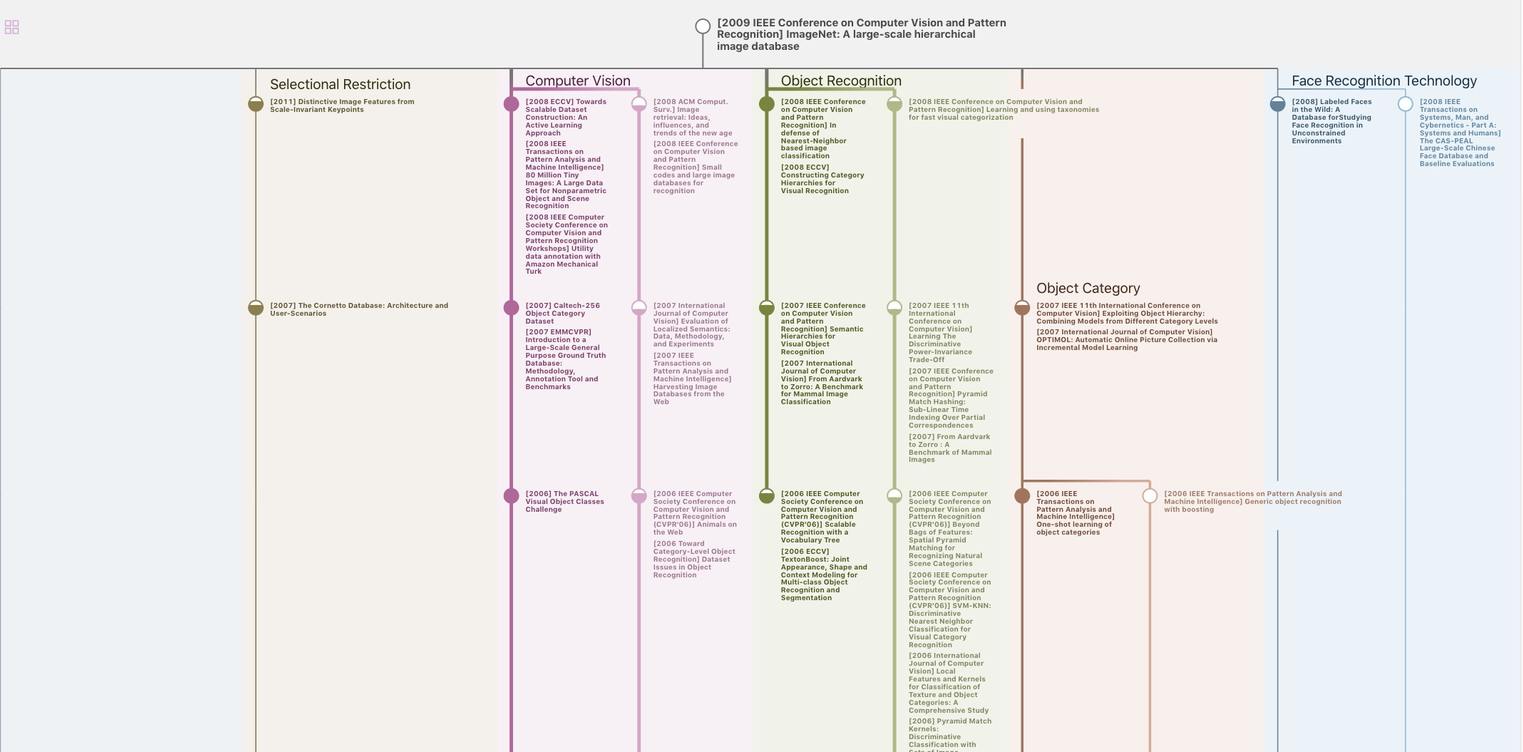
生成溯源树,研究论文发展脉络
Chat Paper
正在生成论文摘要