Machine Learning Emulation of Urban Land Surface Processes
JOURNAL OF ADVANCES IN MODELING EARTH SYSTEMS(2022)
摘要
Can we improve the modeling of urban land surface processes with machine learning (ML)? A prior comparison of urban land surface models (ULSMs) found that no single model is "best" at predicting all common surface fluxes. Here, we develop an urban neural network (UNN) trained on the mean predicted fluxes from 22 ULSMs at one site. The UNN emulates the mean output of ULSMs accurately. When compared to a reference ULSM (Town Energy Balance; TEB), the UNN has greater accuracy relative to flux observations, less computational cost, and requires fewer input parameters. When coupled to the Weather Research Forecasting (WRF) model using TensorFlow bindings, WRF-UNN is stable and more accurate than the reference WRF-TEB. Although the application is currently constrained by the training data (1 site), we show a novel approach to improve the modeling of surface fluxes by combining the strengths of several ULSMs into one using ML.
更多查看译文
关键词
machine learning, neural network, Weather Research Forecasting (WRF), numerical weather prediction (NWP), coupling, urban land surface
AI 理解论文
溯源树
样例
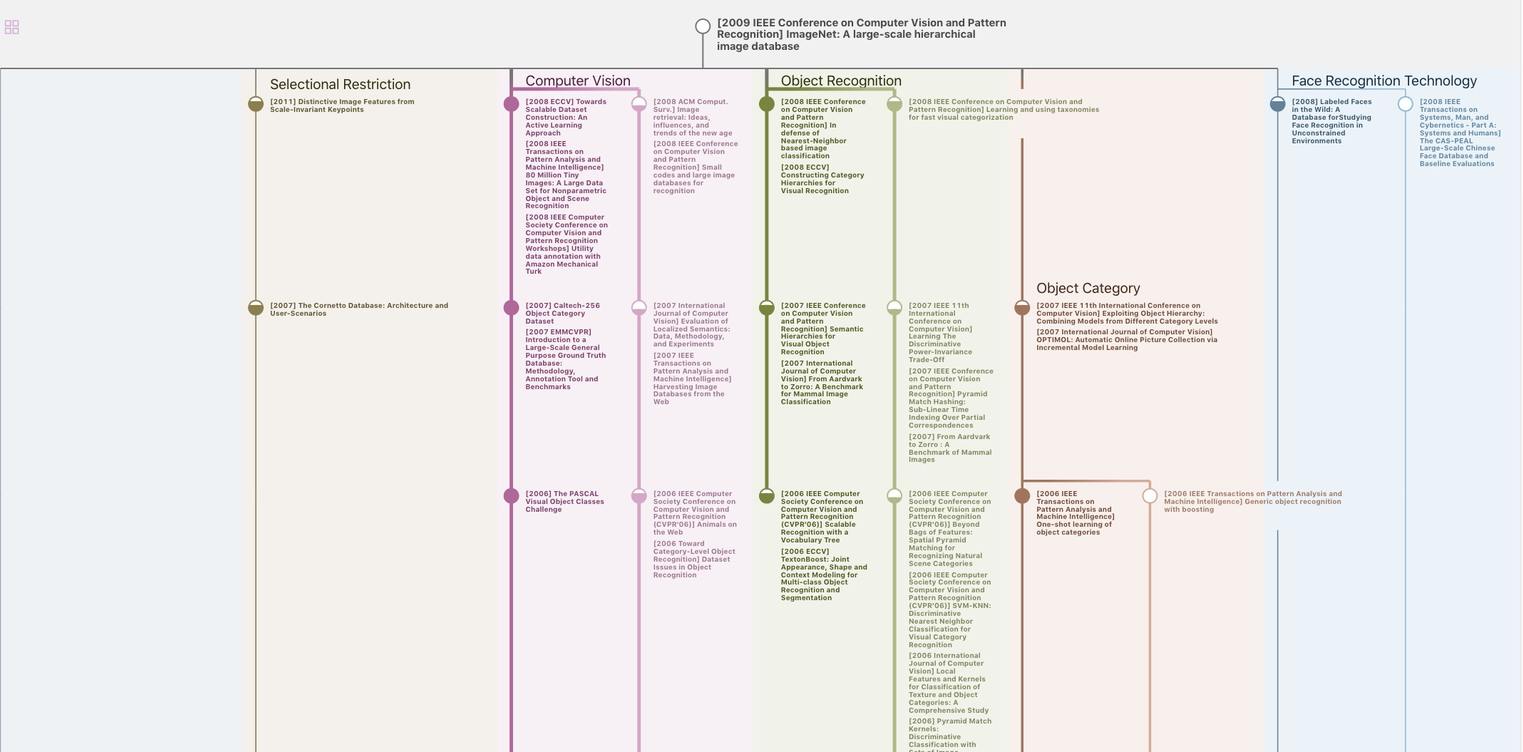
生成溯源树,研究论文发展脉络
Chat Paper
正在生成论文摘要