Hybrid Bayesian network discovery with latent variables by scoring multiple interventions
Data Min. Knowl. Discov.(2022)
摘要
In Bayesian Networks (BNs), the direction of edges is crucial for causal reasoning and inference. However, Markov equivalence class considerations mean it is not always possible to establish edge orientations, which is why many BN structure learning algorithms cannot orientate all edges from purely observational data. Moreover, latent confounders can lead to false positive edges. Relatively few methods have been proposed to address these issues. In this work, we present the hybrid mFGS-BS (majority rule and Fast Greedy equivalence Search with Bayesian Scoring) algorithm for structure learning from discrete data that involves an observational data set and one or more interventional data sets. The algorithm assumes causal insufficiency in the presence of latent variables and produces a Partial Ancestral Graph (PAG). Structure learning relies on a hybrid approach and a novel Bayesian scoring paradigm that calculates the posterior probability of each directed edge being added to the learnt graph. Experimental results based on well-known networks of up to 109 variables and 10 k sample size show that mFGS-BS improves structure learning accuracy relative to the state-of-the-art and it is computationally efficient.
更多查看译文
关键词
Ancestral graphs,Causal insufficiency,Latent confounders,Structure learning
AI 理解论文
溯源树
样例
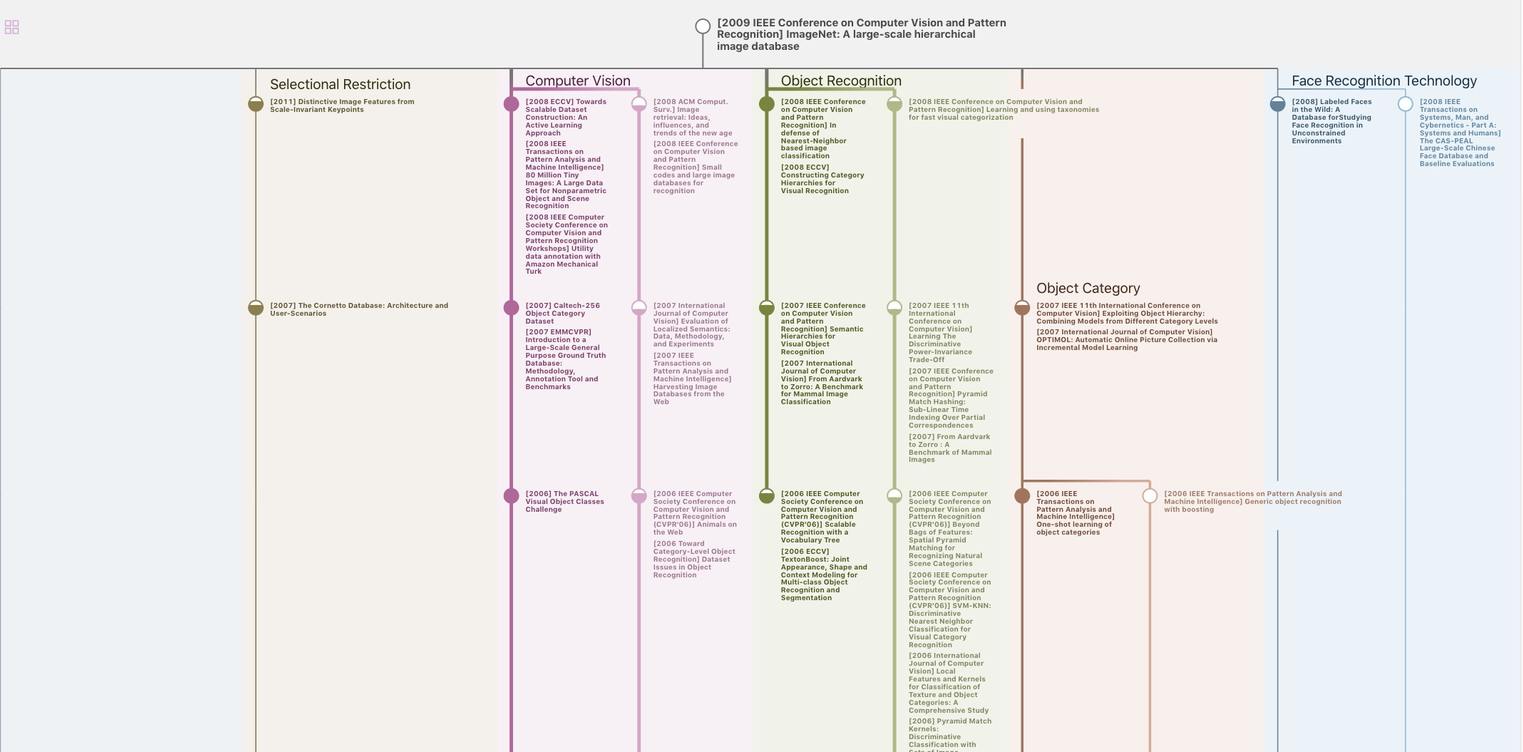
生成溯源树,研究论文发展脉络
Chat Paper
正在生成论文摘要