District Cooling System Control for Providing Operating Reserve Based on Safe Deep Reinforcement Learning
IEEE TRANSACTIONS ON POWER SYSTEMS(2024)
摘要
Heating, ventilation, and air conditioning (HVAC) systems are well proved to be capable to provide operating reserve for power systems. As a type of large-capacity and energy-efficient HVAC system (up to 100 MW), district cooling system (DCS) is emerging in modern cities and has huge potential to be regulated as a flexible load. However, strategically controlling a DCS to provide flexibility is challenging, because one DCS services multiple buildings with complex thermal dynamics and uncertain cooling demands. Improper control may lead to significant thermal discomfort and even deteriorate the power system's operation security. To address the above issues, we propose a model-free control strategy based on the deep reinforcement learning (DRL) without the requirement of accurate system model and uncertainty distribution. To avoid damaging "trial & error" actions that may violate the system's operation security during the training process, we further propose a safe layer combined to the DDPG to guarantee the satisfaction of critical constraints, forming a safe-DDPG scheme. Moreover, after providing operating reserve, DCS increases power and tries to recover all the buildings' temperature back to set values, which may cause an instantaneous peak-power rebound and bring a secondary negative impact on power systems. Therefore, we design a self-adaption reward function within the proposed safe-DDPG scheme to constrain the peak-power effectively. Numerical studies based on a realistic DCS demonstrate the effectiveness of the proposed methods.
更多查看译文
关键词
Cooling,Buildings,HVAC,Uncertainty,Power demand,Power system dynamics,Real-time systems,District cooling system,operating reserve,model-free control,safe deep reinforcement learning
AI 理解论文
溯源树
样例
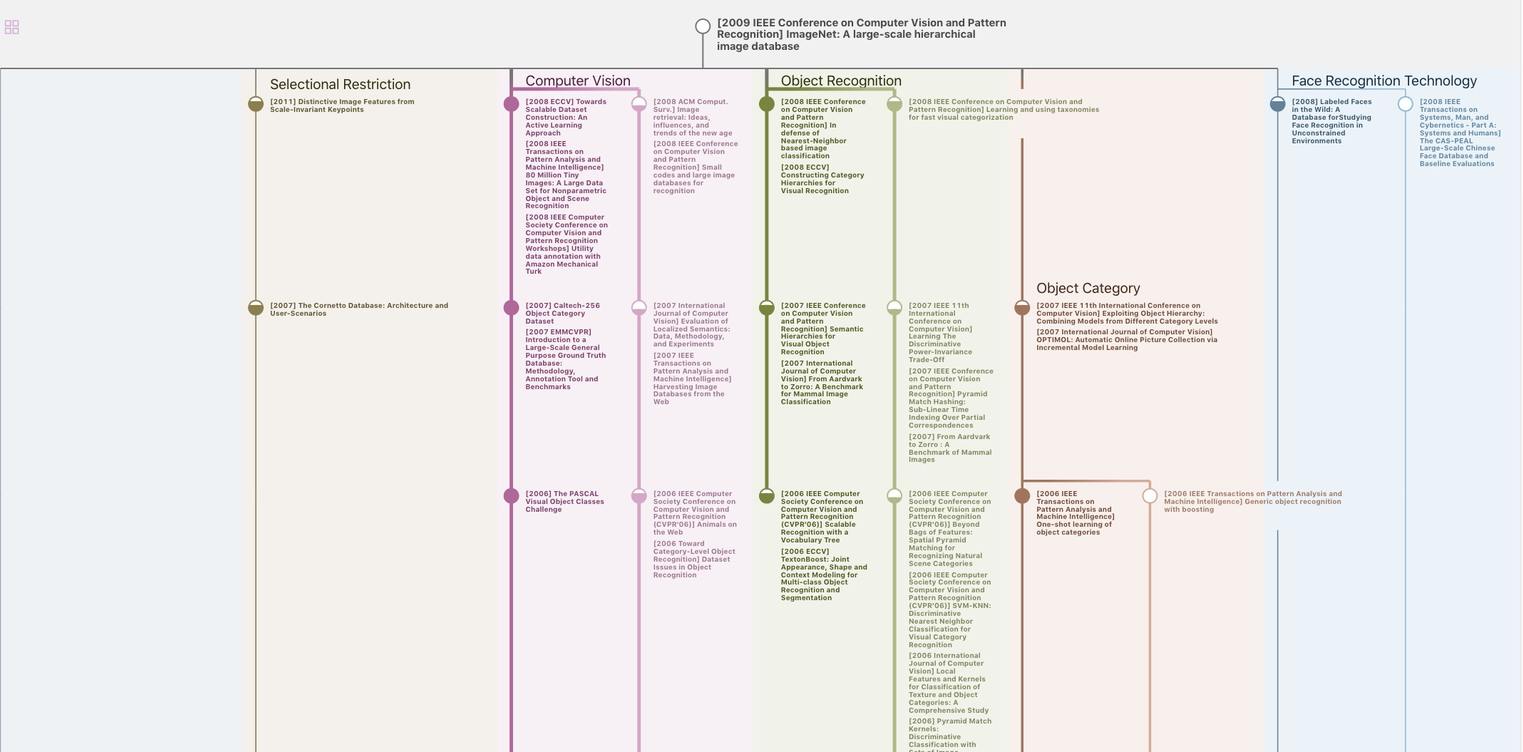
生成溯源树,研究论文发展脉络
Chat Paper
正在生成论文摘要