Wiener Guided DIP for Unsupervised Blind Image Deconvolution
WACV(2023)
摘要
Blind deconvolution is an ill-posed problem arising in various fields ranging from microscopy to astronomy. Its ill-posed nature demands adequate priors and initialization to arrive at a desirable solution. Recently, it has been shown that deep networks can serve as an image generation prior (DIP) during unsupervised blind deconvolution optimization, however, DIP's high frequency artifact suppression ability is not explicitly exploited. We propose to use Wiener-deconvolution to guide DIP during optimization in order to better leverage DIP's ability for blind image deconvolution. Wiener-deconvolution sharpens an image while introducing high-frequency artifacts, which are reproduced by DIP with a delay compared to low-frequency features and sharp edges, similar to what has been observed for noise. We embed the computational process in a constrained optimization problem together with an automatic kernel initialization method and show that the proposed method yields higher performance and stability across multiple datasets.
更多查看译文
关键词
Algorithms: Low-level and physics-based vision
AI 理解论文
溯源树
样例
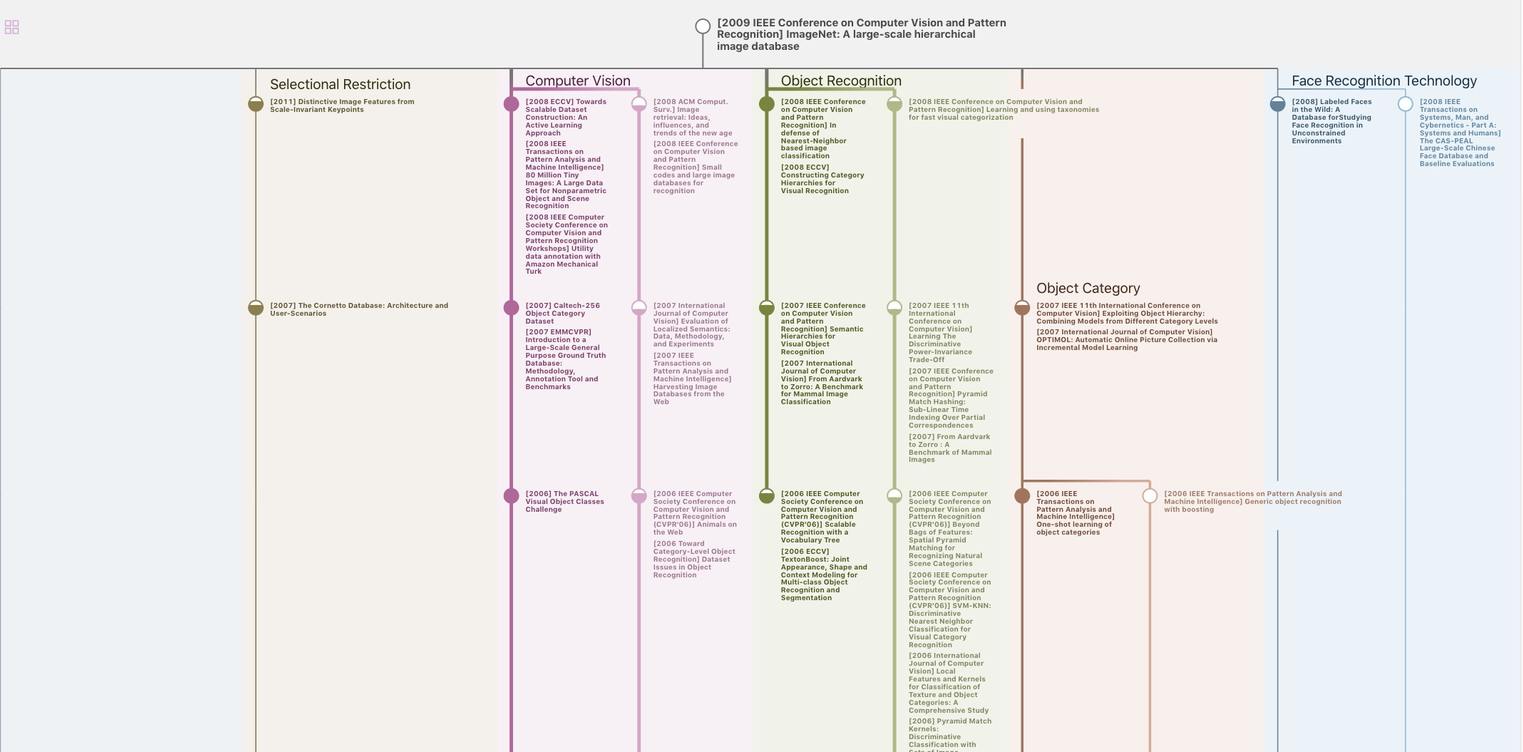
生成溯源树,研究论文发展脉络
Chat Paper
正在生成论文摘要