Efficient Large Scale Language Modeling with Mixtures of Experts.
emnlp 2022(2022)
摘要
Mixture of Experts layers (MoEs) enable efficient scaling of language models through conditional computation. This paper presents a detailed empirical study of how autoregressive MoE language models scale in comparison with dense models in a wide range of settings: in- and out-of-domain language modeling, zero- and few-shot priming, and full-shot fine-tuning. With the exception of fine-tuning, we find MoEs to be substantially more compute efficient. At more modest training budgets, MoEs can match the performance of dense models using $\sim$4 times less compute. This gap narrows at scale, but our largest MoE model (1.1T parameters) consistently outperforms a compute-equivalent dense model (6.7B parameters). Overall, this performance gap varies greatly across tasks and domains, suggesting that MoE and dense models generalize differently in ways that are worthy of future study. We make our code and models publicly available for research use.
更多查看译文
关键词
experts,language,mixtures
AI 理解论文
溯源树
样例
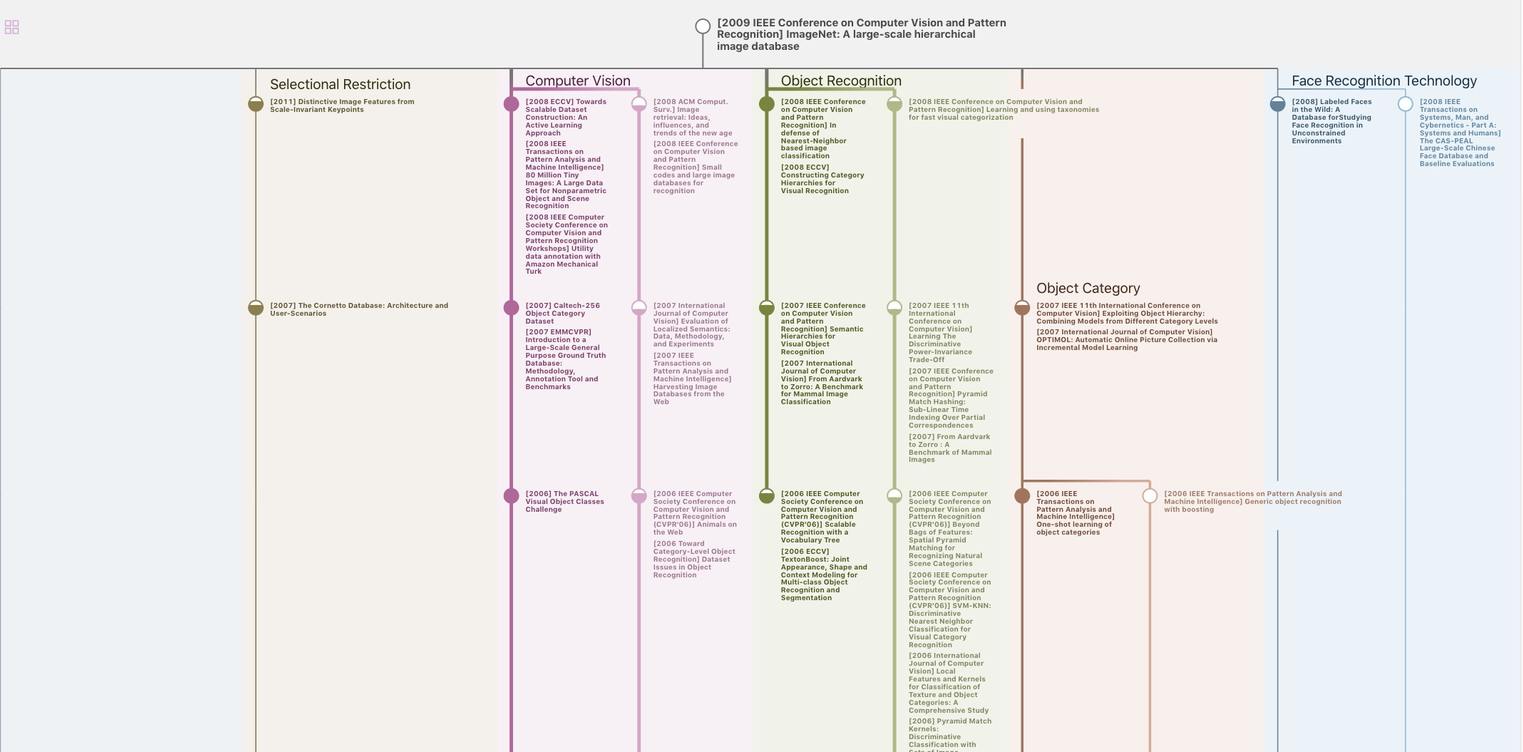
生成溯源树,研究论文发展脉络
Chat Paper
正在生成论文摘要