POD-Galerkin reduced order models and physics-informed neural networks for solving inverse problems for the Navier–Stokes equations
arxiv(2023)
摘要
We present a Reduced Order Model (ROM) which exploits recent developments in Physics Informed Neural Networks (PINNs) for solving inverse problems for the Navier–Stokes equations (NSE). In the proposed approach, the presence of simulated data for the fluid dynamics fields is assumed. A POD-Galerkin ROM is then constructed by applying POD on the snapshots matrices of the fluid fields and performing a Galerkin projection of the NSE (or the modified equations in case of turbulence modeling) onto the POD reduced basis. A POD-Galerkin PINN ROM is then derived by introducing deep neural networks which approximate the reduced outputs with the input being time and/or parameters of the model. The neural networks incorporate the physical equations (the POD-Galerkin reduced equations) into their structure as part of the loss function. Using this approach, the reduced model is able to approximate unknown parameters such as physical constants or the boundary conditions. A demonstration of the applicability of the proposed ROM is illustrated by three cases which are the steady flow around a backward step, the flow around a circular cylinder and the unsteady turbulent flow around a surface mounted cubic obstacle.
更多查看译文
关键词
Proper orthogonal decomposition,Inverse problems,Physics-based machine learning,Navier–Stokes equations
AI 理解论文
溯源树
样例
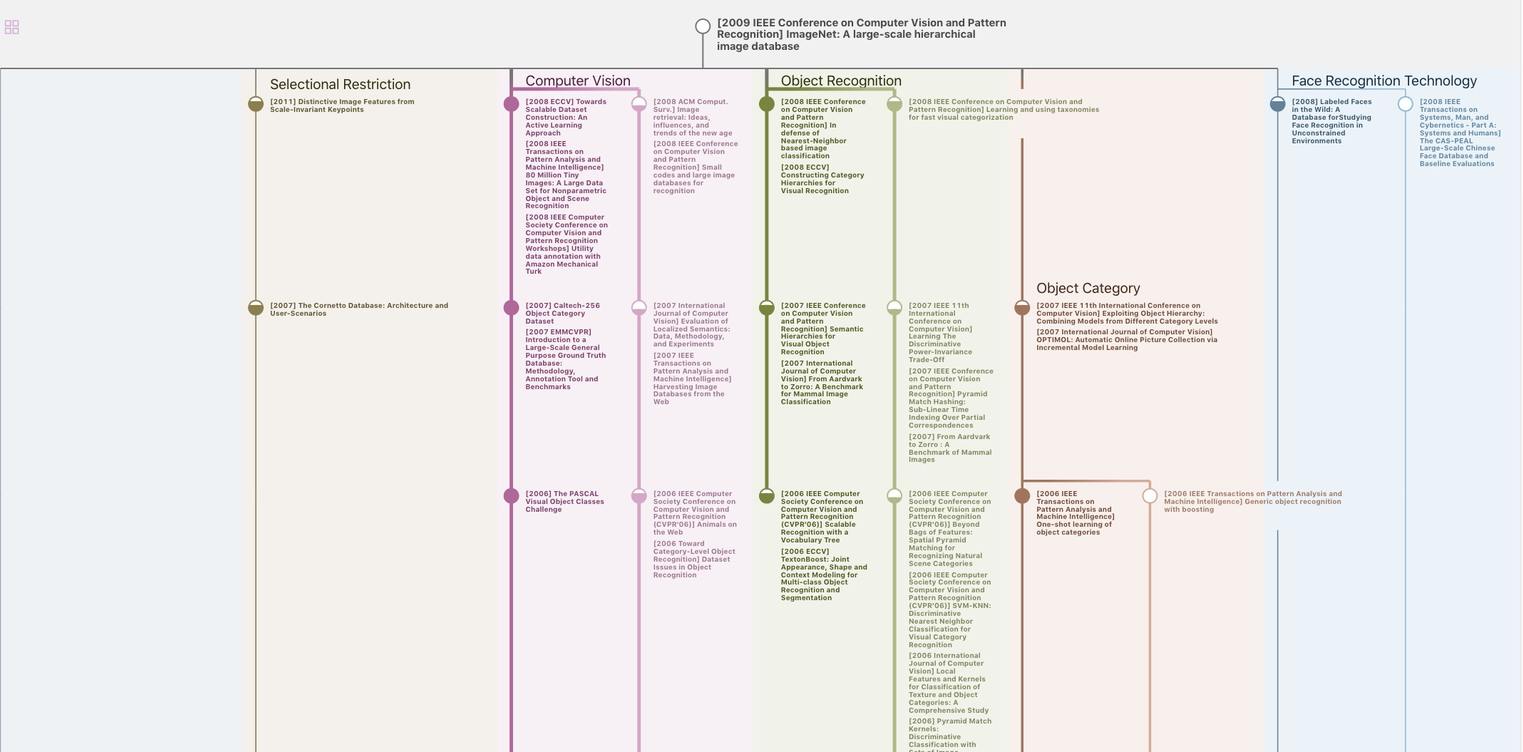
生成溯源树,研究论文发展脉络
Chat Paper
正在生成论文摘要