Classifier calibration: a survey on how to assess and improve predicted class probabilities
arxiv(2023)
摘要
This paper provides both an introduction to and a detailed overview of the principles and practice of classifier calibration. A well-calibrated classifier correctly quantifies the level of uncertainty or confidence associated with its instance-wise predictions. This is essential for critical applications, optimal decision making, cost-sensitive classification, and for some types of context change. Calibration research has a rich history which predates the birth of machine learning as an academic field by decades. However, a recent increase in the interest on calibration has led to new methods and the extension from binary to the multiclass setting. The space of options and issues to consider is large, and navigating it requires the right set of concepts and tools. We provide both introductory material and up-to-date technical details of the main concepts and methods, including proper scoring rules and other evaluation metrics, visualisation approaches, a comprehensive account of post-hoc calibration methods for binary and multiclass classification, and several advanced topics.
更多查看译文
关键词
classifier calibration
AI 理解论文
溯源树
样例
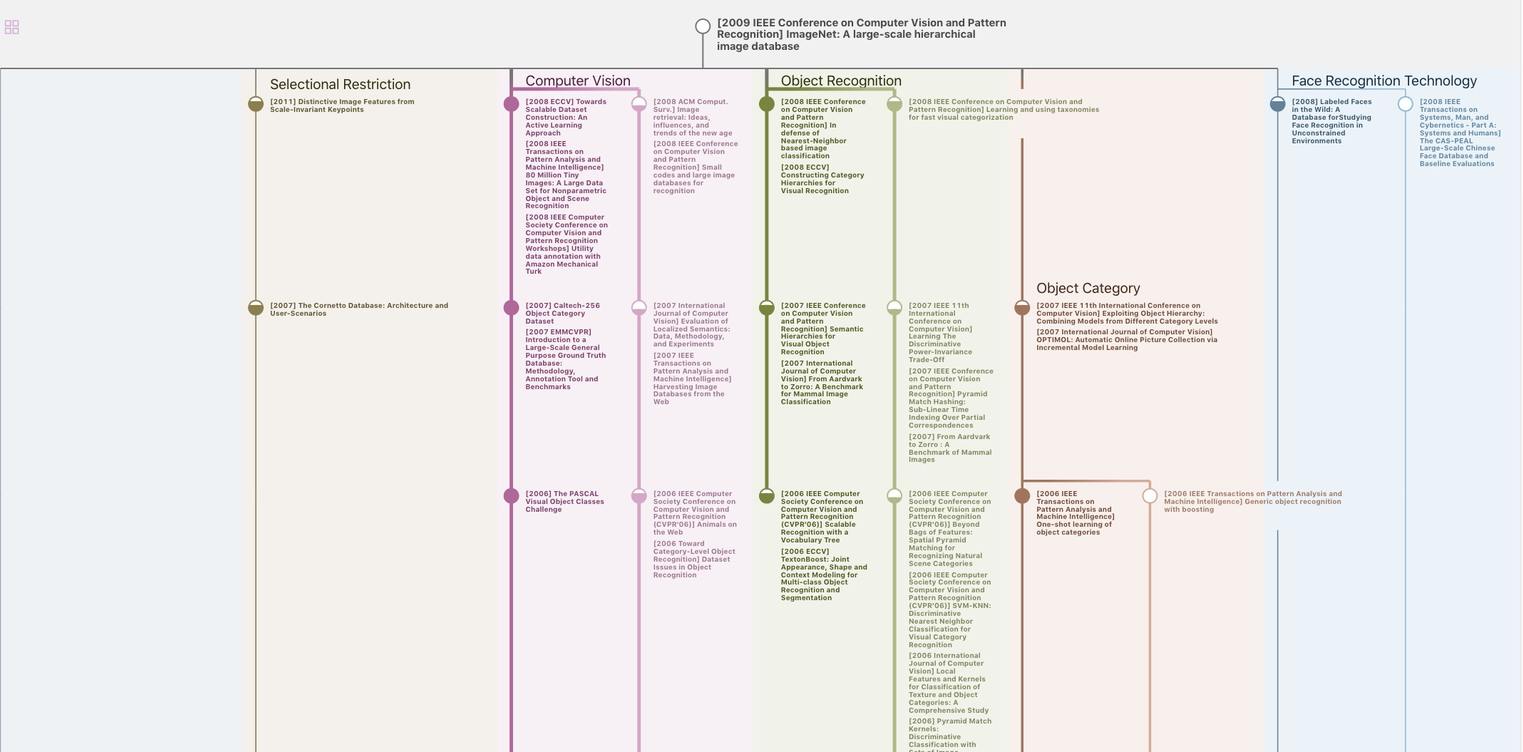
生成溯源树,研究论文发展脉络
Chat Paper
正在生成论文摘要